Added support for document loaders for Azure Blob Storage using a
connection string. Fixes#1805
---------
Co-authored-by: Mick Vleeshouwer <mick@imick.nl>
Ran into a broken build if bs4 wasn't installed in the project.
Minor tweak to follow the other doc loaders optional package-loading
conventions.
Also updated html docs to include reference to this new html loader.
side note: Should there be 2 different html-to-text document loaders?
This new one only handles local files, while the existing unstructured
html loader handles HTML from local and remote. So it seems like the
improvement was adding the title to the metadata, which is useful but
could also be added to `html.py`
In https://github.com/hwchase17/langchain/issues/1716 , it was
identified that there were two .py files performing similar tasks. As a
resolution, one of the files has been removed, as its purpose had
already been fulfilled by the other file. Additionally, the init has
been updated accordingly.
Furthermore, the how_to_guides.rst file has been updated to include
links to documentation that was previously missing. This was deemed
necessary as the existing list on
https://langchain.readthedocs.io/en/latest/modules/document_loaders/how_to_guides.html
was incomplete, causing confusion for users who rely on the full list of
documentation on the left sidebar of the website.
The GPT Index project is transitioning to the new project name,
LlamaIndex.
I've updated a few files referencing the old project name and repository
URL to the current ones.
From the [LlamaIndex repo](https://github.com/jerryjliu/llama_index):
> NOTE: We are rebranding GPT Index as LlamaIndex! We will carry out
this transition gradually.
>
> 2/25/2023: By default, our docs/notebooks/instructions now reference
"LlamaIndex" instead of "GPT Index".
>
> 2/19/2023: By default, our docs/notebooks/instructions now use the
llama-index package. However the gpt-index package still exists as a
duplicate!
>
> 2/16/2023: We have a duplicate llama-index pip package. Simply replace
all imports of gpt_index with llama_index if you choose to pip install
llama-index.
I'm not associated with LlamaIndex in any way. I just noticed the
discrepancy when studying the lanchain documentation.
# What does this PR do?
This PR adds similar to `llms` a SageMaker-powered `embeddings` class.
This is helpful if you want to leverage Hugging Face models on SageMaker
for creating your indexes.
I added a example into the
[docs/modules/indexes/examples/embeddings.ipynb](https://github.com/hwchase17/langchain/compare/master...philschmid:add-sm-embeddings?expand=1#diff-e82629e2894974ec87856aedd769d4bdfe400314b03734f32bee5990bc7e8062)
document. The example currently includes some `_### TEMPORARY: Showing
how to deploy a SageMaker Endpoint from a Hugging Face model ###_ ` code
showing how you can deploy a sentence-transformers to SageMaker and then
run the methods of the embeddings class.
@hwchase17 please let me know if/when i should remove the `_###
TEMPORARY: Showing how to deploy a SageMaker Endpoint from a Hugging
Face model ###_` in the description i linked to a detail blog on how to
deploy a Sentence Transformers so i think we don't need to include those
steps here.
I also reused the `ContentHandlerBase` from
`langchain.llms.sagemaker_endpoint` and changed the output type to `any`
since it is depending on the implementation.
Fixes the import typo in the vector db text generator notebook for the
chroma library
Co-authored-by: Anupam <anupam@10-16-252-145.dynapool.wireless.nyu.edu>
Use the following code to test:
```python
import os
from langchain.llms import OpenAI
from langchain.chains.api import podcast_docs
from langchain.chains import APIChain
# Get api key here: https://openai.com/pricing
os.environ["OPENAI_API_KEY"] = "sk-xxxxx"
# Get api key here: https://www.listennotes.com/api/pricing/
listen_api_key = 'xxx'
llm = OpenAI(temperature=0)
headers = {"X-ListenAPI-Key": listen_api_key}
chain = APIChain.from_llm_and_api_docs(llm, podcast_docs.PODCAST_DOCS, headers=headers, verbose=True)
chain.run("Search for 'silicon valley bank' podcast episodes, audio length is more than 30 minutes, return only 1 results")
```
Known issues: the api response data might be too big, and we'll get such
error:
`openai.error.InvalidRequestError: This model's maximum context length
is 4097 tokens, however you requested 6733 tokens (6477 in your prompt;
256 for the completion). Please reduce your prompt; or completion
length.`
New to Langchain, was a bit confused where I should find the toolkits
section when I'm at `agent/key_concepts` docs. I added a short link that
points to the how to section.
```
class Joke(BaseModel):
setup: str = Field(description="question to set up a joke")
punchline: str = Field(description="answer to resolve the joke")
joke_query = "Tell me a joke."
# Or, an example with compound type fields.
#class FloatArray(BaseModel):
# values: List[float] = Field(description="list of floats")
#
#float_array_query = "Write out a few terms of fiboacci."
model = OpenAI(model_name='text-davinci-003', temperature=0.0)
parser = PydanticOutputParser(pydantic_object=Joke)
prompt = PromptTemplate(
template="Answer the user query.\n{format_instructions}\n{query}\n",
input_variables=["query"],
partial_variables={"format_instructions": parser.get_format_instructions()}
)
_input = prompt.format_prompt(query=joke_query)
print("Prompt:\n", _input.to_string())
output = model(_input.to_string())
print("Completion:\n", output)
parsed_output = parser.parse(output)
print("Parsed completion:\n", parsed_output)
```
```
Prompt:
Answer the user query.
The output should be formatted as a JSON instance that conforms to the JSON schema below. For example, the object {"foo": ["bar", "baz"]} conforms to the schema {"foo": {"description": "a list of strings field", "type": "string"}}.
Here is the output schema:
---
{"setup": {"description": "question to set up a joke", "type": "string"}, "punchline": {"description": "answer to resolve the joke", "type": "string"}}
---
Tell me a joke.
Completion:
{"setup": "Why don't scientists trust atoms?", "punchline": "Because they make up everything!"}
Parsed completion:
setup="Why don't scientists trust atoms?" punchline='Because they make up everything!'
```
Ofc, works only with LMs of sufficient capacity. DaVinci is reliable but
not always.
---------
Co-authored-by: Harrison Chase <hw.chase.17@gmail.com>
PromptLayer now has support for [several different tracking
features.](https://magniv.notion.site/Track-4deee1b1f7a34c1680d085f82567dab9)
In order to use any of these features you need to have a request id
associated with the request.
In this PR we add a boolean argument called `return_pl_id` which will
add `pl_request_id` to the `generation_info` dictionary associated with
a generation.
We also updated the relevant documentation.
add the state_of_the_union.txt file so that its easier to follow through
with the example.
---------
Co-authored-by: Jithin James <jjmachan@pop-os.localdomain>
* Zapier Wrapper and Tools (implemented by Zapier Team)
* Zapier Toolkit, examples with mrkl agent
---------
Co-authored-by: Mike Knoop <mikeknoop@gmail.com>
Co-authored-by: Robert Lewis <robert.lewis@zapier.com>
### Summary
Allows users to pass in `**unstructured_kwargs` to Unstructured document
loaders. Implemented with the `strategy` kwargs in mind, but will pass
in other kwargs like `include_page_breaks` as well. The two currently
supported strategies are `"hi_res"`, which is more accurate but takes
longer, and `"fast"`, which processes faster but with lower accuracy.
The `"hi_res"` strategy is the default. For PDFs, if `detectron2` is not
available and the user selects `"hi_res"`, the loader will fallback to
using the `"fast"` strategy.
### Testing
#### Make sure the `strategy` kwarg works
Run the following in iPython to verify that the `"fast"` strategy is
indeed faster.
```python
from langchain.document_loaders import UnstructuredFileLoader
loader = UnstructuredFileLoader("layout-parser-paper-fast.pdf", strategy="fast", mode="elements")
%timeit loader.load()
loader = UnstructuredFileLoader("layout-parser-paper-fast.pdf", mode="elements")
%timeit loader.load()
```
On my system I get:
```python
In [3]: from langchain.document_loaders import UnstructuredFileLoader
In [4]: loader = UnstructuredFileLoader("layout-parser-paper-fast.pdf", strategy="fast", mode="elements")
In [5]: %timeit loader.load()
247 ms ± 369 µs per loop (mean ± std. dev. of 7 runs, 1 loop each)
In [6]: loader = UnstructuredFileLoader("layout-parser-paper-fast.pdf", mode="elements")
In [7]: %timeit loader.load()
2.45 s ± 31 ms per loop (mean ± std. dev. of 7 runs, 1 loop each)
```
#### Make sure older versions of `unstructured` still work
Run `pip install unstructured==0.5.3` and then verify the following runs
without error:
```python
from langchain.document_loaders import UnstructuredFileLoader
loader = UnstructuredFileLoader("layout-parser-paper-fast.pdf", mode="elements")
loader.load()
```
# Description
Add `RediSearch` vectorstore for LangChain
RediSearch: [RediSearch quick
start](https://redis.io/docs/stack/search/quick_start/)
# How to use
```
from langchain.vectorstores.redisearch import RediSearch
rds = RediSearch.from_documents(docs, embeddings,redisearch_url="redis://localhost:6379")
```
Seeing a lot of issues in Discord in which the LLM is not using the
correct LIMIT clause for different SQL dialects. ie, it's using `LIMIT`
for mssql instead of `TOP`, or instead of `ROWNUM` for Oracle, etc.
I think this could be due to us specifying the LIMIT statement in the
example rows portion of `table_info`. So the LLM is seeing the `LIMIT`
statement used in the prompt.
Since we can't specify each dialect's method here, I think it's fine to
just replace the `SELECT... LIMIT 3;` statement with `3 rows from
table_name table:`, and wrap everything in a block comment directly
following the `CREATE` statement. The Rajkumar et al paper wrapped the
example rows and `SELECT` statement in a block comment as well anyway.
Thoughts @fpingham?
`OnlinePDFLoader` and `PagedPDFSplitter` lived separate from the rest of
the pdf loaders.
Because they're all similar, I propose moving all to `pdy.py` and the
same docs/examples page.
Additionally, `PagedPDFSplitter` naming doesn't match the pattern the
rest of the loaders follow, so I renamed to `PyPDFLoader` and had it
inherit from `BasePDFLoader` so it can now load from remote file
sources.
Provide shared memory capability for the Agent.
Inspired by #1293 .
## Problem
If both Agent and Tools (i.e., LLMChain) use the same memory, both of
them will save the context. It can be annoying in some cases.
## Solution
Create a memory wrapper that ignores the save and clear, thereby
preventing updates from Agent or Tools.
Simple CSV document loader which wraps `csv` reader, and preps the file
with a single `Document` per row.
The column header is prepended to each value for context which is useful
for context with embedding and semantic search
This PR adds additional evaluation metrics for data-augmented QA,
resulting in a report like this at the end of the notebook:
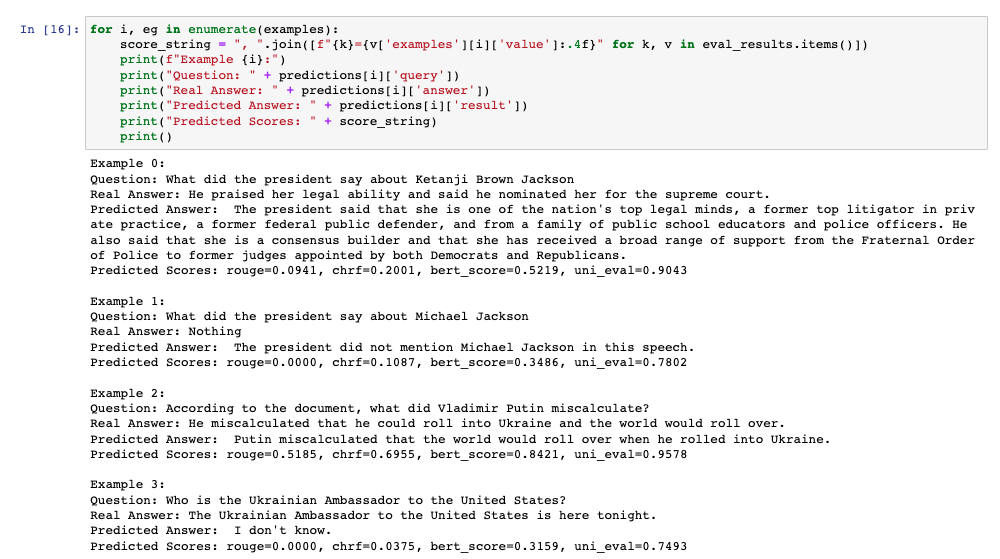
The score calculation is based on the
[Critique](https://docs.inspiredco.ai/critique/) toolkit, an API-based
toolkit (like OpenAI) that has minimal dependencies, so it should be
easy for people to run if they choose.
The code could further be simplified by actually adding a chain that
calls Critique directly, but that probably should be saved for another
PR if necessary. Any comments or change requests are welcome!
This pull request proposes an update to the Lightweight wrapper
library's documentation. The current documentation provides an example
of how to use the library's requests.run method, as follows:
requests.run("https://www.google.com"). However, this example does not
work for the 0.0.102 version of the library.
Testing:
The changes have been tested locally to ensure they are working as
intended.
Thank you for considering this pull request.
Different PDF libraries have different strengths and weaknesses. PyMuPDF
does a good job at extracting the most amount of content from the doc,
regardless of the source quality, extremely fast (especially compared to
Unstructured).
https://pymupdf.readthedocs.io/en/latest/index.html
- Added instructions on setting up self hosted searx
- Add notebook example with agent
- Use `localhost:8888` as example url to stay consistent since public
instances are not really usable.
Co-authored-by: blob42 <spike@w530>
The YAML and JSON examples of prompt serialization now give a strange
`No '_type' key found, defaulting to 'prompt'` message when you try to
run them yourself or copy the format of the files. The reason for this
harmless warning is that the _type key was not in the config files,
which means they are parsed as a standard prompt.
This could be confusing to new users (like it was confusing to me after
upgrading from 0.0.85 to 0.0.86+ for my few_shot prompts that needed a
_type added to the example_prompt config), so this update includes the
_type key just for clarity.
Obviously this is not critical as the warning is harmless, but it could
be confusing to track down or be interpreted as an error by a new user,
so this update should resolve that.
This PR:
- Increases `qdrant-client` version to 1.0.4
- Introduces custom content and metadata keys (as requested in #1087)
- Moves all the `QdrantClient` parameters into the method parameters to
simplify code completion
This PR adds
* `ZeroShotAgent.as_sql_agent`, which returns an agent for interacting
with a sql database. This builds off of `SQLDatabaseChain`. The main
advantages are 1) answering general questions about the db, 2) access to
a tool for double checking queries, and 3) recovering from errors
* `ZeroShotAgent.as_json_agent` which returns an agent for interacting
with json blobs.
* Several examples in notebooks
---------
Co-authored-by: Harrison Chase <hw.chase.17@gmail.com>
Currently, table information is gathered through SQLAlchemy as complete
table DDL and a user-selected number of sample rows from each table.
This PR adds the option to use user-defined table information instead of
automatically collecting it. This will use the provided table
information and fall back to the automatic gathering for tables that the
user didn't provide information for.
Off the top of my head, there are a few cases where this can be quite
useful:
- The first n rows of a table are uninformative, or very similar to one
another. In this case, hand-crafting example rows for a table such that
they provide the good, diverse information can be very helpful. Another
approach we can think about later is getting a random sample of n rows
instead of the first n rows, but there are some performance
considerations that need to be taken there. Even so, hand-crafting the
sample rows is useful and can guarantee the model sees informative data.
- The user doesn't want every column to be available to the model. This
is not an elegant way to fulfill this specific need since the user would
have to provide the table definition instead of a simple list of columns
to include or ignore, but it does work for this purpose.
- For the developers, this makes it a lot easier to compare/benchmark
the performance of different prompting structures for providing table
information in the prompt.
These are cases I've run into myself (particularly cases 1 and 3) and
I've found these changes useful. Personally, I keep custom table info
for a few tables in a yaml file for versioning and easy loading.
Definitely open to other opinions/approaches though!
iFixit is a wikipedia-like site that has a huge amount of open content
on how to fix things, questions/answers for common troubleshooting and
"things" related content that is more technical in nature. All content
is licensed under CC-BY-SA-NC 3.0
Adding docs from iFixit as context for user questions like "I dropped my
phone in water, what do I do?" or "My macbook pro is making a whining
noise, what's wrong with it?" can yield significantly better responses
than context free response from LLMs.
### Summary
Adds a document loader for image files such as `.jpg` and `.png` files.
### Testing
Run the following using the example document from the [`unstructured`
repo](https://github.com/Unstructured-IO/unstructured/tree/main/example-docs).
```python
from langchain.document_loaders.image import UnstructuredImageLoader
loader = UnstructuredImageLoader("layout-parser-paper-fast.jpg")
loader.load()
```
nitpicking but just thought i'd add this typo which I found when going
through the How-to 😄 (unless it was intentional) also, it's amazing that
you added ReAct to LangChain!
Checking if weaviate similarity_search kwargs contains "certainty" and
use it accordingly. The minimal level of certainty must be a float, and
it is computed by normalized distance.
Thanks for all your hard work!
I noticed a small typo in the bash util doc so here's a quick update.
Additionally, my formatter caught some spacing in the `.md` as well.
Happy to revert that if it's an issue.
The main change is just
```
- A common use case this is for letting it interact with your local file system.
+ A common use case for this is letting the LLM interact with your local file system.
```
## Testing
`make docs_build` succeeds locally and the changes show as expected ✌️
<img width="704" alt="image"
src="https://user-images.githubusercontent.com/17773666/221376160-e99e59a6-b318-49d1-a1d7-89f5c17cdab4.png">
I've added a simple
[CoNLL-U](https://universaldependencies.org/format.html) document
loader. CoNLL-U is a common format for NLP tasks and is used, for
example, in the Universal Dependencies treebank corpora. The loader
reads a single file in standard CoNLL-U format and returns a document.
### Summary
Adds a document loader for MS Word Documents. Works with both `.docx`
and `.doc` files as longer as the user has installed
`unstructured>=0.4.11`.
### Testing
The follow workflow test the loader for both `.doc` and `.docx` files
using example docs from the `unstructured` repo.
#### `.docx`
```python
from langchain.document_loaders import UnstructuredWordDocumentLoader
filename = "../unstructured/example-docs/fake.docx"
loader = UnstructuredWordDocumentLoader(filename)
loader.load()
```
#### `.doc`
```python
from langchain.document_loaders import UnstructuredWordDocumentLoader
filename = "../unstructured/example-docs/fake.doc"
loader = UnstructuredWordDocumentLoader(filename)
loader.load()
```
`NotebookLoader.load()` loads the `.ipynb` notebook file into a
`Document` object.
**Parameters**:
* `include_outputs` (bool): whether to include cell outputs in the
resulting document (default is False).
* `max_output_length` (int): the maximum number of characters to include
from each cell output (default is 10).
* `remove_newline` (bool): whether to remove newline characters from the
cell sources and outputs (default is False).
* `traceback` (bool): whether to include full traceback (default is
False).
### Summary
Updates the docs to remove the `nltk` download steps from
`unstructured`. As of `unstructured` `0.4.14`, this is handled
automatically in the relevant modules within `unstructured`.
Link for easier navigation (it's not immediately clear where to find
more info on SimpleSequentialChain (3 clicks away)
---------
Co-authored-by: Larry Fisherman <l4rryfisherman@protonmail.com>
Added a GitBook document loader. It lets you both, (1) fetch text from
any single GitBook page, or (2) fetch all relative paths and return
their respective content in Documents.
I've modified the `scrape` method in the `WebBaseLoader` to accept
custom web paths if given, but happy to remove it and move that logic
into the `GitbookLoader` itself.
### Description
This PR adds a wrapper which adds support for the OpenSearch vector
database. Using opensearch-py client we are ingesting the embeddings of
given text into opensearch cluster using Bulk API. We can perform the
`similarity_search` on the index using the 3 popular searching methods
of OpenSearch k-NN plugin:
- `Approximate k-NN Search` use approximate nearest neighbor (ANN)
algorithms from the [nmslib](https://github.com/nmslib/nmslib),
[faiss](https://github.com/facebookresearch/faiss), and
[Lucene](https://lucene.apache.org/) libraries to power k-NN search.
- `Script Scoring` extends OpenSearch’s script scoring functionality to
execute a brute force, exact k-NN search.
- `Painless Scripting` adds the distance functions as painless
extensions that can be used in more complex combinations. Also, supports
brute force, exact k-NN search like Script Scoring.
### Issues Resolved
https://github.com/hwchase17/langchain/issues/1054
---------
Signed-off-by: Naveen Tatikonda <navtat@amazon.com>
Follow-up of @hinthornw's PR:
- Migrate the Tool abstraction to a separate file (`BaseTool`).
- `Tool` implementation of `BaseTool` takes in function and coroutine to
more easily maintain backwards compatibility
- Add a Toolkit abstraction that can own the generation of tools around
a shared concept or state
---------
Co-authored-by: William FH <13333726+hinthornw@users.noreply.github.com>
Co-authored-by: Harrison Chase <hw.chase.17@gmail.com>
Co-authored-by: Francisco Ingham <fpingham@gmail.com>
Co-authored-by: Dhruv Anand <105786647+dhruv-anand-aintech@users.noreply.github.com>
Co-authored-by: cragwolfe <cragcw@gmail.com>
Co-authored-by: Anton Troynikov <atroyn@users.noreply.github.com>
Co-authored-by: Oliver Klingefjord <oliver@klingefjord.com>
Co-authored-by: William Fu-Hinthorn <whinthorn@Williams-MBP-3.attlocal.net>
Co-authored-by: Bruno Bornsztein <bruno.bornsztein@gmail.com>
This is a work in progress PR to track my progres.
## TODO:
- [x] Get results using the specifed searx host
- [x] Prioritize returning an `answer` or results otherwise
- [ ] expose the field `infobox` when available
- [ ] expose `score` of result to help agent's decision
- [ ] expose the `suggestions` field to agents so they could try new
queries if no results are found with the orignial query ?
- [ ] Dynamic tool description for agents ?
- Searx offers many engines and a search syntax that agents can take
advantage of. It would be nice to generate a dynamic Tool description so
that it can be used many times as a tool but for different purposes.
- [x] Limit number of results
- [ ] Implement paging
- [x] Miror the usage of the Google Search tool
- [x] easy selection of search engines
- [x] Documentation
- [ ] update HowTo guide notebook on Search Tools
- [ ] Handle async
- [ ] Tests
### Add examples / documentation on possible uses with
- [ ] getting factual answers with `!wiki` option and `infoboxes`
- [ ] getting `suggestions`
- [ ] getting `corrections`
---------
Co-authored-by: blob42 <spike@w530>
Co-authored-by: Harrison Chase <hw.chase.17@gmail.com>
Alternate implementation to PR #960 Again - only FAISS is implemented.
If accepted can add this to other vectorstores or leave as
NotImplemented? Suggestions welcome...
This PR updates `PromptLayerOpenAI` to now support requests using the
[Async
API](https://langchain.readthedocs.io/en/latest/modules/llms/async_llm.html)
It also updates the documentation on Async API to let users know that
PromptLayerOpenAI also supports this.
`PromptLayerOpenAI` now redefines `_agenerate` a similar was to how it
redefines `_generate`
Adds Google Search integration with [Serper](https://serper.dev) a
low-cost alternative to SerpAPI (10x cheaper + generous free tier).
Includes documentation, tests and examples. Hopefully I am not missing
anything.
Developers can sign up for a free account at
[serper.dev](https://serper.dev) and obtain an api key.
## Usage
```python
from langchain.utilities import GoogleSerperAPIWrapper
from langchain.llms.openai import OpenAI
from langchain.agents import initialize_agent, Tool
import os
os.environ["SERPER_API_KEY"] = ""
os.environ['OPENAI_API_KEY'] = ""
llm = OpenAI(temperature=0)
search = GoogleSerperAPIWrapper()
tools = [
Tool(
name="Intermediate Answer",
func=search.run
)
]
self_ask_with_search = initialize_agent(tools, llm, agent="self-ask-with-search", verbose=True)
self_ask_with_search.run("What is the hometown of the reigning men's U.S. Open champion?")
```
### Output
```
Entering new AgentExecutor chain...
Yes.
Follow up: Who is the reigning men's U.S. Open champion?
Intermediate answer: Current champions Carlos Alcaraz, 2022 men's singles champion.
Follow up: Where is Carlos Alcaraz from?
Intermediate answer: El Palmar, Spain
So the final answer is: El Palmar, Spain
> Finished chain.
'El Palmar, Spain'
```
This PR updates the usage instructions for PromptLayerOpenAI in
Langchain's documentation. The updated instructions provide more detail
and conform better to the style of other LLM integration documentation
pages.
No code changes were made in this PR, only improvements to the
documentation. This update will make it easier for users to understand
how to use `PromptLayerOpenAI`
Currently the chain is getting the column names and types on the one
side and the example rows on the other. It is easier for the llm to read
the table information if the column name and examples are shown together
so that it can easily understand to which columns do the examples refer
to. For an instantiation of this, please refer to the changes in the
`sqlite.ipynb` notebook.
Also changed `eval` for `ast.literal_eval` when interpreting the results
from the sample row query since it is a better practice.
---------
Co-authored-by: Francisco Ingham <>
---------
Co-authored-by: Francisco Ingham <fpingham@gmail.com>
The provided example uses the default `max_length` of `20` tokens, which
leads to the example generation getting cut off. 20 tokens is way too
short to show CoT reasoning, so I boosted it to `64`.
Without knowing HF's API well, it can be hard to figure out just where
those `model_kwargs` come from, and `max_length` is a super critical
one.
Co-authored-by: Andrew White <white.d.andrew@gmail.com>
Co-authored-by: Harrison Chase <harrisonchase@Harrisons-MBP.attlocal.net>
Co-authored-by: Peng Qu <82029664+pengqu123@users.noreply.github.com>
Supporting asyncio in langchain primitives allows for users to run them
concurrently and creates more seamless integration with
asyncio-supported frameworks (FastAPI, etc.)
Summary of changes:
**LLM**
* Add `agenerate` and `_agenerate`
* Implement in OpenAI by leveraging `client.Completions.acreate`
**Chain**
* Add `arun`, `acall`, `_acall`
* Implement them in `LLMChain` and `LLMMathChain` for now
**Agent**
* Refactor and leverage async chain and llm methods
* Add ability for `Tools` to contain async coroutine
* Implement async SerpaPI `arun`
Create demo notebook.
Open questions:
* Should all the async stuff go in separate classes? I've seen both
patterns (keeping the same class and having async and sync methods vs.
having class separation)
PR to fix outdated environment details in the docs, see issue #897
I added code comments as pointers to where users go to get API keys, and
where they can find the relevant environment variable.
Signed-off-by: Filip Haltmayer <filip.haltmayer@zilliz.com>
Signed-off-by: Frank Liu <frank.liu@zilliz.com>
Co-authored-by: Filip Haltmayer <81822489+filip-halt@users.noreply.github.com>
Co-authored-by: Frank Liu <frank@frankzliu.com>
It's generally considered to be a good practice to pin dependencies to
prevent surprise breakages when a new version of a dependency is
released. This commit adds the ability to pin dependencies when loading
from LangChainHub.
Centralizing this logic and using urllib fixes an issue identified by
some windows users highlighted in this video -
https://youtu.be/aJ6IQUh8MLQ?t=537
The agents usually benefit from understanding what the data looks like
to be able to filter effectively. Sending just one row in the table info
allows the agent to understand the data before querying and get better
results.
---------
Co-authored-by: Francisco Ingham <>
---------
Co-authored-by: Francisco Ingham <fpingham@gmail.com>
On the [Getting Started
page](https://langchain.readthedocs.io/en/latest/modules/prompts/getting_started.html)
for prompt templates, I believe the very last example
```python
print(dynamic_prompt.format(adjective=long_string))
```
should actually be
```python
print(dynamic_prompt.format(input=long_string))
```
The existing example produces `KeyError: 'input'` as expected
***
On the [Create a custom prompt
template](https://langchain.readthedocs.io/en/latest/modules/prompts/examples/custom_prompt_template.html#id1)
page, I believe the line
```python
Function Name: {kwargs["function_name"]}
```
should actually be
```python
Function Name: {kwargs["function_name"].__name__}
```
The existing example produces the prompt:
```
Given the function name and source code, generate an English language explanation of the function.
Function Name: <function get_source_code at 0x7f907bc0e0e0>
Source Code:
def get_source_code(function_name):
# Get the source code of the function
return inspect.getsource(function_name)
Explanation:
```
***
On the [Example
Selectors](https://langchain.readthedocs.io/en/latest/modules/prompts/examples/example_selectors.html)
page, the first example does not define `example_prompt`, which is also
subtly different from previous example prompts used. For user
convenience, I suggest including
```python
example_prompt = PromptTemplate(
input_variables=["input", "output"],
template="Input: {input}\nOutput: {output}",
)
```
in the code to be copy-pasted
tl;dr: input -> word, output -> antonym, rename to dynamic_prompt
consistently
The provided code in this example doesn't run, because the keys are
`word` and `antonym`, rather than `input` and `output`.
Also, the `ExampleSelector`-based prompt is named `few_shot_prompt` when
defined and `dynamic_prompt` in the follow-up example. The former name
is less descriptive and collides with an earlier example, so I opted for
the latter.
Thanks for making a really cool library!
For using Azure OpenAI API, we need to set multiple env vars. But as can
be seen in openai package
[here](48b69293a3/openai/__init__.py (L35)),
the env var for setting base url is named `OPENAI_API_BASE` and not
`OPENAI_API_BASE_URL`. This PR fixes that part in the documentation.
I originally had only modified the `from_llm` to include the prompt but
I realized that if the prompt keys used on the custom prompt didn't
match the default prompt, it wouldn't work because of how `apply` works.
So I made some changes to the evaluate method to check if the prompt is
the default and if not, it will check if the input keys are the same as
the prompt key and update the inputs appropriately.
Let me know if there is a better way to do this.
Also added the custom prompt to the QA eval notebook.
add a chain that applies a prompt to all inputs and then returns not
only an answer but scores it
add examples for question answering and question answering with sources
Small quick fix:
Suggest making the order of the menu the same as it is written on the
page (Getting Started -> Key Concepts). Before the menu order was not
the same as it was on the page. Not sure if this is the only place the
menu is affected.
Mismatch is found here:
https://langchain.readthedocs.io/en/latest/modules/llms.html
- Add support for local build and linkchecking of docs
- Add GitHub Action to automatically check links before prior to
publication
- Minor reformat of Contributing readme
- Fix existing broken links
Co-authored-by: Hunter Gerlach <hunter@huntergerlach.com>
Co-authored-by: Hunter Gerlach <HunterGerlach@users.noreply.github.com>
Co-authored-by: Hunter Gerlach <hunter@huntergerlach.com>
I noticed (after publication) that the getting_started link on the main
page was borked. This should fix it.
Co-authored-by: Hunter Gerlach <hunter@huntergerlach.com>
Big docs refactor! Motivation is to make it easier for people to find
resources they are looking for. To accomplish this, there are now three
main sections:
- Getting Started: steps for getting started, walking through most core
functionality
- Modules: these are different modules of functionality that langchain
provides. Each part here has a "getting started", "how to", "key
concepts" and "reference" section (except in a few select cases where it
didnt easily fit).
- Use Cases: this is to separate use cases (like summarization, question
answering, evaluation, etc) from the modules, and provide a different
entry point to the code base.
There is also a full reference section, as well as extra resources
(glossary, gallery, etc)
Co-authored-by: Shreya Rajpal <ShreyaR@users.noreply.github.com>
I was honored by the twitter mention, so used PyCharm to try and... help
docs even a little bit.
Mostly typo-s and correct spellings.
PyCharm really complains about "really good" being used all the time and
recommended alternative wordings haha
Hi! This PR adds support for the Azure OpenAI service to LangChain.
I've tried to follow the contributing guidelines.
Co-authored-by: Keiji Kanazawa <{ID}+{username}@users.noreply.github.com>
Created a generic SQLAlchemyCache class to plug any database supported
by SQAlchemy. (I am using Postgres).
I also based the class SQLiteCache class on this class SQLAlchemyCache.
As a side note, I'm questioning the need for two distinct class
LLMCache, FullLLMCache. Shouldn't we merge both ?
Nothing of substance was changed. I simply corrected a few minor errors
that could slow down the reader.
Co-authored-by: Hunter Gerlach <hunter@huntergerlach.com>
Love the project, a ton of fun!
I think the PR is pretty self-explanatory, happy to make any changes! I
am working on using it in an `LLMBashChain` and may update as that
progresses.
Co-authored-by: Harrison Chase <hw.chase.17@gmail.com>
With the original prompt, the chain keeps trying to jump straight to
doing math directly, without first looking up ages. With this two-part
question, it behaves more as intended:
> Entering new ZeroShotAgent chain...
How old is Olivia Wilde's boyfriend? What is that number raised to the
0.23 power?
Thought: I need to find out how old Olivia Wilde's boyfriend is, and
then use a calculator to calculate the power.
Action: Search
Action Input: Olivia Wilde's boyfriend age
Observation: While Wilde, 37, and Styles, 27, have both kept a low
profile when it comes to talking about their relationship, Wilde did
address their ...
Thought: Olivia Wilde's boyfriend is 27 years old.
Action: Calculator
Action Input: 27^0.23
> Entering new LLMMathChain chain...
27^0.23
```python
import math
print(math.pow(27, 0.23))
```
Answer: 2.1340945944237553
> Finished LLMMathChain chain.
Observation: Answer: 2.1340945944237553
Thought: I now know the final answer.
Final Answer: 2.1340945944237553
> Finished ZeroShotAgent chain.
Add MemoryChain and ConversationChain as chains that take a docstore in
addition to the prompt, and use the docstore to stuff context into the
prompt. This can be used to have an ongoing conversation with a chatbot.
Probably needs a bit of refactoring for code quality
Co-authored-by: Harrison Chase <hw.chase.17@gmail.com>
Also updated docs, and noticed an issue with the add_texts method on
VectorStores that I had missed before -- the metadatas arg should be
required to match the classmethod which initializes the VectorStores
(the add_example methods break otherwise in the ExampleSelectors)
Without the print on the `llm` call, the new user sees no visible effect
when just getting started. The assumption here is the new user is
running this in a new sandbox script file or repl via copy-paste.