# Improve Cypher QA prompt
The current QA prompt is optimized for networkX answer generation, which
returns all the possible triples.
However, Cypher search is a bit more focused and doesn't necessary
return all the context information.
Due to that reason, the model sometimes refuses to generate an answer
even though the information is provided:
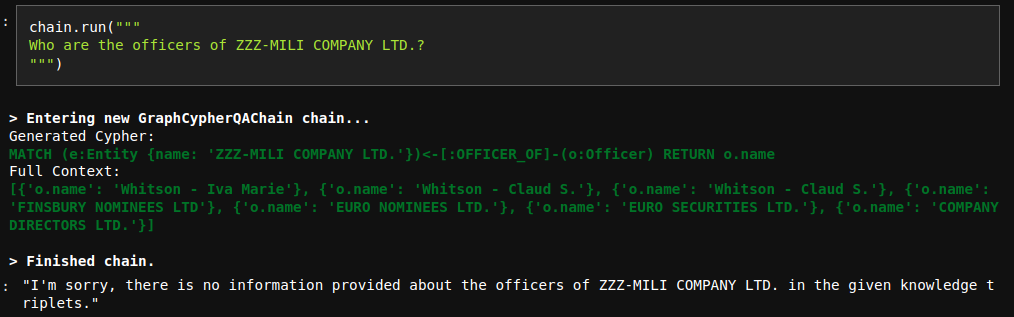
To fix this issue, I have updated the prompt. Interestingly, I tried
many variations with less instructions and they didn't work properly.
However, the current fix works nicely.
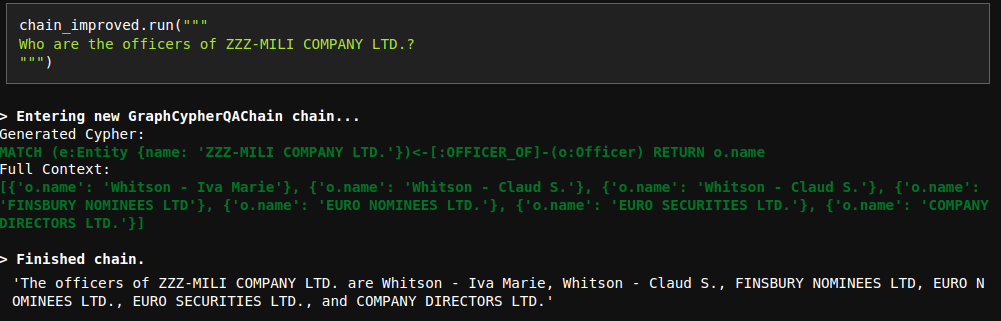
# Reuse `length_func` in `MapReduceDocumentsChain`
Pretty straightforward refactor in `MapReduceDocumentsChain`. Reusing
the local variable `length_func`, instead of the longer alternative
`self.combine_document_chain.prompt_length`.
@hwchase17
Follow up of https://github.com/hwchase17/langchain/pull/5015
Thanks for catching this!
Just a small PR to adjust couple of strings to these changes
Signed-off-by: jupyterjazz <saba.sturua@jina.ai>
# Beam
Calls the Beam API wrapper to deploy and make subsequent calls to an
instance of the gpt2 LLM in a cloud deployment. Requires installation of
the Beam library and registration of Beam Client ID and Client Secret.
Additional calls can then be made through the instance of the large
language model in your code or by calling the Beam API.
---------
Co-authored-by: Dev 2049 <dev.dev2049@gmail.com>
# Vectara Integration
This PR provides integration with Vectara. Implemented here are:
* langchain/vectorstore/vectara.py
* tests/integration_tests/vectorstores/test_vectara.py
* langchain/retrievers/vectara_retriever.py
And two IPYNB notebooks to do more testing:
* docs/modules/chains/index_examples/vectara_text_generation.ipynb
* docs/modules/indexes/vectorstores/examples/vectara.ipynb
---------
Co-authored-by: Dev 2049 <dev.dev2049@gmail.com>
# DOCS added missed document_loader examples
Added missed examples: `JSON`, `Open Document Format (ODT)`,
`Wikipedia`, `tomarkdown`.
Updated them to a consistent format.
## Who can review?
@hwchase17
@dev2049
# Clarification of the reference to the "get_text_legth" function in
getting_started.md
Reference to the function "get_text_legth" in the documentation did not
make sense. Comment added for clarification.
@hwchase17
# Docs: updated getting_started.md
Just accommodating some unnecessary spaces in the example of "pass few
shot examples to a prompt template".
@vowelparrot
# Same as PR #5045, but for async
<!--
Thank you for contributing to LangChain! Your PR will appear in our next
release under the title you set. Please make sure it highlights your
valuable contribution.
Replace this with a description of the change, the issue it fixes (if
applicable), and relevant context. List any dependencies required for
this change.
After you're done, someone will review your PR. They may suggest
improvements. If no one reviews your PR within a few days, feel free to
@-mention the same people again, as notifications can get lost.
-->
<!-- Remove if not applicable -->
Fixes#4825
I had forgotten to update the asynchronous counterpart `aadd_documents`
with the bug fix from PR #5045, so this PR also fixes `aadd_documents`
too.
## Who can review?
Community members can review the PR once tests pass. Tag
maintainers/contributors who might be interested:
@dev2049
<!-- For a quicker response, figure out the right person to tag with @
@hwchase17 - project lead
Tracing / Callbacks
- @agola11
Async
- @agola11
DataLoaders
- @eyurtsev
Models
- @hwchase17
- @agola11
Agents / Tools / Toolkits
- @vowelparrot
VectorStores / Retrievers / Memory
- @dev2049
-->
# Add async versions of predict() and predict_messages()
#4615 introduced a unifying interface for "base" and "chat" LLM models
via the new `predict()` and `predict_messages()` methods that allow both
types of models to operate on string and message-based inputs,
respectively.
This PR adds async versions of the same (`apredict()` and
`apredict_messages()`) that are identical except for their use of
`agenerate()` in place of `generate()`, which means they repurpose all
existing work on the async backend.
## Who can review?
Community members can review the PR once tests pass. Tag
maintainers/contributors who might be interested:
@hwchase17 (follows his work on #4615)
@agola11 (async)
---------
Co-authored-by: Harrison Chase <hw.chase.17@gmail.com>
# Check whether 'other' is empty before popping
This PR could fix a potential 'popping empty set' error.
Co-authored-by: Junlin Zhou <jlzhou@zjuici.com>
# Add MosaicML inference endpoints
This PR adds support in langchain for MosaicML inference endpoints. We
both serve a select few open source models, and allow customers to
deploy their own models using our inference service. Docs are here
(https://docs.mosaicml.com/en/latest/inference.html), and sign up form
is here (https://forms.mosaicml.com/demo?utm_source=langchain). I'm not
intimately familiar with the details of langchain, or the contribution
process, so please let me know if there is anything that needs fixing or
this is the wrong way to submit a new integration, thanks!
I'm also not sure what the procedure is for integration tests. I have
tested locally with my api key.
## Who can review?
@hwchase17
---------
Co-authored-by: Harrison Chase <hw.chase.17@gmail.com>
This PR introduces a new module, `elasticsearch_embeddings.py`, which
provides a wrapper around Elasticsearch embedding models. The new
ElasticsearchEmbeddings class allows users to generate embeddings for
documents and query texts using a [model deployed in an Elasticsearch
cluster](https://www.elastic.co/guide/en/machine-learning/current/ml-nlp-model-ref.html#ml-nlp-model-ref-text-embedding).
### Main features:
1. The ElasticsearchEmbeddings class initializes with an Elasticsearch
connection object and a model_id, providing an interface to interact
with the Elasticsearch ML client through
[infer_trained_model](https://elasticsearch-py.readthedocs.io/en/v8.7.0/api.html?highlight=trained%20model%20infer#elasticsearch.client.MlClient.infer_trained_model)
.
2. The `embed_documents()` method generates embeddings for a list of
documents, and the `embed_query()` method generates an embedding for a
single query text.
3. The class supports custom input text field names in case the deployed
model expects a different field name than the default `text_field`.
4. The implementation is compatible with any model deployed in
Elasticsearch that generates embeddings as output.
### Benefits:
1. Simplifies the process of generating embeddings using Elasticsearch
models.
2. Provides a clean and intuitive interface to interact with the
Elasticsearch ML client.
3. Allows users to easily integrate Elasticsearch-generated embeddings.
Related issue https://github.com/hwchase17/langchain/issues/3400
---------
Co-authored-by: Dev 2049 <dev.dev2049@gmail.com>
Some LLM's will produce numbered lists with leading whitespace, i.e. in
response to "What is the sum of 2 and 3?":
```
Plan:
1. Add 2 and 3.
2. Given the above steps taken, please respond to the users original question.
```
This commit updates the PlanningOutputParser regex to ignore leading
whitespace before the step number, enabling it to correctly parse this
format.
# Allowing openAI fine-tuned models
Very simple fix that checks whether a openAI `model_name` is a
fine-tuned model when loading `context_size` and when computing call's
cost in the `openai_callback`.
Fixes#2887
---------
Co-authored-by: Dev 2049 <dev.dev2049@gmail.com>
# Fix typo + add wikipedia package installation part in
human_input_llm.ipynb
This PR
1. Fixes typo ("the the human input LLM"),
2. Addes wikipedia package installation part (in accordance with
`WikipediaQueryRun`
[documentation](https://python.langchain.com/en/latest/modules/agents/tools/examples/wikipedia.html))
in `human_input_llm.ipynb`
(`docs/modules/models/llms/examples/human_input_llm.ipynb`)
# Add link to Psychic from document loaders documentation page
In my previous PR I forgot to update `document_loaders.rst` to link to
`psychic.ipynb` to make it discoverable from the main documentation.
# Add AzureCognitiveServicesToolkit to call Azure Cognitive Services
API: achieve some multimodal capabilities
This PR adds a toolkit named AzureCognitiveServicesToolkit which bundles
the following tools:
- AzureCogsImageAnalysisTool: calls Azure Cognitive Services image
analysis API to extract caption, objects, tags, and text from images.
- AzureCogsFormRecognizerTool: calls Azure Cognitive Services form
recognizer API to extract text, tables, and key-value pairs from
documents.
- AzureCogsSpeech2TextTool: calls Azure Cognitive Services speech to
text API to transcribe speech to text.
- AzureCogsText2SpeechTool: calls Azure Cognitive Services text to
speech API to synthesize text to speech.
This toolkit can be used to process image, document, and audio inputs.
---------
Co-authored-by: Dev 2049 <dev.dev2049@gmail.com>
# Add a WhyLabs callback handler
* Adds a simple WhyLabsCallbackHandler
* Add required dependencies as optional
* protect against missing modules with imports
* Add docs/ecosystem basic example
based on initial prototype from @andrewelizondo
> this integration gathers privacy preserving telemetry on text with
whylogs and sends stastical profiles to WhyLabs platform to monitoring
these metrics over time. For more information on what WhyLabs is see:
https://whylabs.ai
After you run the notebook (if you have env variables set for the API
Keys, org_id and dataset_id) you get something like this in WhyLabs:

Co-authored-by: Andre Elizondo <andre@whylabs.ai>
Co-authored-by: Dev 2049 <dev.dev2049@gmail.com>
# Improve TextSplitter.split_documents, collect page_content and
metadata in one iteration
## Who can review?
Community members can review the PR once tests pass. Tag
maintainers/contributors who might be interested:
@eyurtsev In the case where documents is a generator that can only be
iterated once making this change is a huge help. Otherwise a silent
issue happens where metadata is empty for all documents when documents
is a generator. So we expand the argument from `List[Document]` to
`Union[Iterable[Document], Sequence[Document]]`
---------
Co-authored-by: Steven Tartakovsky <tartakovsky.developer@gmail.com>
Implementation is similar to search_distance and where_filter
# adds 'additional' support to Weaviate queries
Co-authored-by: Dev 2049 <dev.dev2049@gmail.com>
OpenLM is a zero-dependency OpenAI-compatible LLM provider that can call
different inference endpoints directly via HTTP. It implements the
OpenAI Completion class so that it can be used as a drop-in replacement
for the OpenAI API. This changeset utilizes BaseOpenAI for minimal added
code.
---------
Co-authored-by: Dev 2049 <dev.dev2049@gmail.com>
# Add Mastodon toots loader.
Loader works either with public toots, or Mastodon app credentials. Toot
text and user info is loaded.
I've also added integration test for this new loader as it works with
public data, and a notebook with example output run now.
---------
Co-authored-by: Dev 2049 <dev.dev2049@gmail.com>
# Assign `current_time` to `datetime.now()` if it `current_time is None`
in `time_weighted_retriever`
Fixes#4825
As implemented, `add_documents` in `TimeWeightedVectorStoreRetriever`
assigns `doc.metadata["last_accessed_at"]` and
`doc.metadata["created_at"]` to `datetime.datetime.now()` if
`current_time` is not in `kwargs`.
```python
def add_documents(self, documents: List[Document], **kwargs: Any) -> List[str]:
"""Add documents to vectorstore."""
current_time = kwargs.get("current_time", datetime.datetime.now())
# Avoid mutating input documents
dup_docs = [deepcopy(d) for d in documents]
for i, doc in enumerate(dup_docs):
if "last_accessed_at" not in doc.metadata:
doc.metadata["last_accessed_at"] = current_time
if "created_at" not in doc.metadata:
doc.metadata["created_at"] = current_time
doc.metadata["buffer_idx"] = len(self.memory_stream) + i
self.memory_stream.extend(dup_docs)
return self.vectorstore.add_documents(dup_docs, **kwargs)
```
However, from the way `add_documents` is being called from
`GenerativeAgentMemory`, `current_time` is set as a `kwarg`, but it is
given a value of `None`:
```python
def add_memory(
self, memory_content: str, now: Optional[datetime] = None
) -> List[str]:
"""Add an observation or memory to the agent's memory."""
importance_score = self._score_memory_importance(memory_content)
self.aggregate_importance += importance_score
document = Document(
page_content=memory_content, metadata={"importance": importance_score}
)
result = self.memory_retriever.add_documents([document], current_time=now)
```
The default of `now` was set in #4658 to be None. The proposed fix is
the following:
```python
def add_documents(self, documents: List[Document], **kwargs: Any) -> List[str]:
"""Add documents to vectorstore."""
current_time = kwargs.get("current_time", datetime.datetime.now())
# `current_time` may exist in kwargs, but may still have the value of None.
if current_time is None:
current_time = datetime.datetime.now()
```
Alternatively, we could just set the default of `now` to be
`datetime.datetime.now()` everywhere instead. Thoughts @hwchase17? If we
still want to keep the default to be `None`, then this PR should fix the
above issue. If we want to set the default to be
`datetime.datetime.now()` instead, I can update this PR with that
alternative fix. EDIT: seems like from #5018 it looks like we would
prefer to keep the default to be `None`, in which case this PR should
fix the error.
# changed ValueError to ImportError
Code cleaning.
Fixed inconsistencies in ImportError handling. Sometimes it raises
ImportError and sometime ValueError.
I've changed all cases to the `raise ImportError`
Also:
- added installation instruction in the error message, where it missed;
- fixed several installation instructions in the error message;
- fixed several error handling in regards to the ImportError
Added link option in _process_response
<!--
In _process_respons "snippet" provided non working links for the case
that "links" had the correct answer. Thus added an elif statement before
snippet
-->
<!-- Remove if not applicable -->
Fixes # (issue)
In _process_response link provided correct answers while the snippet
reply provided non working links
@vowelparrot
## Before submitting
<!-- If you're adding a new integration, include an integration test and
an example notebook showing its use! -->
## Who can review?
Community members can review the PR once tests pass. Tag
maintainers/contributors who might be interested:
<!-- For a quicker response, figure out the right person to tag with @
@hwchase17 - project lead
Tracing / Callbacks
- @agola11
Async
- @agola11
DataLoaders
- @eyurtsev
Models
- @hwchase17
- @agola11
Agents / Tools / Toolkits
- @vowelparrot
VectorStores / Retrievers / Memory
- @dev2049
-->
---------
Co-authored-by: Dev 2049 <dev.dev2049@gmail.com>
`from langchain.experimental.autonomous_agents.autogpt.agent import
AutoGPT` results in an import error as AutoGPT is not defined in the
__init__.py file
https://python.langchain.com/en/latest/use_cases/autonomous_agents/marathon_times.html
An Alternate, way would be to be directly update the import statement to
be `from langchain.experimental import AutoGPT`
Co-authored-by: Dev 2049 <dev.dev2049@gmail.com>
# fix a bug in the add_texts method of Weaviate vector store that creats
wrong embeddings
The following is the original code in the `add_texts` method of the
Weaviate vector store, from line 131 to 153, which contains a bug. The
code here includes some extra explanations in the form of comments and
some omissions.
```python
for i, doc in enumerate(texts):
# some code omitted
if self._embedding is not None:
# variable texts is a list of string and doc here is just a string.
# list(doc) actually breaks up the string into characters.
# so, embeddings[0] is just the embedding of the first character
embeddings = self._embedding.embed_documents(list(doc))
batch.add_data_object(
data_object=data_properties,
class_name=self._index_name,
uuid=_id,
vector=embeddings[0],
)
```
To fix this bug, I pulled the embedding operation out of the for loop
and embed all texts at once.
Co-authored-by: Shawn91 <zyx199199@qq.com>
Co-authored-by: Dev 2049 <dev.dev2049@gmail.com>
# PowerBI major refinement in working of tool and tweaks in the rest
I've gained some experience with more complex sets and the earlier
implementation had too many tries by the agent to create DAX, so
refactored the code to run the LLM to create dax based on a question and
then immediately run the same against the dataset, with retries and a
prompt that includes the error for the retry. This works much better!
Also did some other refactoring of the inner workings, making things
clearer, more concise and faster.
# Row-wise cosine similarity between two equal-width matrices and return
the max top_k score and index, the score all greater than
threshold_score.
Co-authored-by: Dev 2049 <dev.dev2049@gmail.com>
Enhance the code to support SSL authentication for Elasticsearch when
using the VectorStore module, as previous versions did not provide this
capability.
@dev2049
---------
Co-authored-by: caidong <zhucaidong1992@gmail.com>
Co-authored-by: Dev 2049 <dev.dev2049@gmail.com>
# Improve pinecone hybrid search retriever adding metadata support
I simply remove the hardwiring of metadata to the existing
implementation allowing one to pass `metadatas` attribute to the
constructors and in `get_relevant_documents`. I also add one missing pip
install to the accompanying notebook (I am not adding dependencies, they
were pre-existing).
First contribution, just hoping to help, feel free to critique :)
my twitter username is `@andreliebschner`
While looking at hybrid search I noticed #3043 and #1743. I think the
former can be closed as following the example right now (even prior to
my improvements) works just fine, the latter I think can be also closed
safely, maybe pointing out the relevant classes and example. Should I
reply those issues mentioning someone?
@dev2049, @hwchase17
---------
Co-authored-by: Andreas Liebschner <a.liebschner@shopfully.com>
This is a highly optimized update to the pull request
https://github.com/hwchase17/langchain/pull/3269
Summary:
1) Added ability to MRKL agent to self solve the ValueError(f"Could not
parse LLM output: `{llm_output}`") error, whenever llm (especially
gpt-3.5-turbo) does not follow the format of MRKL Agent, while returning
"Action:" & "Action Input:".
2) The way I am solving this error is by responding back to the llm with
the messages "Invalid Format: Missing 'Action:' after 'Thought:'" &
"Invalid Format: Missing 'Action Input:' after 'Action:'" whenever
Action: and Action Input: are not present in the llm output
respectively.
For a detailed explanation, look at the previous pull request.
New Updates:
1) Since @hwchase17 , requested in the previous PR to communicate the
self correction (error) message, using the OutputParserException, I have
added new ability to the OutputParserException class to store the
observation & previous llm_output in order to communicate it to the next
Agent's prompt. This is done, without breaking/modifying any of the
functionality OutputParserException previously performs (i.e.
OutputParserException can be used in the same way as before, without
passing any observation & previous llm_output too).
---------
Co-authored-by: Deepak S V <svdeepak99@users.noreply.github.com>
tldr: The docarray [integration
PR](https://github.com/hwchase17/langchain/pull/4483) introduced a
pinned dependency to protobuf. This is a docarray dependency, not a
langchain dependency. Since this is handled by the docarray
dependencies, it is unnecessary here.
Further, as a pinned dependency, this quickly leads to incompatibilities
with application code that consumes the library. Much less with a
heavily used library like protobuf.
Detail: as we see in the [docarray
integration](https://github.com/hwchase17/langchain/pull/4483/files#diff-50c86b7ed8ac2cf95bd48334961bf0530cdc77b5a56f852c5c61b89d735fd711R81-R83),
the transitive dependencies of docarray were also listed as langchain
dependencies. This is unnecessary as the docarray project has an
appropriate
[extras](a01a05542d/pyproject.toml (L70)).
The docarray project also does not require this _pinned_ version of
protobuf, rather [a minimum
version](a01a05542d/pyproject.toml (L41)).
So this pinned version was likely in error.
To fix this, this PR reverts the explicit hnswlib and protobuf
dependencies and adds the hnswlib extras install for docarray (which
installs hnswlib and protobuf, as originally intended). Because version
`0.32.0`
of the docarray hnswlib extras added protobuf, we bump the docarray
dependency from `^0.31.0` to `^0.32.0`.
# revert docarray explicit transitive dependencies and use extras
instead
## Who can review?
@dev2049 -- reviewed the original PR
@eyurtsev -- bumped the pinned protobuf dependency a few days ago
---------
Co-authored-by: Dev 2049 <dev.dev2049@gmail.com>
Update to pull request https://github.com/hwchase17/langchain/pull/3215
Summary:
1) Improved the sanitization of query (using regex), by removing python
command (since gpt-3.5-turbo sometimes assumes python console as a
terminal, and runs python command first which causes error). Also
sometimes 1 line python codes contain single backticks.
2) Added 7 new test cases.
For more details, view the previous pull request.
---------
Co-authored-by: Deepak S V <svdeepak99@users.noreply.github.com>
Let user inspect the token ids in addition to getting th enumber of tokens
---------
Co-authored-by: Zach Schillaci <40636930+zachschillaci27@users.noreply.github.com>