mirror of
https://github.com/hwchase17/langchain
synced 2024-10-29 17:07:25 +00:00
154 lines
5.9 KiB
Plaintext
154 lines
5.9 KiB
Plaintext
|
# Flyte
|
||
|
|
||
|
> [Flyte](https://github.com/flyteorg/flyte) is an open-source orchestrator that facilitates building production-grade data and ML pipelines.
|
||
|
> It is built for scalability and reproducibility, leveraging Kubernetes as its underlying platform.
|
||
|
|
||
|
The purpose of this notebook is to demonstrate the integration of a `FlyteCallback` into your Flyte task, enabling you to effectively monitor and track your LangChain experiments.
|
||
|
|
||
|
## Installation & Setup
|
||
|
|
||
|
- Install the Flytekit library by running the command `pip install flytekit`.
|
||
|
- Install the Flytekit-Envd plugin by running the command `pip install flytekitplugins-envd`.
|
||
|
- Install LangChain by running the command `pip install langchain`.
|
||
|
- Install [Docker](https://docs.docker.com/engine/install/) on your system.
|
||
|
|
||
|
## Flyte Tasks
|
||
|
|
||
|
A Flyte [task](https://docs.flyte.org/projects/cookbook/en/latest/auto/core/flyte_basics/task.html) serves as the foundational building block of Flyte.
|
||
|
To execute LangChain experiments, you need to write Flyte tasks that define the specific steps and operations involved.
|
||
|
|
||
|
NOTE: The [getting started guide](https://docs.flyte.org/projects/cookbook/en/latest/index.html) offers detailed, step-by-step instructions on installing Flyte locally and running your initial Flyte pipeline.
|
||
|
|
||
|
First, import the necessary dependencies to support your LangChain experiments.
|
||
|
|
||
|
```python
|
||
|
import os
|
||
|
|
||
|
from flytekit import ImageSpec, task
|
||
|
from langchain.agents import AgentType, initialize_agent, load_tools
|
||
|
from langchain.callbacks import FlyteCallbackHandler
|
||
|
from langchain.chains import LLMChain
|
||
|
from langchain.chat_models import ChatOpenAI
|
||
|
from langchain.prompts import PromptTemplate
|
||
|
from langchain.schema import HumanMessage
|
||
|
```
|
||
|
|
||
|
Set up the necessary environment variables to utilize the OpenAI API and Serp API:
|
||
|
|
||
|
```python
|
||
|
# Set OpenAI API key
|
||
|
os.environ["OPENAI_API_KEY"] = "<your_openai_api_key>"
|
||
|
|
||
|
# Set Serp API key
|
||
|
os.environ["SERPAPI_API_KEY"] = "<your_serp_api_key>"
|
||
|
```
|
||
|
|
||
|
Replace `<your_openai_api_key>` and `<your_serp_api_key>` with your respective API keys obtained from OpenAI and Serp API.
|
||
|
|
||
|
To guarantee reproducibility of your pipelines, Flyte tasks are containerized.
|
||
|
Each Flyte task must be associated with an image, which can either be shared across the entire Flyte [workflow](https://docs.flyte.org/projects/cookbook/en/latest/auto/core/flyte_basics/basic_workflow.html) or provided separately for each task.
|
||
|
|
||
|
To streamline the process of supplying the required dependencies for each Flyte task, you can initialize an [`ImageSpec`](https://docs.flyte.org/projects/cookbook/en/latest/auto/core/image_spec/image_spec.html) object.
|
||
|
This approach automatically triggers a Docker build, alleviating the need for users to manually create a Docker image.
|
||
|
|
||
|
```python
|
||
|
custom_image = ImageSpec(
|
||
|
name="langchain-flyte",
|
||
|
packages=[
|
||
|
"langchain",
|
||
|
"openai",
|
||
|
"spacy",
|
||
|
"https://github.com/explosion/spacy-models/releases/download/en_core_web_sm-3.5.0/en_core_web_sm-3.5.0.tar.gz",
|
||
|
"textstat",
|
||
|
"google-search-results",
|
||
|
],
|
||
|
registry="<your-registry>",
|
||
|
)
|
||
|
```
|
||
|
|
||
|
You have the flexibility to push the Docker image to a registry of your preference.
|
||
|
[Docker Hub](https://hub.docker.com/) or [GitHub Container Registry (GHCR)](https://docs.github.com/en/packages/working-with-a-github-packages-registry/working-with-the-container-registry) is a convenient option to begin with.
|
||
|
|
||
|
Once you have selected a registry, you can proceed to create Flyte tasks that log the LangChain metrics to Flyte Deck.
|
||
|
|
||
|
The following examples demonstrate tasks related to OpenAI LLM, chains and agent with tools:
|
||
|
|
||
|
### LLM
|
||
|
|
||
|
```python
|
||
|
@task(disable_deck=False, container_image=custom_image)
|
||
|
def langchain_llm() -> str:
|
||
|
llm = ChatOpenAI(
|
||
|
model_name="gpt-3.5-turbo",
|
||
|
temperature=0.2,
|
||
|
callbacks=[FlyteCallbackHandler()],
|
||
|
)
|
||
|
return llm([HumanMessage(content="Tell me a joke")]).content
|
||
|
```
|
||
|
|
||
|
### Chain
|
||
|
|
||
|
```python
|
||
|
@task(disable_deck=False, container_image=custom_image)
|
||
|
def langchain_chain() -> list[dict[str, str]]:
|
||
|
template = """You are a playwright. Given the title of play, it is your job to write a synopsis for that title.
|
||
|
Title: {title}
|
||
|
Playwright: This is a synopsis for the above play:"""
|
||
|
llm = ChatOpenAI(
|
||
|
model_name="gpt-3.5-turbo",
|
||
|
temperature=0,
|
||
|
callbacks=[FlyteCallbackHandler()],
|
||
|
)
|
||
|
prompt_template = PromptTemplate(input_variables=["title"], template=template)
|
||
|
synopsis_chain = LLMChain(
|
||
|
llm=llm, prompt=prompt_template, callbacks=[FlyteCallbackHandler()]
|
||
|
)
|
||
|
test_prompts = [
|
||
|
{
|
||
|
"title": "documentary about good video games that push the boundary of game design"
|
||
|
},
|
||
|
]
|
||
|
return synopsis_chain.apply(test_prompts)
|
||
|
```
|
||
|
|
||
|
### Agent
|
||
|
|
||
|
```python
|
||
|
@task(disable_deck=False, container_image=custom_image)
|
||
|
def langchain_agent() -> str:
|
||
|
llm = OpenAI(
|
||
|
model_name="gpt-3.5-turbo",
|
||
|
temperature=0,
|
||
|
callbacks=[FlyteCallbackHandler()],
|
||
|
)
|
||
|
tools = load_tools(
|
||
|
["serpapi", "llm-math"], llm=llm, callbacks=[FlyteCallbackHandler()]
|
||
|
)
|
||
|
agent = initialize_agent(
|
||
|
tools,
|
||
|
llm,
|
||
|
agent=AgentType.ZERO_SHOT_REACT_DESCRIPTION,
|
||
|
callbacks=[FlyteCallbackHandler()],
|
||
|
verbose=True,
|
||
|
)
|
||
|
return agent.run(
|
||
|
"Who is Leonardo DiCaprio's girlfriend? Could you calculate her current age and raise it to the power of 0.43?"
|
||
|
)
|
||
|
```
|
||
|
|
||
|
These tasks serve as a starting point for running your LangChain experiments within Flyte.
|
||
|
|
||
|
## Execute the Flyte Tasks on Kubernetes
|
||
|
|
||
|
To execute the Flyte tasks on the configured Flyte backend, use the following command:
|
||
|
|
||
|
```bash
|
||
|
pyflyte run --image <your-image> langchain_flyte.py langchain_llm
|
||
|
```
|
||
|
|
||
|
This command will initiate the execution of the `langchain_llm` task on the Flyte backend. You can trigger the remaining two tasks in a similar manner.
|
||
|
|
||
|
The metrics will be displayed on the Flyte UI as follows:
|
||
|
|
||
|
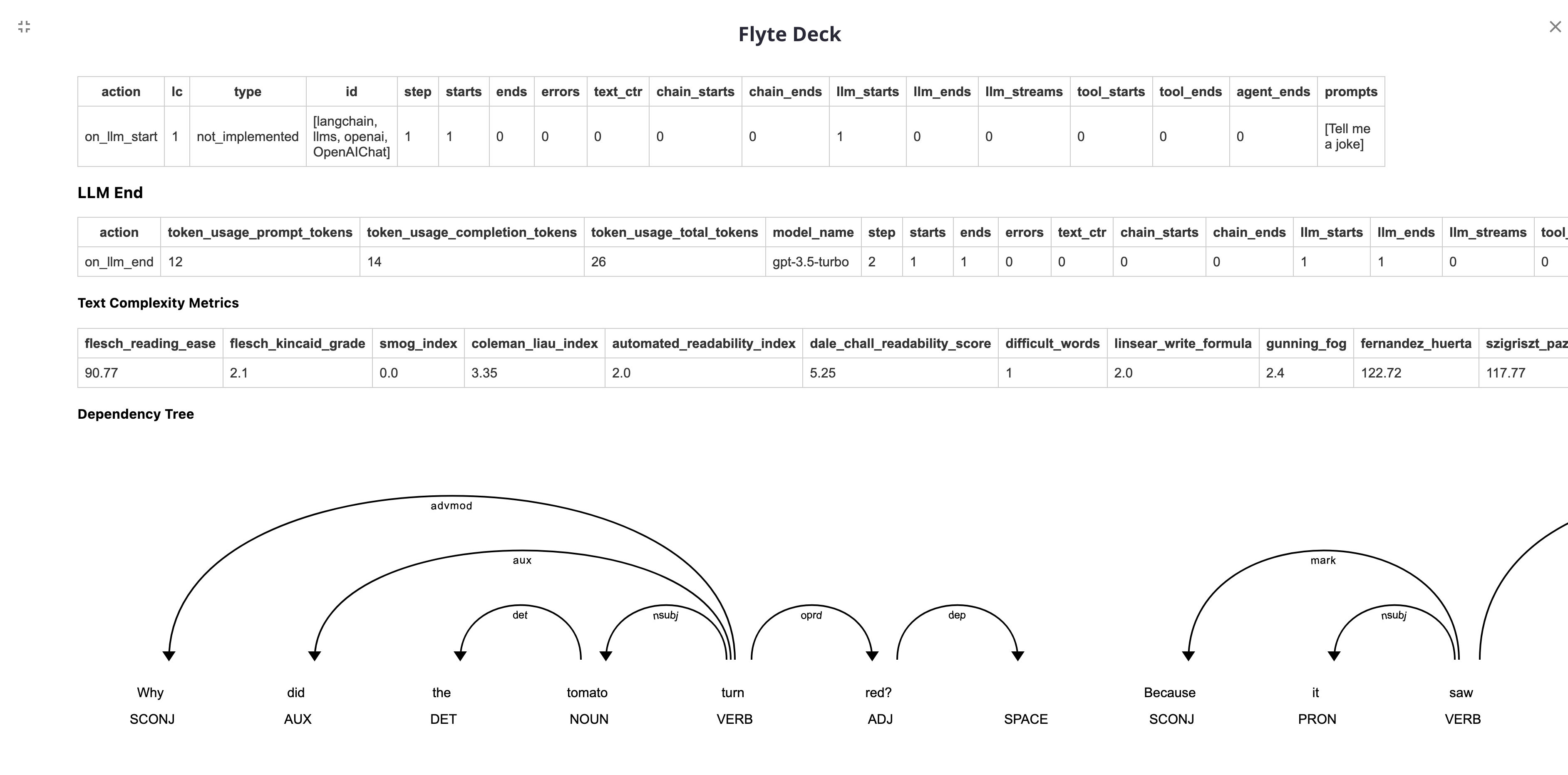
|