mirror of https://github.com/hwchase17/langchain
Signed-off-by: Samhita Alla <aallasamhita@gmail.com> Co-authored-by: Samhita Alla <aallasamhita@gmail.com>vwp/fix_promptlayer
parent
e3b7effc8f
commit
e4625846e5
@ -0,0 +1,153 @@
|
||||
# Flyte
|
||||
|
||||
> [Flyte](https://github.com/flyteorg/flyte) is an open-source orchestrator that facilitates building production-grade data and ML pipelines.
|
||||
> It is built for scalability and reproducibility, leveraging Kubernetes as its underlying platform.
|
||||
|
||||
The purpose of this notebook is to demonstrate the integration of a `FlyteCallback` into your Flyte task, enabling you to effectively monitor and track your LangChain experiments.
|
||||
|
||||
## Installation & Setup
|
||||
|
||||
- Install the Flytekit library by running the command `pip install flytekit`.
|
||||
- Install the Flytekit-Envd plugin by running the command `pip install flytekitplugins-envd`.
|
||||
- Install LangChain by running the command `pip install langchain`.
|
||||
- Install [Docker](https://docs.docker.com/engine/install/) on your system.
|
||||
|
||||
## Flyte Tasks
|
||||
|
||||
A Flyte [task](https://docs.flyte.org/projects/cookbook/en/latest/auto/core/flyte_basics/task.html) serves as the foundational building block of Flyte.
|
||||
To execute LangChain experiments, you need to write Flyte tasks that define the specific steps and operations involved.
|
||||
|
||||
NOTE: The [getting started guide](https://docs.flyte.org/projects/cookbook/en/latest/index.html) offers detailed, step-by-step instructions on installing Flyte locally and running your initial Flyte pipeline.
|
||||
|
||||
First, import the necessary dependencies to support your LangChain experiments.
|
||||
|
||||
```python
|
||||
import os
|
||||
|
||||
from flytekit import ImageSpec, task
|
||||
from langchain.agents import AgentType, initialize_agent, load_tools
|
||||
from langchain.callbacks import FlyteCallbackHandler
|
||||
from langchain.chains import LLMChain
|
||||
from langchain.chat_models import ChatOpenAI
|
||||
from langchain.prompts import PromptTemplate
|
||||
from langchain.schema import HumanMessage
|
||||
```
|
||||
|
||||
Set up the necessary environment variables to utilize the OpenAI API and Serp API:
|
||||
|
||||
```python
|
||||
# Set OpenAI API key
|
||||
os.environ["OPENAI_API_KEY"] = "<your_openai_api_key>"
|
||||
|
||||
# Set Serp API key
|
||||
os.environ["SERPAPI_API_KEY"] = "<your_serp_api_key>"
|
||||
```
|
||||
|
||||
Replace `<your_openai_api_key>` and `<your_serp_api_key>` with your respective API keys obtained from OpenAI and Serp API.
|
||||
|
||||
To guarantee reproducibility of your pipelines, Flyte tasks are containerized.
|
||||
Each Flyte task must be associated with an image, which can either be shared across the entire Flyte [workflow](https://docs.flyte.org/projects/cookbook/en/latest/auto/core/flyte_basics/basic_workflow.html) or provided separately for each task.
|
||||
|
||||
To streamline the process of supplying the required dependencies for each Flyte task, you can initialize an [`ImageSpec`](https://docs.flyte.org/projects/cookbook/en/latest/auto/core/image_spec/image_spec.html) object.
|
||||
This approach automatically triggers a Docker build, alleviating the need for users to manually create a Docker image.
|
||||
|
||||
```python
|
||||
custom_image = ImageSpec(
|
||||
name="langchain-flyte",
|
||||
packages=[
|
||||
"langchain",
|
||||
"openai",
|
||||
"spacy",
|
||||
"https://github.com/explosion/spacy-models/releases/download/en_core_web_sm-3.5.0/en_core_web_sm-3.5.0.tar.gz",
|
||||
"textstat",
|
||||
"google-search-results",
|
||||
],
|
||||
registry="<your-registry>",
|
||||
)
|
||||
```
|
||||
|
||||
You have the flexibility to push the Docker image to a registry of your preference.
|
||||
[Docker Hub](https://hub.docker.com/) or [GitHub Container Registry (GHCR)](https://docs.github.com/en/packages/working-with-a-github-packages-registry/working-with-the-container-registry) is a convenient option to begin with.
|
||||
|
||||
Once you have selected a registry, you can proceed to create Flyte tasks that log the LangChain metrics to Flyte Deck.
|
||||
|
||||
The following examples demonstrate tasks related to OpenAI LLM, chains and agent with tools:
|
||||
|
||||
### LLM
|
||||
|
||||
```python
|
||||
@task(disable_deck=False, container_image=custom_image)
|
||||
def langchain_llm() -> str:
|
||||
llm = ChatOpenAI(
|
||||
model_name="gpt-3.5-turbo",
|
||||
temperature=0.2,
|
||||
callbacks=[FlyteCallbackHandler()],
|
||||
)
|
||||
return llm([HumanMessage(content="Tell me a joke")]).content
|
||||
```
|
||||
|
||||
### Chain
|
||||
|
||||
```python
|
||||
@task(disable_deck=False, container_image=custom_image)
|
||||
def langchain_chain() -> list[dict[str, str]]:
|
||||
template = """You are a playwright. Given the title of play, it is your job to write a synopsis for that title.
|
||||
Title: {title}
|
||||
Playwright: This is a synopsis for the above play:"""
|
||||
llm = ChatOpenAI(
|
||||
model_name="gpt-3.5-turbo",
|
||||
temperature=0,
|
||||
callbacks=[FlyteCallbackHandler()],
|
||||
)
|
||||
prompt_template = PromptTemplate(input_variables=["title"], template=template)
|
||||
synopsis_chain = LLMChain(
|
||||
llm=llm, prompt=prompt_template, callbacks=[FlyteCallbackHandler()]
|
||||
)
|
||||
test_prompts = [
|
||||
{
|
||||
"title": "documentary about good video games that push the boundary of game design"
|
||||
},
|
||||
]
|
||||
return synopsis_chain.apply(test_prompts)
|
||||
```
|
||||
|
||||
### Agent
|
||||
|
||||
```python
|
||||
@task(disable_deck=False, container_image=custom_image)
|
||||
def langchain_agent() -> str:
|
||||
llm = OpenAI(
|
||||
model_name="gpt-3.5-turbo",
|
||||
temperature=0,
|
||||
callbacks=[FlyteCallbackHandler()],
|
||||
)
|
||||
tools = load_tools(
|
||||
["serpapi", "llm-math"], llm=llm, callbacks=[FlyteCallbackHandler()]
|
||||
)
|
||||
agent = initialize_agent(
|
||||
tools,
|
||||
llm,
|
||||
agent=AgentType.ZERO_SHOT_REACT_DESCRIPTION,
|
||||
callbacks=[FlyteCallbackHandler()],
|
||||
verbose=True,
|
||||
)
|
||||
return agent.run(
|
||||
"Who is Leonardo DiCaprio's girlfriend? Could you calculate her current age and raise it to the power of 0.43?"
|
||||
)
|
||||
```
|
||||
|
||||
These tasks serve as a starting point for running your LangChain experiments within Flyte.
|
||||
|
||||
## Execute the Flyte Tasks on Kubernetes
|
||||
|
||||
To execute the Flyte tasks on the configured Flyte backend, use the following command:
|
||||
|
||||
```bash
|
||||
pyflyte run --image <your-image> langchain_flyte.py langchain_llm
|
||||
```
|
||||
|
||||
This command will initiate the execution of the `langchain_llm` task on the Flyte backend. You can trigger the remaining two tasks in a similar manner.
|
||||
|
||||
The metrics will be displayed on the Flyte UI as follows:
|
||||
|
||||
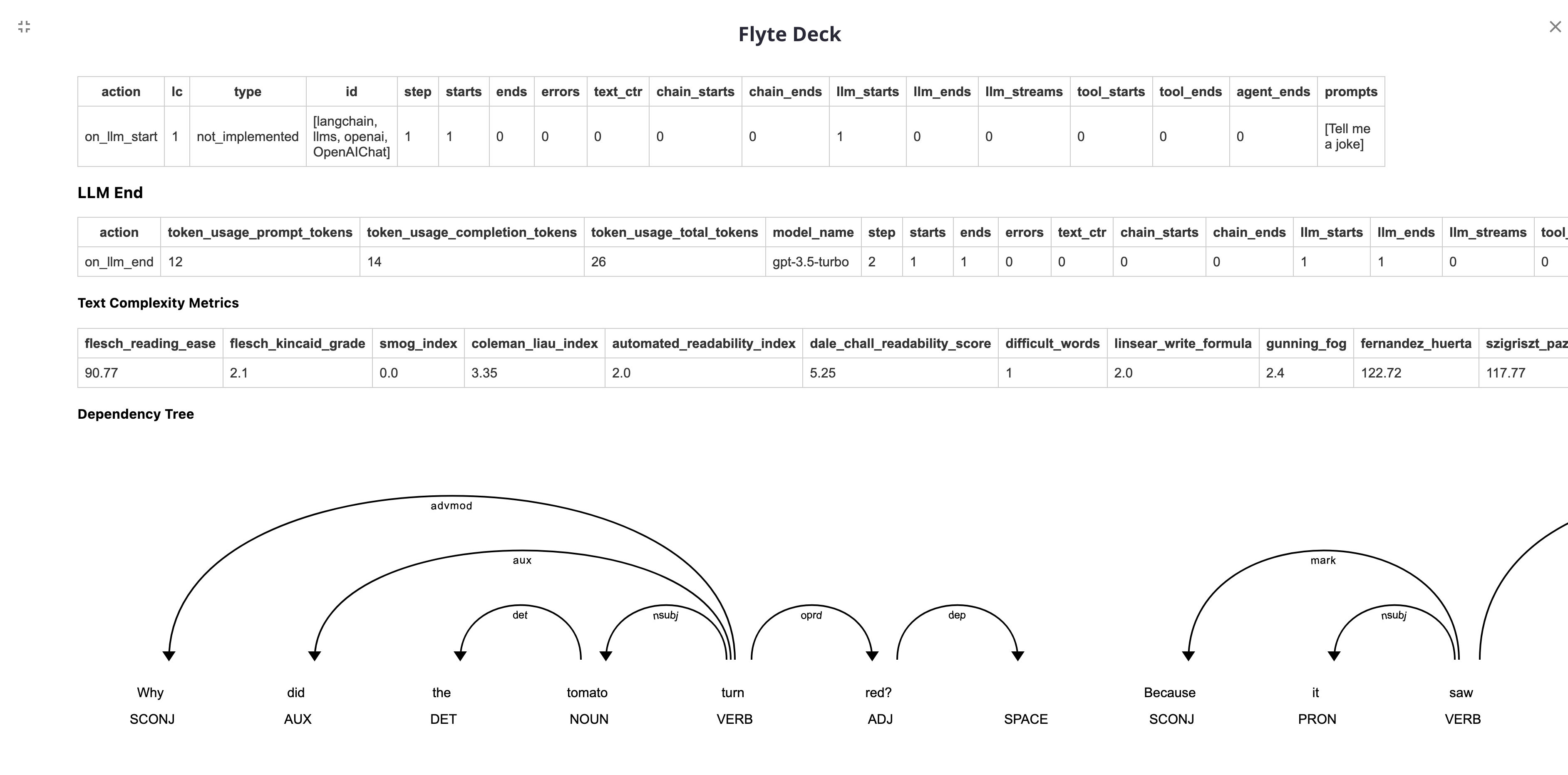
|
@ -0,0 +1,366 @@
|
||||
"""FlyteKit callback handler."""
|
||||
from __future__ import annotations
|
||||
|
||||
import logging
|
||||
from copy import deepcopy
|
||||
from typing import TYPE_CHECKING, Any, Dict, List, Tuple, Union
|
||||
|
||||
from langchain.callbacks.base import BaseCallbackHandler
|
||||
from langchain.callbacks.utils import (
|
||||
BaseMetadataCallbackHandler,
|
||||
flatten_dict,
|
||||
import_pandas,
|
||||
import_spacy,
|
||||
import_textstat,
|
||||
)
|
||||
from langchain.schema import AgentAction, AgentFinish, LLMResult
|
||||
|
||||
if TYPE_CHECKING:
|
||||
import flytekit
|
||||
from flytekitplugins.deck import renderer
|
||||
|
||||
logger = logging.getLogger(__name__)
|
||||
|
||||
|
||||
def import_flytekit() -> Tuple[flytekit, renderer]:
|
||||
try:
|
||||
import flytekit # noqa: F401
|
||||
from flytekitplugins.deck import renderer # noqa: F401
|
||||
except ImportError:
|
||||
raise ImportError(
|
||||
"To use the flyte callback manager you need"
|
||||
"to have the `flytekit` and `flytekitplugins-deck-standard`"
|
||||
"packages installed. Please install them with `pip install flytekit`"
|
||||
"and `pip install flytekitplugins-deck-standard`."
|
||||
)
|
||||
return flytekit, renderer
|
||||
|
||||
|
||||
def analyze_text(
|
||||
text: str,
|
||||
nlp: Any = None,
|
||||
) -> dict:
|
||||
"""Analyze text using textstat and spacy.
|
||||
|
||||
Parameters:
|
||||
text (str): The text to analyze.
|
||||
nlp (spacy.lang): The spacy language model to use for visualization.
|
||||
|
||||
Returns:
|
||||
(dict): A dictionary containing the complexity metrics and visualization
|
||||
files serialized to HTML string.
|
||||
"""
|
||||
resp: Dict[str, Any] = {}
|
||||
textstat = import_textstat()
|
||||
text_complexity_metrics = {
|
||||
"flesch_reading_ease": textstat.flesch_reading_ease(text),
|
||||
"flesch_kincaid_grade": textstat.flesch_kincaid_grade(text),
|
||||
"smog_index": textstat.smog_index(text),
|
||||
"coleman_liau_index": textstat.coleman_liau_index(text),
|
||||
"automated_readability_index": textstat.automated_readability_index(text),
|
||||
"dale_chall_readability_score": textstat.dale_chall_readability_score(text),
|
||||
"difficult_words": textstat.difficult_words(text),
|
||||
"linsear_write_formula": textstat.linsear_write_formula(text),
|
||||
"gunning_fog": textstat.gunning_fog(text),
|
||||
"fernandez_huerta": textstat.fernandez_huerta(text),
|
||||
"szigriszt_pazos": textstat.szigriszt_pazos(text),
|
||||
"gutierrez_polini": textstat.gutierrez_polini(text),
|
||||
"crawford": textstat.crawford(text),
|
||||
"gulpease_index": textstat.gulpease_index(text),
|
||||
"osman": textstat.osman(text),
|
||||
}
|
||||
resp.update({"text_complexity_metrics": text_complexity_metrics})
|
||||
resp.update(text_complexity_metrics)
|
||||
|
||||
if nlp is not None:
|
||||
spacy = import_spacy()
|
||||
doc = nlp(text)
|
||||
dep_out = spacy.displacy.render( # type: ignore
|
||||
doc, style="dep", jupyter=False, page=True
|
||||
)
|
||||
|
||||
ent_out = spacy.displacy.render( # type: ignore
|
||||
doc, style="ent", jupyter=False, page=True
|
||||
)
|
||||
|
||||
text_visualizations = {
|
||||
"dependency_tree": dep_out,
|
||||
"entities": ent_out,
|
||||
}
|
||||
|
||||
resp.update(text_visualizations)
|
||||
|
||||
return resp
|
||||
|
||||
|
||||
class FlyteCallbackHandler(BaseMetadataCallbackHandler, BaseCallbackHandler):
|
||||
"""This callback handler is designed specifically for usage within a Flyte task."""
|
||||
|
||||
def __init__(self) -> None:
|
||||
"""Initialize callback handler."""
|
||||
import_textstat() # Raise error since it is required
|
||||
flytekit, renderer = import_flytekit()
|
||||
self.pandas = import_pandas()
|
||||
|
||||
spacy = None
|
||||
try:
|
||||
spacy = import_spacy()
|
||||
except ImportError:
|
||||
logger.warning(
|
||||
"Spacy library is not installed. \
|
||||
It may result in the inability to log \
|
||||
certain metrics that can be captured with Spacy."
|
||||
)
|
||||
|
||||
super().__init__()
|
||||
|
||||
self.nlp = None
|
||||
if spacy:
|
||||
try:
|
||||
self.nlp = spacy.load("en_core_web_sm")
|
||||
except OSError:
|
||||
logger.warning(
|
||||
"FlyteCallbackHandler uses spacy's en_core_web_sm model"
|
||||
" for certain metrics. To download,"
|
||||
" run the following command in your terminal:"
|
||||
" `python -m spacy download en_core_web_sm` command."
|
||||
)
|
||||
|
||||
self.table_renderer = renderer.TableRenderer
|
||||
self.markdown_renderer = renderer.MarkdownRenderer
|
||||
|
||||
self.deck = flytekit.Deck(
|
||||
"LangChain Metrics",
|
||||
self.markdown_renderer().to_html("## LangChain Metrics"),
|
||||
)
|
||||
|
||||
def on_llm_start(
|
||||
self, serialized: Dict[str, Any], prompts: List[str], **kwargs: Any
|
||||
) -> None:
|
||||
"""Run when LLM starts."""
|
||||
|
||||
self.step += 1
|
||||
self.llm_starts += 1
|
||||
self.starts += 1
|
||||
|
||||
resp: Dict[str, Any] = {}
|
||||
resp.update({"action": "on_llm_start"})
|
||||
resp.update(flatten_dict(serialized))
|
||||
resp.update(self.get_custom_callback_meta())
|
||||
|
||||
prompt_responses = []
|
||||
for prompt in prompts:
|
||||
prompt_responses.append(prompt)
|
||||
|
||||
resp.update({"prompts": prompt_responses})
|
||||
|
||||
self.deck.append(self.markdown_renderer().to_html("### LLM Start"))
|
||||
self.deck.append(
|
||||
self.table_renderer().to_html(self.pandas.DataFrame([resp])) + "\n"
|
||||
)
|
||||
|
||||
def on_llm_new_token(self, token: str, **kwargs: Any) -> None:
|
||||
"""Run when LLM generates a new token."""
|
||||
|
||||
def on_llm_end(self, response: LLMResult, **kwargs: Any) -> None:
|
||||
"""Run when LLM ends running."""
|
||||
self.step += 1
|
||||
self.llm_ends += 1
|
||||
self.ends += 1
|
||||
|
||||
resp: Dict[str, Any] = {}
|
||||
resp.update({"action": "on_llm_end"})
|
||||
resp.update(flatten_dict(response.llm_output or {}))
|
||||
resp.update(self.get_custom_callback_meta())
|
||||
|
||||
self.deck.append(self.markdown_renderer().to_html("### LLM End"))
|
||||
self.deck.append(self.table_renderer().to_html(self.pandas.DataFrame([resp])))
|
||||
|
||||
for generations in response.generations:
|
||||
for generation in generations:
|
||||
generation_resp = deepcopy(resp)
|
||||
generation_resp.update(flatten_dict(generation.dict()))
|
||||
if self.nlp:
|
||||
generation_resp.update(
|
||||
analyze_text(
|
||||
generation.text,
|
||||
nlp=self.nlp,
|
||||
)
|
||||
)
|
||||
|
||||
complexity_metrics: Dict[str, float] = generation_resp.pop("text_complexity_metrics") # type: ignore # noqa: E501
|
||||
self.deck.append(
|
||||
self.markdown_renderer().to_html("#### Text Complexity Metrics")
|
||||
)
|
||||
self.deck.append(
|
||||
self.table_renderer().to_html(
|
||||
self.pandas.DataFrame([complexity_metrics])
|
||||
)
|
||||
+ "\n"
|
||||
)
|
||||
|
||||
dependency_tree = generation_resp["dependency_tree"]
|
||||
self.deck.append(
|
||||
self.markdown_renderer().to_html("#### Dependency Tree")
|
||||
)
|
||||
self.deck.append(dependency_tree)
|
||||
|
||||
entities = generation_resp["entities"]
|
||||
self.deck.append(self.markdown_renderer().to_html("#### Entities"))
|
||||
self.deck.append(entities)
|
||||
else:
|
||||
self.deck.append(
|
||||
self.markdown_renderer().to_html("#### Generated Response")
|
||||
)
|
||||
self.deck.append(self.markdown_renderer().to_html(generation.text))
|
||||
|
||||
def on_llm_error(
|
||||
self, error: Union[Exception, KeyboardInterrupt], **kwargs: Any
|
||||
) -> None:
|
||||
"""Run when LLM errors."""
|
||||
self.step += 1
|
||||
self.errors += 1
|
||||
|
||||
def on_chain_start(
|
||||
self, serialized: Dict[str, Any], inputs: Dict[str, Any], **kwargs: Any
|
||||
) -> None:
|
||||
"""Run when chain starts running."""
|
||||
self.step += 1
|
||||
self.chain_starts += 1
|
||||
self.starts += 1
|
||||
|
||||
resp: Dict[str, Any] = {}
|
||||
resp.update({"action": "on_chain_start"})
|
||||
resp.update(flatten_dict(serialized))
|
||||
resp.update(self.get_custom_callback_meta())
|
||||
|
||||
chain_input = ",".join([f"{k}={v}" for k, v in inputs.items()])
|
||||
input_resp = deepcopy(resp)
|
||||
input_resp["inputs"] = chain_input
|
||||
|
||||
self.deck.append(self.markdown_renderer().to_html("### Chain Start"))
|
||||
self.deck.append(
|
||||
self.table_renderer().to_html(self.pandas.DataFrame([input_resp])) + "\n"
|
||||
)
|
||||
|
||||
def on_chain_end(self, outputs: Dict[str, Any], **kwargs: Any) -> None:
|
||||
"""Run when chain ends running."""
|
||||
self.step += 1
|
||||
self.chain_ends += 1
|
||||
self.ends += 1
|
||||
|
||||
resp: Dict[str, Any] = {}
|
||||
chain_output = ",".join([f"{k}={v}" for k, v in outputs.items()])
|
||||
resp.update({"action": "on_chain_end", "outputs": chain_output})
|
||||
resp.update(self.get_custom_callback_meta())
|
||||
|
||||
self.deck.append(self.markdown_renderer().to_html("### Chain End"))
|
||||
self.deck.append(
|
||||
self.table_renderer().to_html(self.pandas.DataFrame([resp])) + "\n"
|
||||
)
|
||||
|
||||
def on_chain_error(
|
||||
self, error: Union[Exception, KeyboardInterrupt], **kwargs: Any
|
||||
) -> None:
|
||||
"""Run when chain errors."""
|
||||
self.step += 1
|
||||
self.errors += 1
|
||||
|
||||
def on_tool_start(
|
||||
self, serialized: Dict[str, Any], input_str: str, **kwargs: Any
|
||||
) -> None:
|
||||
"""Run when tool starts running."""
|
||||
self.step += 1
|
||||
self.tool_starts += 1
|
||||
self.starts += 1
|
||||
|
||||
resp: Dict[str, Any] = {}
|
||||
resp.update({"action": "on_tool_start", "input_str": input_str})
|
||||
resp.update(flatten_dict(serialized))
|
||||
resp.update(self.get_custom_callback_meta())
|
||||
|
||||
self.deck.append(self.markdown_renderer().to_html("### Tool Start"))
|
||||
self.deck.append(
|
||||
self.table_renderer().to_html(self.pandas.DataFrame([resp])) + "\n"
|
||||
)
|
||||
|
||||
def on_tool_end(self, output: str, **kwargs: Any) -> None:
|
||||
"""Run when tool ends running."""
|
||||
self.step += 1
|
||||
self.tool_ends += 1
|
||||
self.ends += 1
|
||||
|
||||
resp: Dict[str, Any] = {}
|
||||
resp.update({"action": "on_tool_end", "output": output})
|
||||
resp.update(self.get_custom_callback_meta())
|
||||
|
||||
self.deck.append(self.markdown_renderer().to_html("### Tool End"))
|
||||
self.deck.append(
|
||||
self.table_renderer().to_html(self.pandas.DataFrame([resp])) + "\n"
|
||||
)
|
||||
|
||||
def on_tool_error(
|
||||
self, error: Union[Exception, KeyboardInterrupt], **kwargs: Any
|
||||
) -> None:
|
||||
"""Run when tool errors."""
|
||||
self.step += 1
|
||||
self.errors += 1
|
||||
|
||||
def on_text(self, text: str, **kwargs: Any) -> None:
|
||||
"""
|
||||
Run when agent is ending.
|
||||
"""
|
||||
self.step += 1
|
||||
self.text_ctr += 1
|
||||
|
||||
resp: Dict[str, Any] = {}
|
||||
resp.update({"action": "on_text", "text": text})
|
||||
resp.update(self.get_custom_callback_meta())
|
||||
|
||||
self.deck.append(self.markdown_renderer().to_html("### On Text"))
|
||||
self.deck.append(
|
||||
self.table_renderer().to_html(self.pandas.DataFrame([resp])) + "\n"
|
||||
)
|
||||
|
||||
def on_agent_finish(self, finish: AgentFinish, **kwargs: Any) -> None:
|
||||
"""Run when agent ends running."""
|
||||
self.step += 1
|
||||
self.agent_ends += 1
|
||||
self.ends += 1
|
||||
|
||||
resp: Dict[str, Any] = {}
|
||||
resp.update(
|
||||
{
|
||||
"action": "on_agent_finish",
|
||||
"output": finish.return_values["output"],
|
||||
"log": finish.log,
|
||||
}
|
||||
)
|
||||
resp.update(self.get_custom_callback_meta())
|
||||
|
||||
self.deck.append(self.markdown_renderer().to_html("### Agent Finish"))
|
||||
self.deck.append(
|
||||
self.table_renderer().to_html(self.pandas.DataFrame([resp])) + "\n"
|
||||
)
|
||||
|
||||
def on_agent_action(self, action: AgentAction, **kwargs: Any) -> Any:
|
||||
"""Run on agent action."""
|
||||
self.step += 1
|
||||
self.tool_starts += 1
|
||||
self.starts += 1
|
||||
|
||||
resp: Dict[str, Any] = {}
|
||||
resp.update(
|
||||
{
|
||||
"action": "on_agent_action",
|
||||
"tool": action.tool,
|
||||
"tool_input": action.tool_input,
|
||||
"log": action.log,
|
||||
}
|
||||
)
|
||||
resp.update(self.get_custom_callback_meta())
|
||||
|
||||
self.deck.append(self.markdown_renderer().to_html("### Agent Action"))
|
||||
self.deck.append(
|
||||
self.table_renderer().to_html(self.pandas.DataFrame([resp])) + "\n"
|
||||
)
|
Loading…
Reference in New Issue