forked from Archives/langchain
Harrison/aim integration (#2178)
Co-authored-by: Hovhannes Tamoyan <hovhannes.tamoyan@gmail.com> Co-authored-by: Gor Arakelyan <arakelyangor10@gmail.com>doc
parent
68f039704c
commit
fe804d2a01
@ -0,0 +1,292 @@
|
||||
{
|
||||
"cells": [
|
||||
{
|
||||
"cell_type": "markdown",
|
||||
"metadata": {},
|
||||
"source": [
|
||||
"# Aim\n",
|
||||
"\n",
|
||||
"Aim makes it super easy to visualize and debug LangChain executions. Aim tracks inputs and outputs of LLMs and tools, as well as actions of agents. \n",
|
||||
"\n",
|
||||
"With Aim, you can easily debug and examine an individual execution:\n",
|
||||
"\n",
|
||||
"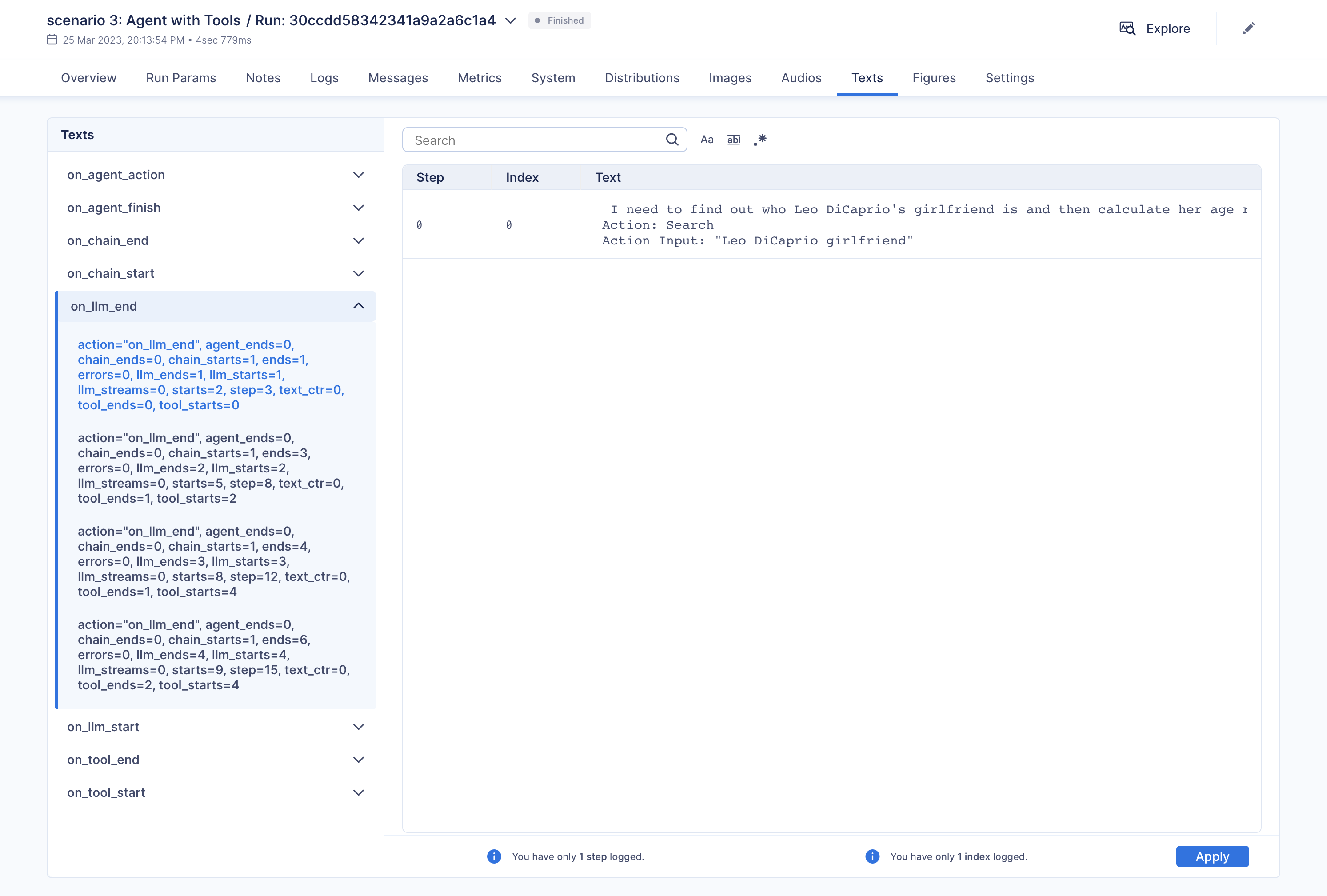\n",
|
||||
"\n",
|
||||
"Additionally, you have the option to compare multiple executions side by side:\n",
|
||||
"\n",
|
||||
"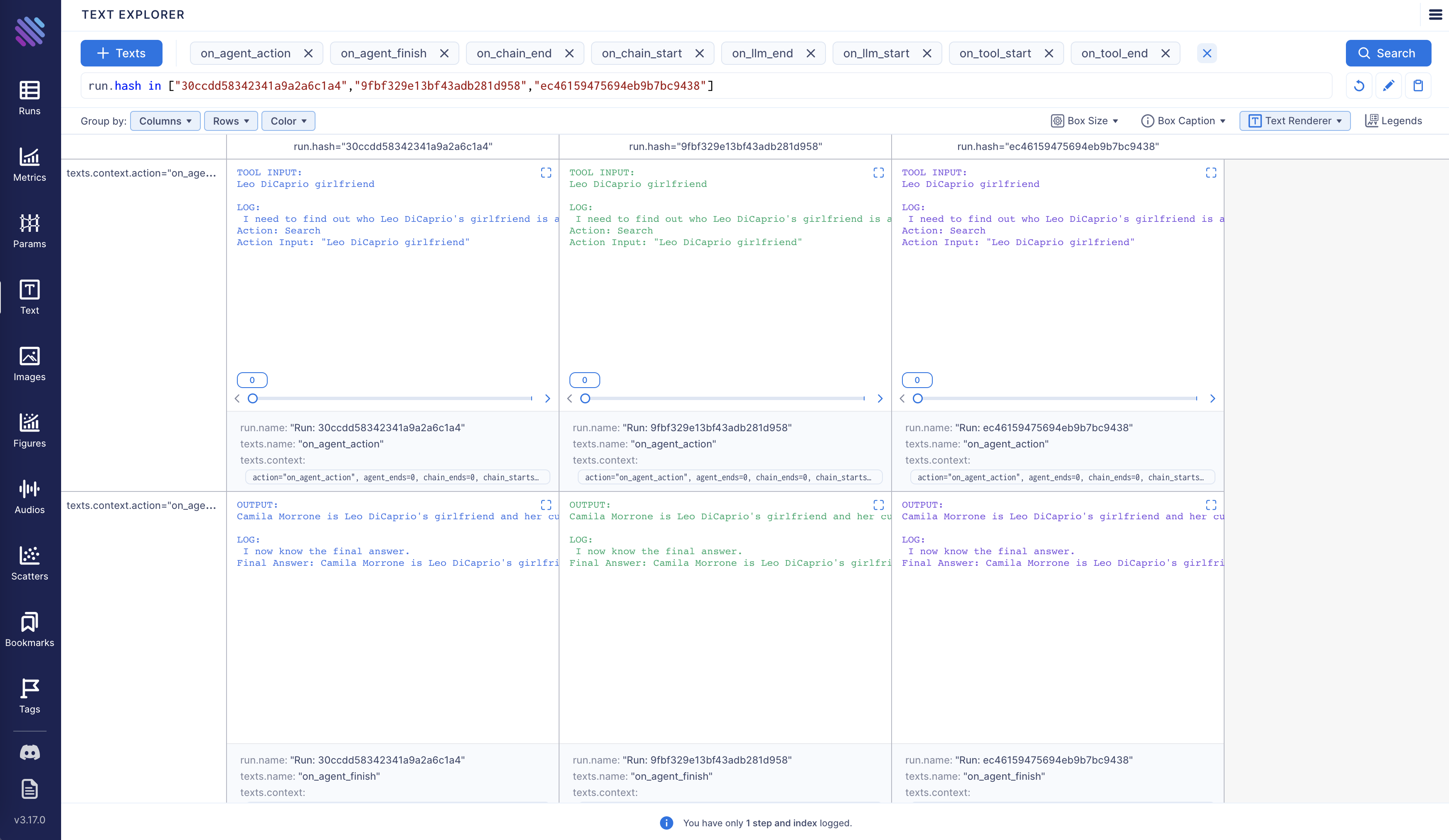\n",
|
||||
"\n",
|
||||
"Aim is fully open source, [learn more](https://github.com/aimhubio/aim) about Aim on GitHub.\n",
|
||||
"\n",
|
||||
"Let's move forward and see how to enable and configure Aim callback."
|
||||
]
|
||||
},
|
||||
{
|
||||
"cell_type": "markdown",
|
||||
"metadata": {},
|
||||
"source": [
|
||||
"<h3>Tracking LangChain Executions with Aim</h3>"
|
||||
]
|
||||
},
|
||||
{
|
||||
"cell_type": "markdown",
|
||||
"metadata": {},
|
||||
"source": [
|
||||
"In this notebook we will explore three usage scenarios. To start off, we will install the necessary packages and import certain modules. Subsequently, we will configure two environment variables that can be established either within the Python script or through the terminal."
|
||||
]
|
||||
},
|
||||
{
|
||||
"cell_type": "code",
|
||||
"execution_count": null,
|
||||
"metadata": {
|
||||
"id": "mf88kuCJhbVu"
|
||||
},
|
||||
"outputs": [],
|
||||
"source": [
|
||||
"!pip install aim\n",
|
||||
"!pip install langchain\n",
|
||||
"!pip install openai\n",
|
||||
"!pip install google-search-results"
|
||||
]
|
||||
},
|
||||
{
|
||||
"cell_type": "code",
|
||||
"execution_count": null,
|
||||
"metadata": {
|
||||
"id": "g4eTuajwfl6L"
|
||||
},
|
||||
"outputs": [],
|
||||
"source": [
|
||||
"import os\n",
|
||||
"from datetime import datetime\n",
|
||||
"\n",
|
||||
"from langchain.llms import OpenAI\n",
|
||||
"from langchain.callbacks.base import CallbackManager\n",
|
||||
"from langchain.callbacks import AimCallbackHandler, StdOutCallbackHandler"
|
||||
]
|
||||
},
|
||||
{
|
||||
"cell_type": "markdown",
|
||||
"metadata": {},
|
||||
"source": [
|
||||
"Our examples use a GPT model as the LLM, and OpenAI offers an API for this purpose. You can obtain the key from the following link: https://platform.openai.com/account/api-keys .\n",
|
||||
"\n",
|
||||
"We will use the SerpApi to retrieve search results from Google. To acquire the SerpApi key, please go to https://serpapi.com/manage-api-key ."
|
||||
]
|
||||
},
|
||||
{
|
||||
"cell_type": "code",
|
||||
"execution_count": null,
|
||||
"metadata": {
|
||||
"id": "T1bSmKd6V2If"
|
||||
},
|
||||
"outputs": [],
|
||||
"source": [
|
||||
"os.environ[\"OPENAI_API_KEY\"] = \"...\"\n",
|
||||
"os.environ[\"SERPAPI_API_KEY\"] = \"...\""
|
||||
]
|
||||
},
|
||||
{
|
||||
"cell_type": "markdown",
|
||||
"metadata": {
|
||||
"id": "QenUYuBZjIzc"
|
||||
},
|
||||
"source": [
|
||||
"The event methods of `AimCallbackHandler` accept the LangChain module or agent as input and log at least the prompts and generated results, as well as the serialized version of the LangChain module, to the designated Aim run."
|
||||
]
|
||||
},
|
||||
{
|
||||
"cell_type": "code",
|
||||
"execution_count": null,
|
||||
"metadata": {
|
||||
"id": "KAz8weWuUeXF"
|
||||
},
|
||||
"outputs": [],
|
||||
"source": [
|
||||
"session_group = datetime.now().strftime(\"%m.%d.%Y_%H.%M.%S\")\n",
|
||||
"aim_callback = AimCallbackHandler(\n",
|
||||
" repo=\".\",\n",
|
||||
" experiment_name=\"scenario 1: OpenAI LLM\",\n",
|
||||
")\n",
|
||||
"\n",
|
||||
"manager = CallbackManager([StdOutCallbackHandler(), aim_callback])\n",
|
||||
"llm = OpenAI(temperature=0, callback_manager=manager, verbose=True)"
|
||||
]
|
||||
},
|
||||
{
|
||||
"cell_type": "markdown",
|
||||
"metadata": {
|
||||
"id": "b8WfByB4fl6N"
|
||||
},
|
||||
"source": [
|
||||
"The `flush_tracker` function is used to record LangChain assets on Aim. By default, the session is reset rather than being terminated outright."
|
||||
]
|
||||
},
|
||||
{
|
||||
"cell_type": "markdown",
|
||||
"metadata": {},
|
||||
"source": [
|
||||
"<h3>Scenario 1</h3> In the first scenario, we will use OpenAI LLM."
|
||||
]
|
||||
},
|
||||
{
|
||||
"cell_type": "code",
|
||||
"execution_count": null,
|
||||
"metadata": {
|
||||
"id": "o_VmneyIUyx8"
|
||||
},
|
||||
"outputs": [],
|
||||
"source": [
|
||||
"# scenario 1 - LLM\n",
|
||||
"llm_result = llm.generate([\"Tell me a joke\", \"Tell me a poem\"] * 3)\n",
|
||||
"aim_callback.flush_tracker(\n",
|
||||
" langchain_asset=llm,\n",
|
||||
" experiment_name=\"scenario 2: Chain with multiple SubChains on multiple generations\",\n",
|
||||
")\n"
|
||||
]
|
||||
},
|
||||
{
|
||||
"cell_type": "markdown",
|
||||
"metadata": {},
|
||||
"source": [
|
||||
"<h3>Scenario 2</h3> Scenario two involves chaining with multiple SubChains across multiple generations."
|
||||
]
|
||||
},
|
||||
{
|
||||
"cell_type": "code",
|
||||
"execution_count": null,
|
||||
"metadata": {
|
||||
"id": "trxslyb1U28Y"
|
||||
},
|
||||
"outputs": [],
|
||||
"source": [
|
||||
"from langchain.prompts import PromptTemplate\n",
|
||||
"from langchain.chains import LLMChain"
|
||||
]
|
||||
},
|
||||
{
|
||||
"cell_type": "code",
|
||||
"execution_count": null,
|
||||
"metadata": {
|
||||
"id": "uauQk10SUzF6"
|
||||
},
|
||||
"outputs": [],
|
||||
"source": [
|
||||
"# scenario 2 - Chain\n",
|
||||
"template = \"\"\"You are a playwright. Given the title of play, it is your job to write a synopsis for that title.\n",
|
||||
"Title: {title}\n",
|
||||
"Playwright: This is a synopsis for the above play:\"\"\"\n",
|
||||
"prompt_template = PromptTemplate(input_variables=[\"title\"], template=template)\n",
|
||||
"synopsis_chain = LLMChain(llm=llm, prompt=prompt_template, callback_manager=manager)\n",
|
||||
"\n",
|
||||
"test_prompts = [\n",
|
||||
" {\"title\": \"documentary about good video games that push the boundary of game design\"},\n",
|
||||
" {\"title\": \"the phenomenon behind the remarkable speed of cheetahs\"},\n",
|
||||
" {\"title\": \"the best in class mlops tooling\"},\n",
|
||||
"]\n",
|
||||
"synopsis_chain.apply(test_prompts)\n",
|
||||
"aim_callback.flush_tracker(\n",
|
||||
" langchain_asset=synopsis_chain, experiment_name=\"scenario 3: Agent with Tools\"\n",
|
||||
")"
|
||||
]
|
||||
},
|
||||
{
|
||||
"cell_type": "markdown",
|
||||
"metadata": {},
|
||||
"source": [
|
||||
"<h3>Scenario 3</h3> The third scenario involves an agent with tools."
|
||||
]
|
||||
},
|
||||
{
|
||||
"cell_type": "code",
|
||||
"execution_count": null,
|
||||
"metadata": {
|
||||
"id": "_jN73xcPVEpI"
|
||||
},
|
||||
"outputs": [],
|
||||
"source": [
|
||||
"from langchain.agents import initialize_agent, load_tools"
|
||||
]
|
||||
},
|
||||
{
|
||||
"cell_type": "code",
|
||||
"execution_count": null,
|
||||
"metadata": {
|
||||
"colab": {
|
||||
"base_uri": "https://localhost:8080/"
|
||||
},
|
||||
"id": "Gpq4rk6VT9cu",
|
||||
"outputId": "68ae261e-d0a2-4229-83c4-762562263b66"
|
||||
},
|
||||
"outputs": [
|
||||
{
|
||||
"name": "stdout",
|
||||
"output_type": "stream",
|
||||
"text": [
|
||||
"\n",
|
||||
"\n",
|
||||
"\u001b[1m> Entering new AgentExecutor chain...\u001b[0m\n",
|
||||
"\u001b[32;1m\u001b[1;3m I need to find out who Leo DiCaprio's girlfriend is and then calculate her age raised to the 0.43 power.\n",
|
||||
"Action: Search\n",
|
||||
"Action Input: \"Leo DiCaprio girlfriend\"\u001b[0m\n",
|
||||
"Observation: \u001b[36;1m\u001b[1;3mLeonardo DiCaprio seemed to prove a long-held theory about his love life right after splitting from girlfriend Camila Morrone just months ...\u001b[0m\n",
|
||||
"Thought:\u001b[32;1m\u001b[1;3m I need to find out Camila Morrone's age\n",
|
||||
"Action: Search\n",
|
||||
"Action Input: \"Camila Morrone age\"\u001b[0m\n",
|
||||
"Observation: \u001b[36;1m\u001b[1;3m25 years\u001b[0m\n",
|
||||
"Thought:\u001b[32;1m\u001b[1;3m I need to calculate 25 raised to the 0.43 power\n",
|
||||
"Action: Calculator\n",
|
||||
"Action Input: 25^0.43\u001b[0m\n",
|
||||
"Observation: \u001b[33;1m\u001b[1;3mAnswer: 3.991298452658078\n",
|
||||
"\u001b[0m\n",
|
||||
"Thought:\u001b[32;1m\u001b[1;3m I now know the final answer\n",
|
||||
"Final Answer: Camila Morrone is Leo DiCaprio's girlfriend and her current age raised to the 0.43 power is 3.991298452658078.\u001b[0m\n",
|
||||
"\n",
|
||||
"\u001b[1m> Finished chain.\u001b[0m\n"
|
||||
]
|
||||
}
|
||||
],
|
||||
"source": [
|
||||
"# scenario 3 - Agent with Tools\n",
|
||||
"tools = load_tools([\"serpapi\", \"llm-math\"], llm=llm, callback_manager=manager)\n",
|
||||
"agent = initialize_agent(\n",
|
||||
" tools,\n",
|
||||
" llm,\n",
|
||||
" agent=\"zero-shot-react-description\",\n",
|
||||
" callback_manager=manager,\n",
|
||||
" verbose=True,\n",
|
||||
")\n",
|
||||
"agent.run(\n",
|
||||
" \"Who is Leo DiCaprio's girlfriend? What is her current age raised to the 0.43 power?\"\n",
|
||||
")\n",
|
||||
"aim_callback.flush_tracker(langchain_asset=agent, reset=False, finish=True)"
|
||||
]
|
||||
}
|
||||
],
|
||||
"metadata": {
|
||||
"accelerator": "GPU",
|
||||
"colab": {
|
||||
"provenance": []
|
||||
},
|
||||
"gpuClass": "standard",
|
||||
"kernelspec": {
|
||||
"display_name": "Python 3 (ipykernel)",
|
||||
"language": "python",
|
||||
"name": "python3"
|
||||
},
|
||||
"language_info": {
|
||||
"codemirror_mode": {
|
||||
"name": "ipython",
|
||||
"version": 3
|
||||
},
|
||||
"file_extension": ".py",
|
||||
"mimetype": "text/x-python",
|
||||
"name": "python",
|
||||
"nbconvert_exporter": "python",
|
||||
"pygments_lexer": "ipython3",
|
||||
"version": "3.9.1"
|
||||
}
|
||||
},
|
||||
"nbformat": 4,
|
||||
"nbformat_minor": 1
|
||||
}
|
@ -0,0 +1,427 @@
|
||||
from copy import deepcopy
|
||||
from typing import Any, Dict, List, Optional, Union
|
||||
|
||||
from langchain.callbacks.base import BaseCallbackHandler
|
||||
from langchain.schema import AgentAction, AgentFinish, LLMResult
|
||||
|
||||
|
||||
def import_aim() -> Any:
|
||||
try:
|
||||
import aim
|
||||
except ImportError:
|
||||
raise ImportError(
|
||||
"To use the Aim callback manager you need to have the"
|
||||
" `aim` python package installed."
|
||||
"Please install it with `pip install aim`"
|
||||
)
|
||||
return aim
|
||||
|
||||
|
||||
class BaseMetadataCallbackHandler:
|
||||
"""This class handles the metadata and associated function states for callbacks.
|
||||
|
||||
Attributes:
|
||||
step (int): The current step.
|
||||
starts (int): The number of times the start method has been called.
|
||||
ends (int): The number of times the end method has been called.
|
||||
errors (int): The number of times the error method has been called.
|
||||
text_ctr (int): The number of times the text method has been called.
|
||||
ignore_llm_ (bool): Whether to ignore llm callbacks.
|
||||
ignore_chain_ (bool): Whether to ignore chain callbacks.
|
||||
ignore_agent_ (bool): Whether to ignore agent callbacks.
|
||||
always_verbose_ (bool): Whether to always be verbose.
|
||||
chain_starts (int): The number of times the chain start method has been called.
|
||||
chain_ends (int): The number of times the chain end method has been called.
|
||||
llm_starts (int): The number of times the llm start method has been called.
|
||||
llm_ends (int): The number of times the llm end method has been called.
|
||||
llm_streams (int): The number of times the text method has been called.
|
||||
tool_starts (int): The number of times the tool start method has been called.
|
||||
tool_ends (int): The number of times the tool end method has been called.
|
||||
agent_ends (int): The number of times the agent end method has been called.
|
||||
"""
|
||||
|
||||
def __init__(self) -> None:
|
||||
self.step = 0
|
||||
|
||||
self.starts = 0
|
||||
self.ends = 0
|
||||
self.errors = 0
|
||||
self.text_ctr = 0
|
||||
|
||||
self.ignore_llm_ = False
|
||||
self.ignore_chain_ = False
|
||||
self.ignore_agent_ = False
|
||||
self.always_verbose_ = False
|
||||
|
||||
self.chain_starts = 0
|
||||
self.chain_ends = 0
|
||||
|
||||
self.llm_starts = 0
|
||||
self.llm_ends = 0
|
||||
self.llm_streams = 0
|
||||
|
||||
self.tool_starts = 0
|
||||
self.tool_ends = 0
|
||||
|
||||
self.agent_ends = 0
|
||||
|
||||
@property
|
||||
def always_verbose(self) -> bool:
|
||||
"""Whether to call verbose callbacks even if verbose is False."""
|
||||
return self.always_verbose_
|
||||
|
||||
@property
|
||||
def ignore_llm(self) -> bool:
|
||||
"""Whether to ignore LLM callbacks."""
|
||||
return self.ignore_llm_
|
||||
|
||||
@property
|
||||
def ignore_chain(self) -> bool:
|
||||
"""Whether to ignore chain callbacks."""
|
||||
return self.ignore_chain_
|
||||
|
||||
@property
|
||||
def ignore_agent(self) -> bool:
|
||||
"""Whether to ignore agent callbacks."""
|
||||
return self.ignore_agent_
|
||||
|
||||
def get_custom_callback_meta(self) -> Dict[str, Any]:
|
||||
return {
|
||||
"step": self.step,
|
||||
"starts": self.starts,
|
||||
"ends": self.ends,
|
||||
"errors": self.errors,
|
||||
"text_ctr": self.text_ctr,
|
||||
"chain_starts": self.chain_starts,
|
||||
"chain_ends": self.chain_ends,
|
||||
"llm_starts": self.llm_starts,
|
||||
"llm_ends": self.llm_ends,
|
||||
"llm_streams": self.llm_streams,
|
||||
"tool_starts": self.tool_starts,
|
||||
"tool_ends": self.tool_ends,
|
||||
"agent_ends": self.agent_ends,
|
||||
}
|
||||
|
||||
def reset_callback_meta(self) -> None:
|
||||
"""Reset the callback metadata."""
|
||||
self.step = 0
|
||||
|
||||
self.starts = 0
|
||||
self.ends = 0
|
||||
self.errors = 0
|
||||
self.text_ctr = 0
|
||||
|
||||
self.ignore_llm_ = False
|
||||
self.ignore_chain_ = False
|
||||
self.ignore_agent_ = False
|
||||
self.always_verbose_ = False
|
||||
|
||||
self.chain_starts = 0
|
||||
self.chain_ends = 0
|
||||
|
||||
self.llm_starts = 0
|
||||
self.llm_ends = 0
|
||||
self.llm_streams = 0
|
||||
|
||||
self.tool_starts = 0
|
||||
self.tool_ends = 0
|
||||
|
||||
self.agent_ends = 0
|
||||
|
||||
return None
|
||||
|
||||
|
||||
class AimCallbackHandler(BaseMetadataCallbackHandler, BaseCallbackHandler):
|
||||
"""Callback Handler that logs to Aim.
|
||||
|
||||
Parameters:
|
||||
repo (:obj:`str`, optional): Aim repository path or Repo object to which
|
||||
Run object is bound. If skipped, default Repo is used.
|
||||
experiment_name (:obj:`str`, optional): Sets Run's `experiment` property.
|
||||
'default' if not specified. Can be used later to query runs/sequences.
|
||||
system_tracking_interval (:obj:`int`, optional): Sets the tracking interval
|
||||
in seconds for system usage metrics (CPU, Memory, etc.). Set to `None`
|
||||
to disable system metrics tracking.
|
||||
log_system_params (:obj:`bool`, optional): Enable/Disable logging of system
|
||||
params such as installed packages, git info, environment variables, etc.
|
||||
|
||||
This handler will utilize the associated callback method called and formats
|
||||
the input of each callback function with metadata regarding the state of LLM run
|
||||
and then logs the response to Aim.
|
||||
"""
|
||||
|
||||
def __init__(
|
||||
self,
|
||||
repo: Optional[str] = None,
|
||||
experiment_name: Optional[str] = None,
|
||||
system_tracking_interval: Optional[int] = 10,
|
||||
log_system_params: bool = True,
|
||||
) -> None:
|
||||
"""Initialize callback handler."""
|
||||
|
||||
super().__init__()
|
||||
|
||||
aim = import_aim()
|
||||
self.repo = repo
|
||||
self.experiment_name = experiment_name
|
||||
self.system_tracking_interval = system_tracking_interval
|
||||
self.log_system_params = log_system_params
|
||||
self._run = aim.Run(
|
||||
repo=self.repo,
|
||||
experiment=self.experiment_name,
|
||||
system_tracking_interval=self.system_tracking_interval,
|
||||
log_system_params=self.log_system_params,
|
||||
)
|
||||
self._run_hash = self._run.hash
|
||||
self.action_records: list = []
|
||||
|
||||
def setup(self, **kwargs: Any) -> None:
|
||||
aim = import_aim()
|
||||
|
||||
if not self._run:
|
||||
if self._run_hash:
|
||||
self._run = aim.Run(
|
||||
self._run_hash,
|
||||
repo=self.repo,
|
||||
system_tracking_interval=self.system_tracking_interval,
|
||||
)
|
||||
else:
|
||||
self._run = aim.Run(
|
||||
repo=self.repo,
|
||||
experiment=self.experiment_name,
|
||||
system_tracking_interval=self.system_tracking_interval,
|
||||
log_system_params=self.log_system_params,
|
||||
)
|
||||
self._run_hash = self._run.hash
|
||||
|
||||
if kwargs:
|
||||
for key, value in kwargs.items():
|
||||
self._run.set(key, value, strict=False)
|
||||
|
||||
def on_llm_start(
|
||||
self, serialized: Dict[str, Any], prompts: List[str], **kwargs: Any
|
||||
) -> None:
|
||||
"""Run when LLM starts."""
|
||||
aim = import_aim()
|
||||
|
||||
self.step += 1
|
||||
self.llm_starts += 1
|
||||
self.starts += 1
|
||||
|
||||
resp = {"action": "on_llm_start"}
|
||||
resp.update(self.get_custom_callback_meta())
|
||||
|
||||
prompts_res = deepcopy(prompts)
|
||||
|
||||
self._run.track(
|
||||
[aim.Text(prompt) for prompt in prompts_res],
|
||||
name="on_llm_start",
|
||||
context=resp,
|
||||
)
|
||||
|
||||
def on_llm_end(self, response: LLMResult, **kwargs: Any) -> None:
|
||||
"""Run when LLM ends running."""
|
||||
aim = import_aim()
|
||||
self.step += 1
|
||||
self.llm_ends += 1
|
||||
self.ends += 1
|
||||
|
||||
resp = {"action": "on_llm_end"}
|
||||
resp.update(self.get_custom_callback_meta())
|
||||
|
||||
response_res = deepcopy(response)
|
||||
|
||||
generated = [
|
||||
aim.Text(generation.text)
|
||||
for generations in response_res.generations
|
||||
for generation in generations
|
||||
]
|
||||
self._run.track(
|
||||
generated,
|
||||
name="on_llm_end",
|
||||
context=resp,
|
||||
)
|
||||
|
||||
def on_llm_new_token(self, token: str, **kwargs: Any) -> None:
|
||||
"""Run when LLM generates a new token."""
|
||||
self.step += 1
|
||||
self.llm_streams += 1
|
||||
|
||||
def on_llm_error(
|
||||
self, error: Union[Exception, KeyboardInterrupt], **kwargs: Any
|
||||
) -> None:
|
||||
"""Run when LLM errors."""
|
||||
self.step += 1
|
||||
self.errors += 1
|
||||
|
||||
def on_chain_start(
|
||||
self, serialized: Dict[str, Any], inputs: Dict[str, Any], **kwargs: Any
|
||||
) -> None:
|
||||
"""Run when chain starts running."""
|
||||
aim = import_aim()
|
||||
self.step += 1
|
||||
self.chain_starts += 1
|
||||
self.starts += 1
|
||||
|
||||
resp = {"action": "on_chain_start"}
|
||||
resp.update(self.get_custom_callback_meta())
|
||||
|
||||
inputs_res = deepcopy(inputs)
|
||||
|
||||
self._run.track(
|
||||
aim.Text(inputs_res["input"]), name="on_chain_start", context=resp
|
||||
)
|
||||
|
||||
def on_chain_end(self, outputs: Dict[str, Any], **kwargs: Any) -> None:
|
||||
"""Run when chain ends running."""
|
||||
aim = import_aim()
|
||||
self.step += 1
|
||||
self.chain_ends += 1
|
||||
self.ends += 1
|
||||
|
||||
resp = {"action": "on_chain_end"}
|
||||
resp.update(self.get_custom_callback_meta())
|
||||
|
||||
outputs_res = deepcopy(outputs)
|
||||
|
||||
self._run.track(
|
||||
aim.Text(outputs_res["output"]), name="on_chain_end", context=resp
|
||||
)
|
||||
|
||||
def on_chain_error(
|
||||
self, error: Union[Exception, KeyboardInterrupt], **kwargs: Any
|
||||
) -> None:
|
||||
"""Run when chain errors."""
|
||||
self.step += 1
|
||||
self.errors += 1
|
||||
|
||||
def on_tool_start(
|
||||
self, serialized: Dict[str, Any], input_str: str, **kwargs: Any
|
||||
) -> None:
|
||||
"""Run when tool starts running."""
|
||||
aim = import_aim()
|
||||
self.step += 1
|
||||
self.tool_starts += 1
|
||||
self.starts += 1
|
||||
|
||||
resp = {"action": "on_tool_start"}
|
||||
resp.update(self.get_custom_callback_meta())
|
||||
|
||||
self._run.track(aim.Text(input_str), name="on_tool_start", context=resp)
|
||||
|
||||
def on_tool_end(self, output: str, **kwargs: Any) -> None:
|
||||
"""Run when tool ends running."""
|
||||
aim = import_aim()
|
||||
self.step += 1
|
||||
self.tool_ends += 1
|
||||
self.ends += 1
|
||||
|
||||
resp = {"action": "on_tool_end"}
|
||||
resp.update(self.get_custom_callback_meta())
|
||||
|
||||
self._run.track(aim.Text(output), name="on_tool_end", context=resp)
|
||||
|
||||
def on_tool_error(
|
||||
self, error: Union[Exception, KeyboardInterrupt], **kwargs: Any
|
||||
) -> None:
|
||||
"""Run when tool errors."""
|
||||
self.step += 1
|
||||
self.errors += 1
|
||||
|
||||
def on_text(self, text: str, **kwargs: Any) -> None:
|
||||
"""
|
||||
Run when agent is ending.
|
||||
"""
|
||||
self.step += 1
|
||||
self.text_ctr += 1
|
||||
|
||||
def on_agent_finish(self, finish: AgentFinish, **kwargs: Any) -> None:
|
||||
"""Run when agent ends running."""
|
||||
aim = import_aim()
|
||||
self.step += 1
|
||||
self.agent_ends += 1
|
||||
self.ends += 1
|
||||
|
||||
resp = {"action": "on_agent_finish"}
|
||||
resp.update(self.get_custom_callback_meta())
|
||||
|
||||
finish_res = deepcopy(finish)
|
||||
|
||||
text = "OUTPUT:\n{}\n\nLOG:\n{}".format(
|
||||
finish_res.return_values["output"], finish_res.log
|
||||
)
|
||||
self._run.track(aim.Text(text), name="on_agent_finish", context=resp)
|
||||
|
||||
def on_agent_action(self, action: AgentAction, **kwargs: Any) -> Any:
|
||||
"""Run on agent action."""
|
||||
aim = import_aim()
|
||||
self.step += 1
|
||||
self.tool_starts += 1
|
||||
self.starts += 1
|
||||
|
||||
resp = {
|
||||
"action": "on_agent_action",
|
||||
"tool": action.tool,
|
||||
}
|
||||
resp.update(self.get_custom_callback_meta())
|
||||
|
||||
action_res = deepcopy(action)
|
||||
|
||||
text = "TOOL INPUT:\n{}\n\nLOG:\n{}".format(
|
||||
action_res.tool_input, action_res.log
|
||||
)
|
||||
self._run.track(aim.Text(text), name="on_agent_action", context=resp)
|
||||
|
||||
def flush_tracker(
|
||||
self,
|
||||
repo: Optional[str] = None,
|
||||
experiment_name: Optional[str] = None,
|
||||
system_tracking_interval: Optional[int] = 10,
|
||||
log_system_params: bool = True,
|
||||
langchain_asset: Any = None,
|
||||
reset: bool = True,
|
||||
finish: bool = False,
|
||||
) -> None:
|
||||
"""Flush the tracker and reset the session.
|
||||
|
||||
Args:
|
||||
repo (:obj:`str`, optional): Aim repository path or Repo object to which
|
||||
Run object is bound. If skipped, default Repo is used.
|
||||
experiment_name (:obj:`str`, optional): Sets Run's `experiment` property.
|
||||
'default' if not specified. Can be used later to query runs/sequences.
|
||||
system_tracking_interval (:obj:`int`, optional): Sets the tracking interval
|
||||
in seconds for system usage metrics (CPU, Memory, etc.). Set to `None`
|
||||
to disable system metrics tracking.
|
||||
log_system_params (:obj:`bool`, optional): Enable/Disable logging of system
|
||||
params such as installed packages, git info, environment variables, etc.
|
||||
langchain_asset: The langchain asset to save.
|
||||
reset: Whether to reset the session.
|
||||
finish: Whether to finish the run.
|
||||
|
||||
Returns:
|
||||
None
|
||||
"""
|
||||
|
||||
if langchain_asset:
|
||||
try:
|
||||
for key, value in langchain_asset.dict().items():
|
||||
self._run.set(key, value, strict=False)
|
||||
except Exception:
|
||||
pass
|
||||
|
||||
if finish or reset:
|
||||
self._run.close()
|
||||
self.reset_callback_meta()
|
||||
if reset:
|
||||
self.__init__( # type: ignore
|
||||
repo=repo if repo else self.repo,
|
||||
experiment_name=experiment_name
|
||||
if experiment_name
|
||||
else self.experiment_name,
|
||||
system_tracking_interval=system_tracking_interval
|
||||
if system_tracking_interval
|
||||
else self.system_tracking_interval,
|
||||
log_system_params=log_system_params
|
||||
if log_system_params
|
||||
else self.log_system_params,
|
||||
)
|
Loading…
Reference in New Issue