forked from Archives/langchain
ElasticVectorSearch: Add in vector search backed by Elastic (#67)
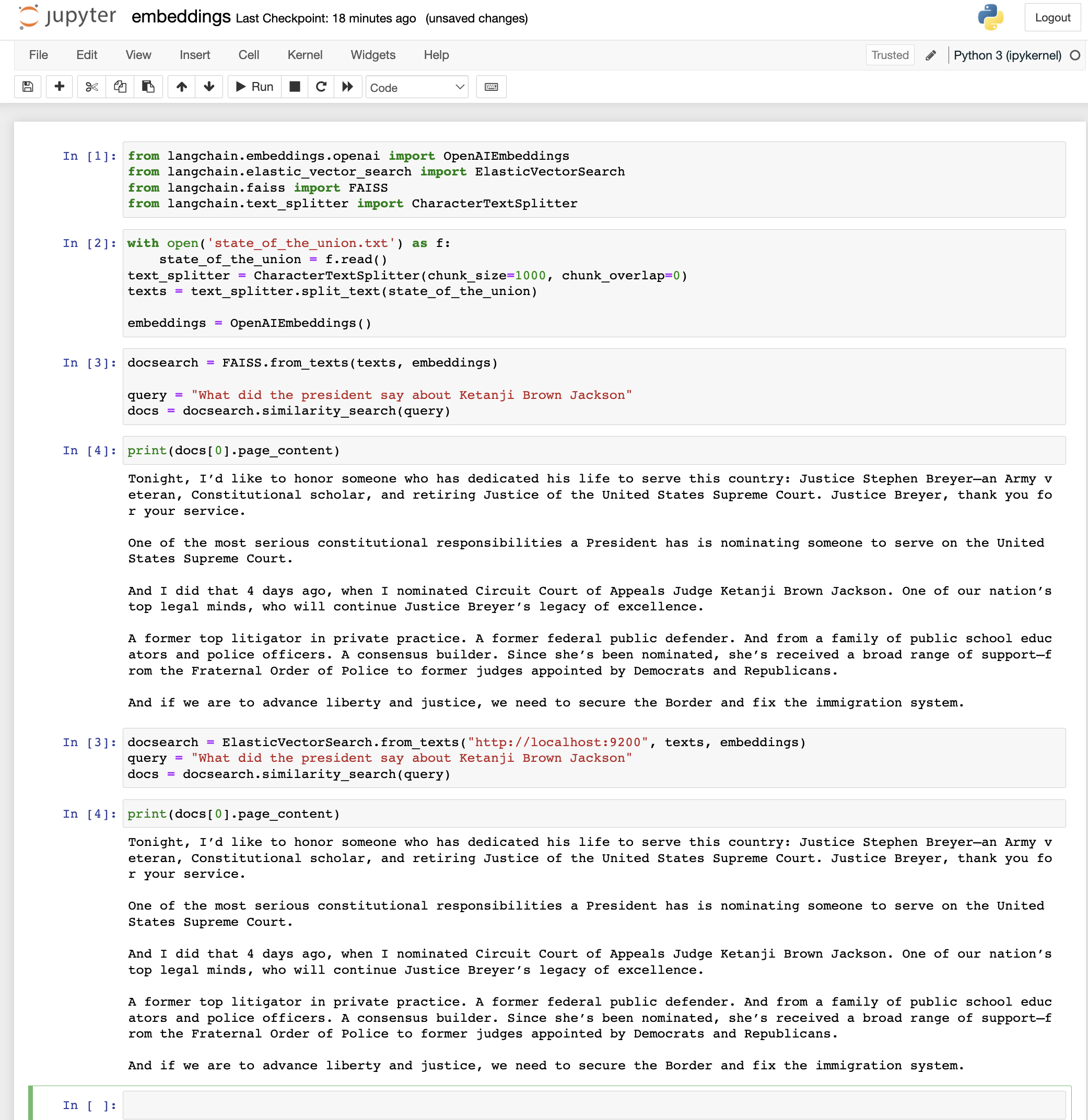 woo! Co-authored-by: Harrison Chase <hw.chase.17@gmail.com>harrison/prompt_examples
parent
efbc03bda8
commit
e48e562ea5
@ -0,0 +1,146 @@
|
||||
"""Wrapper around Elasticsearch vector database."""
|
||||
import uuid
|
||||
from typing import Callable, Dict, List
|
||||
|
||||
from langchain.docstore.document import Document
|
||||
from langchain.embeddings.base import Embeddings
|
||||
|
||||
|
||||
def _default_text_mapping(dim: int) -> Dict:
|
||||
return {
|
||||
"properties": {
|
||||
"text": {"type": "text"},
|
||||
"vector": {"type": "dense_vector", "dims": dim},
|
||||
}
|
||||
}
|
||||
|
||||
|
||||
def _default_script_query(query_vector: List[int]) -> Dict:
|
||||
return {
|
||||
"script_score": {
|
||||
"query": {"match_all": {}},
|
||||
"script": {
|
||||
"source": "cosineSimilarity(params.query_vector, 'vector') + 1.0",
|
||||
"params": {"query_vector": query_vector},
|
||||
},
|
||||
}
|
||||
}
|
||||
|
||||
|
||||
class ElasticVectorSearch:
|
||||
"""Wrapper around Elasticsearch as a vector database.
|
||||
|
||||
Example:
|
||||
.. code-block:: python
|
||||
|
||||
from langchain import ElasticVectorSearch
|
||||
elastic_vector_search = ElasticVectorSearch(
|
||||
"http://localhost:9200",
|
||||
"embeddings",
|
||||
mapping,
|
||||
embedding_function
|
||||
)
|
||||
|
||||
"""
|
||||
|
||||
def __init__(
|
||||
self,
|
||||
elastic_url: str,
|
||||
index_name: str,
|
||||
mapping: Dict,
|
||||
embedding_function: Callable,
|
||||
):
|
||||
"""Initialize with necessary components."""
|
||||
try:
|
||||
import elasticsearch
|
||||
except ImportError:
|
||||
raise ValueError(
|
||||
"Could not import elasticsearch python packge. "
|
||||
"Please install it with `pip install elasticearch`."
|
||||
)
|
||||
self.embedding_function = embedding_function
|
||||
self.index_name = index_name
|
||||
try:
|
||||
es_client = elasticsearch.Elasticsearch(elastic_url) # noqa
|
||||
except ValueError as e:
|
||||
raise ValueError(
|
||||
"Your elasticsearch client string is misformatted. " f"Got error: {e} "
|
||||
)
|
||||
self.client = es_client
|
||||
self.mapping = mapping
|
||||
|
||||
def similarity_search(self, query: str, k: int = 4) -> List[Document]:
|
||||
"""Return docs most similar to query.
|
||||
|
||||
Args:
|
||||
query: Text to look up documents similar to.
|
||||
k: Number of Documents to return. Defaults to 4.
|
||||
|
||||
Returns:
|
||||
List of Documents most similar to the query.
|
||||
"""
|
||||
embedding = self.embedding_function(query)
|
||||
script_query = _default_script_query(embedding)
|
||||
response = self.client.search(index=self.index_name, query=script_query)
|
||||
texts = [hit["_source"]["text"] for hit in response["hits"]["hits"][:k]]
|
||||
documents = [Document(page_content=text) for text in texts]
|
||||
return documents
|
||||
|
||||
@classmethod
|
||||
def from_texts(
|
||||
cls, elastic_url: str, texts: List[str], embedding: Embeddings
|
||||
) -> "ElasticVectorSearch":
|
||||
"""Construct ElasticVectorSearch wrapper from raw documents.
|
||||
|
||||
This is a user friendly interface that:
|
||||
1. Embeds documents.
|
||||
2. Creates a new index for the embeddings in the Elasticsearch instance.
|
||||
3. Adds the documents to the newly created Elasticsearch index.
|
||||
|
||||
This is intended to be a quick way to get started.
|
||||
|
||||
Example:
|
||||
.. code-block:: python
|
||||
|
||||
from langchain import ElasticVectorSearch
|
||||
from langchain.embeddings import OpenAIEmbeddings
|
||||
embeddings = OpenAIEmbeddings()
|
||||
elastic_vector_search = ElasticVectorSearch.from_texts(
|
||||
"http://localhost:9200",
|
||||
texts,
|
||||
embeddings
|
||||
)
|
||||
"""
|
||||
try:
|
||||
import elasticsearch
|
||||
from elasticsearch.helpers import bulk
|
||||
except ImportError:
|
||||
raise ValueError(
|
||||
"Could not import elasticsearch python packge. "
|
||||
"Please install it with `pip install elasticearch`."
|
||||
)
|
||||
try:
|
||||
client = elasticsearch.Elasticsearch(elastic_url)
|
||||
except ValueError as e:
|
||||
raise ValueError(
|
||||
"Your elasticsearch client string is misformatted. " f"Got error: {e} "
|
||||
)
|
||||
index_name = uuid.uuid4().hex
|
||||
embeddings = embedding.embed_documents(texts)
|
||||
dim = len(embeddings[0])
|
||||
mapping = _default_text_mapping(dim)
|
||||
# TODO would be nice to create index before embedding,
|
||||
# just to save expensive steps for last
|
||||
client.indices.create(index=index_name, mappings=mapping)
|
||||
requests = []
|
||||
for i, text in enumerate(texts):
|
||||
request = {
|
||||
"_op_type": "index",
|
||||
"_index": index_name,
|
||||
"vector": embeddings[i],
|
||||
"text": text,
|
||||
}
|
||||
requests.append(request)
|
||||
bulk(client, requests)
|
||||
client.indices.refresh(index=index_name)
|
||||
return cls(elastic_url, index_name, mapping, embedding.embed_query)
|
Loading…
Reference in New Issue