Accepted incoming changes to resolve merges
commit
2f711b2ace
File diff suppressed because it is too large
Load Diff
File diff suppressed because one or more lines are too long
File diff suppressed because one or more lines are too long
File diff suppressed because it is too large
Load Diff
@ -0,0 +1,601 @@
|
||||
{
|
||||
"cells": [
|
||||
{
|
||||
"cell_type": "markdown",
|
||||
"metadata": {},
|
||||
"source": [
|
||||
"## Named Entity Recognition (NER) to Enrich Text"
|
||||
]
|
||||
},
|
||||
{
|
||||
"cell_type": "markdown",
|
||||
"metadata": {},
|
||||
"source": [
|
||||
"`Named Entity Recognition` (NER) is a `Natural Language Processing` task that identifies and classifies named entities (NE) into predefined semantic categories (such as persons, organizations, locations, events, time expressions, and quantities). By converting raw text into structured information, NER makes data more actionable, facilitating tasks like information extraction, data aggregation, analytics, and social media monitoring.\n",
|
||||
"\n",
|
||||
"This notebook demonstrates how to carry out NER with [chat completion](https://platform.openai.com/docs/api-reference/chat) and [functions-calling](https://platform.openai.com/docs/guides/gpt/function-calling) to enrich a text with links to a knowledge base such as Wikipedia:\n",
|
||||
"\n",
|
||||
"**Text:**\n",
|
||||
"\n",
|
||||
"*In Germany, in 1440, goldsmith Johannes Gutenberg invented the movable-type printing press. His work led to an information revolution and the unprecedented mass-spread of literature throughout Europe. Modelled on the design of the existing screw presses, a single Renaissance movable-type printing press could produce up to 3,600 pages per workday.*\n",
|
||||
"\n",
|
||||
"**Text enriched with Wikipedia links:**\n",
|
||||
"\n",
|
||||
"*In [Germany](https://en.wikipedia.org/wiki/Germany), in 1440, goldsmith [Johannes Gutenberg]() invented the [movable-type printing press](https://en.wikipedia.org/wiki/Movable_Type). His work led to an [information revolution](https://en.wikipedia.org/wiki/Information_revolution) and the unprecedented mass-spread of literature throughout [Europe](https://en.wikipedia.org/wiki/Europe). Modelled on the design of the existing screw presses, a single [Renaissance](https://en.wikipedia.org/wiki/Renaissance) [movable-type printing press](https://en.wikipedia.org/wiki/Movable_Type) could produce up to 3,600 pages per workday.*\n",
|
||||
"\n",
|
||||
"**Inference Costs:** The notebook also illustrates how to estimate OpenAI API costs."
|
||||
]
|
||||
},
|
||||
{
|
||||
"cell_type": "markdown",
|
||||
"metadata": {},
|
||||
"source": [
|
||||
"### 1. Setup"
|
||||
]
|
||||
},
|
||||
{
|
||||
"cell_type": "markdown",
|
||||
"metadata": {},
|
||||
"source": [
|
||||
"#### 1.1 Install/Upgrade Python packages"
|
||||
]
|
||||
},
|
||||
{
|
||||
"cell_type": "code",
|
||||
"execution_count": 1,
|
||||
"metadata": {},
|
||||
"outputs": [
|
||||
{
|
||||
"name": "stdout",
|
||||
"output_type": "stream",
|
||||
"text": [
|
||||
"Note: you may need to restart the kernel to use updated packages.\n",
|
||||
"Note: you may need to restart the kernel to use updated packages.\n",
|
||||
"Note: you may need to restart the kernel to use updated packages.\n"
|
||||
]
|
||||
}
|
||||
],
|
||||
"source": [
|
||||
"%pip install --upgrade openai --quiet\n",
|
||||
"%pip install --upgrade nlpia2-wikipedia --quiet\n",
|
||||
"%pip install --upgrade tenacity --quiet"
|
||||
]
|
||||
},
|
||||
{
|
||||
"cell_type": "markdown",
|
||||
"metadata": {},
|
||||
"source": [
|
||||
"#### 1.2 Load packages and OPENAI_API_KEY"
|
||||
]
|
||||
},
|
||||
{
|
||||
"cell_type": "markdown",
|
||||
"metadata": {},
|
||||
"source": [
|
||||
"You can generate an API key in the OpenAI web interface. See https://platform.openai.com/account/api-keys for details."
|
||||
]
|
||||
},
|
||||
{
|
||||
"cell_type": "markdown",
|
||||
"metadata": {},
|
||||
"source": [
|
||||
"This notebook works with the latest OpeanAI models `gpt-3.5-turbo-0613` and `gpt-4-0613`."
|
||||
]
|
||||
},
|
||||
{
|
||||
"cell_type": "code",
|
||||
"execution_count": 2,
|
||||
"metadata": {},
|
||||
"outputs": [],
|
||||
"source": [
|
||||
"import json\n",
|
||||
"import logging\n",
|
||||
"import os\n",
|
||||
"\n",
|
||||
"import openai\n",
|
||||
"import wikipedia\n",
|
||||
"\n",
|
||||
"from typing import Optional\n",
|
||||
"from IPython.display import display, Markdown\n",
|
||||
"from tenacity import retry, wait_random_exponential, stop_after_attempt\n",
|
||||
"\n",
|
||||
"logging.basicConfig(level=logging.INFO, format=' %(asctime)s - %(levelname)s - %(message)s')\n",
|
||||
"\n",
|
||||
"OPENAI_MODEL = 'gpt-3.5-turbo-0613'\n",
|
||||
"openai.api_key = os.getenv(\"OPENAI_API_KEY\")"
|
||||
]
|
||||
},
|
||||
{
|
||||
"cell_type": "markdown",
|
||||
"metadata": {},
|
||||
"source": [
|
||||
"### 2. Define the NER labels to be Identified"
|
||||
]
|
||||
},
|
||||
{
|
||||
"cell_type": "markdown",
|
||||
"metadata": {},
|
||||
"source": [
|
||||
"We define a standard set of NER labels to showcase a wide range of use cases. However, for our specific task of enriching text with knowledge base links, only a subset is practically required."
|
||||
]
|
||||
},
|
||||
{
|
||||
"cell_type": "code",
|
||||
"execution_count": 3,
|
||||
"metadata": {},
|
||||
"outputs": [],
|
||||
"source": [
|
||||
"labels = [\n",
|
||||
" \"person\", # people, including fictional characters\n",
|
||||
" \"fac\", # buildings, airports, highways, bridges\n",
|
||||
" \"org\", # organizations, companies, agencies, institutions\n",
|
||||
" \"gpe\", # geopolitical entities like countries, cities, states\n",
|
||||
" \"loc\", # non-gpe locations\n",
|
||||
" \"product\", # vehicles, foods, appareal, appliances, software, toys \n",
|
||||
" \"event\", # named sports, scientific milestones, historical events\n",
|
||||
" \"work_of_art\", # titles of books, songs, movies\n",
|
||||
" \"law\", # named laws, acts, or legislations\n",
|
||||
" \"language\", # any named language\n",
|
||||
" \"date\", # absolute or relative dates or periods\n",
|
||||
" \"time\", # time units smaller than a day\n",
|
||||
" \"percent\", # percentage (e.g., \"twenty percent\", \"18%\")\n",
|
||||
" \"money\", # monetary values, including unit\n",
|
||||
" \"quantity\", # measurements, e.g., weight or distance\n",
|
||||
"]"
|
||||
]
|
||||
},
|
||||
{
|
||||
"cell_type": "markdown",
|
||||
"metadata": {},
|
||||
"source": [
|
||||
"### 3. Prepare messages"
|
||||
]
|
||||
},
|
||||
{
|
||||
"cell_type": "markdown",
|
||||
"metadata": {},
|
||||
"source": [
|
||||
"The [chat completions API](https://platform.openai.com/docs/guides/gpt/chat-completions-api) takes a list of messages as input and delivers a model-generated message as an output. While the chat format is primarily designed for facilitating multi-turn conversations, it is equally efficient for single-turn tasks without any preceding conversation. For our purposes, we will specify a message for the system, assistant, and user roles."
|
||||
]
|
||||
},
|
||||
{
|
||||
"cell_type": "markdown",
|
||||
"metadata": {},
|
||||
"source": [
|
||||
"#### 3.1 System Message"
|
||||
]
|
||||
},
|
||||
{
|
||||
"cell_type": "markdown",
|
||||
"metadata": {},
|
||||
"source": [
|
||||
"The `system message` (prompt) sets the assistant's behavior by defining its desired persona and task. We also delineate the specific set of entity labels we aim to identify."
|
||||
]
|
||||
},
|
||||
{
|
||||
"cell_type": "markdown",
|
||||
"metadata": {},
|
||||
"source": [
|
||||
"Although one can instruct the model to format its response, it has to be noted that both `gpt-3.5-turbo-0613` and `gpt-4-0613` have been fine-tuned to discern when a function should be invoked, and to reply with `JSON` formatted according to the function's signature. This capability streamlines our prompt and enables us to receive structured data directly from the model."
|
||||
]
|
||||
},
|
||||
{
|
||||
"cell_type": "code",
|
||||
"execution_count": 4,
|
||||
"metadata": {},
|
||||
"outputs": [],
|
||||
"source": [
|
||||
"def system_message(labels):\n",
|
||||
" return f\"\"\"\n",
|
||||
"You are an expert in Natural Language Processing. Your task is to identify common Named Entities (NER) in a given text.\n",
|
||||
"The possible common Named Entities (NER) types are exclusively: ({\", \".join(labels)}).\"\"\""
|
||||
]
|
||||
},
|
||||
{
|
||||
"cell_type": "markdown",
|
||||
"metadata": {},
|
||||
"source": [
|
||||
"#### 3.2 Assistant Message"
|
||||
]
|
||||
},
|
||||
{
|
||||
"cell_type": "markdown",
|
||||
"metadata": {},
|
||||
"source": [
|
||||
"`Assistant messages` usually store previous assistant responses. However, as in our scenario, they can also be crafted to provide examples of the desired behavior. While OpenAI is able to execute `zero-shot` Named Entity Recognition, we have found that a `one-shot` approach produces more precise results."
|
||||
]
|
||||
},
|
||||
{
|
||||
"cell_type": "code",
|
||||
"execution_count": 5,
|
||||
"metadata": {},
|
||||
"outputs": [],
|
||||
"source": [
|
||||
"def assisstant_message():\n",
|
||||
" return f\"\"\"\n",
|
||||
"EXAMPLE:\n",
|
||||
" Text: 'In Germany, in 1440, goldsmith Johannes Gutenberg invented the movable-type printing press. His work led to an information revolution and the unprecedented mass-spread / \n",
|
||||
" of literature throughout Europe. Modelled on the design of the existing screw presses, a single Renaissance movable-type printing press could produce up to 3,600 pages per workday.'\n",
|
||||
" {{\n",
|
||||
" \"gpe\": [\"Germany\", \"Europe\"],\n",
|
||||
" \"date\": [\"1440\"],\n",
|
||||
" \"person\": [\"Johannes Gutenberg\"],\n",
|
||||
" \"product\": [\"movable-type printing press\"],\n",
|
||||
" \"event\": [\"Renaissance\"],\n",
|
||||
" \"quantity\": [\"3,600 pages\"],\n",
|
||||
" \"time\": [\"workday\"]\n",
|
||||
" }}\n",
|
||||
"--\"\"\""
|
||||
]
|
||||
},
|
||||
{
|
||||
"cell_type": "markdown",
|
||||
"metadata": {},
|
||||
"source": [
|
||||
"#### 3.3 User Message"
|
||||
]
|
||||
},
|
||||
{
|
||||
"cell_type": "markdown",
|
||||
"metadata": {},
|
||||
"source": [
|
||||
"The `user message` provides the specific text for the assistant task:"
|
||||
]
|
||||
},
|
||||
{
|
||||
"cell_type": "code",
|
||||
"execution_count": 6,
|
||||
"metadata": {},
|
||||
"outputs": [],
|
||||
"source": [
|
||||
"def user_message(text):\n",
|
||||
" return f\"\"\"\n",
|
||||
"TASK:\n",
|
||||
" Text: {text}\n",
|
||||
"\"\"\""
|
||||
]
|
||||
},
|
||||
{
|
||||
"cell_type": "markdown",
|
||||
"metadata": {},
|
||||
"source": [
|
||||
"### 4. OpenAI Functions (and Utils)"
|
||||
]
|
||||
},
|
||||
{
|
||||
"cell_type": "markdown",
|
||||
"metadata": {},
|
||||
"source": [
|
||||
"In an OpenAI API call, we can describe `functions` to `gpt-3.5-turbo-0613` and `gpt-4-0613` and have the model intelligently choose to output a `JSON` object containing arguments to call those `functions`. It's important to note that the [chat completions API](https://platform.openai.com/docs/guides/gpt/chat-completions-api) doesn't actually execute the `function`. Instead, it provides the `JSON` output, which can then be used to call the `function` in our code. For more details, refer to the [OpenAI Function Calling Guide](https://platform.openai.com/docs/guides/gpt/function-calling)."
|
||||
]
|
||||
},
|
||||
{
|
||||
"cell_type": "markdown",
|
||||
"metadata": {},
|
||||
"source": [
|
||||
"Our function, `enrich_entities(text, label_entities` gets a block of text and a dictionary containing identified labels and entities as parameters. It then associates the recognized entities with their corresponding links to the Wikipedia articles."
|
||||
]
|
||||
},
|
||||
{
|
||||
"cell_type": "code",
|
||||
"execution_count": 7,
|
||||
"metadata": {},
|
||||
"outputs": [],
|
||||
"source": [
|
||||
"@retry(wait=wait_random_exponential(min=1, max=10), stop=stop_after_attempt(5))\n",
|
||||
"def find_link(entity: str) -> Optional[str]:\n",
|
||||
" \"\"\"\n",
|
||||
" Finds a Wikipedia link for a given entity.\n",
|
||||
" \"\"\"\n",
|
||||
" try:\n",
|
||||
" titles = wikipedia.search(entity)\n",
|
||||
" if titles:\n",
|
||||
" # naively consider the first result as the best\n",
|
||||
" page = wikipedia.page(titles[0])\n",
|
||||
" return page.url\n",
|
||||
" except (wikipedia.exceptions.WikipediaException) as ex:\n",
|
||||
" logging.error(f'Error occurred while searching for Wikipedia link for entity {entity}: {str(ex)}')\n",
|
||||
"\n",
|
||||
" return None"
|
||||
]
|
||||
},
|
||||
{
|
||||
"cell_type": "code",
|
||||
"execution_count": 8,
|
||||
"metadata": {},
|
||||
"outputs": [],
|
||||
"source": [
|
||||
"def find_all_links(label_entities:dict) -> dict:\n",
|
||||
" \"\"\" \n",
|
||||
" Finds all Wikipedia links for the dictionary entities in the whitelist label list.\n",
|
||||
" \"\"\"\n",
|
||||
" whitelist = ['event', 'gpe', 'org', 'person', 'product', 'work_of_art']\n",
|
||||
" \n",
|
||||
" return {e: find_link(e) for label, entities in label_entities.items() \n",
|
||||
" for e in entities\n",
|
||||
" if label in whitelist}"
|
||||
]
|
||||
},
|
||||
{
|
||||
"cell_type": "code",
|
||||
"execution_count": 9,
|
||||
"metadata": {},
|
||||
"outputs": [],
|
||||
"source": [
|
||||
"def enrich_entities(text: str, label_entities: dict) -> str:\n",
|
||||
" \"\"\"\n",
|
||||
" Enriches text with knowledge base links.\n",
|
||||
" \"\"\"\n",
|
||||
" entity_link_dict = find_all_links(label_entities)\n",
|
||||
" logging.info(f\"entity_link_dict: {entity_link_dict}\")\n",
|
||||
" \n",
|
||||
" for entity, link in entity_link_dict.items():\n",
|
||||
" text = text.replace(entity, f\"[{entity}]({link})\")\n",
|
||||
"\n",
|
||||
" return text"
|
||||
]
|
||||
},
|
||||
{
|
||||
"cell_type": "markdown",
|
||||
"metadata": {},
|
||||
"source": [
|
||||
"### 4. ChatCompletion"
|
||||
]
|
||||
},
|
||||
{
|
||||
"cell_type": "markdown",
|
||||
"metadata": {},
|
||||
"source": [
|
||||
"As previously highlighted, `gpt-3.5-turbo-0613` and `gpt-4-0613` have been fine-tuned to detect when a `function` should to be called. Moreover, they can produce a `JSON` response that conforms to the `function` signature. Here's the sequence we follow:\n",
|
||||
"\n",
|
||||
"1. Define our `function` and its associated `JSON` Schema.\n",
|
||||
"2. Invoke the model using the `messages`, `functions` and `function_call` parameters.\n",
|
||||
"3. Convert the output into a `JSON` object, and then call the `function` with the `arguments` provided by the model.\n",
|
||||
"\n",
|
||||
"In practice, one might want to re-invoke the model again by appending the `function` response as a new message, and let the model summarize the results back to the user. Nevertheless, for our purposes, this step is not needed.\n",
|
||||
"\n",
|
||||
"*Note that in a real-case scenario it is strongly recommended to build in user confirmation flows before taking actions.*"
|
||||
]
|
||||
},
|
||||
{
|
||||
"cell_type": "markdown",
|
||||
"metadata": {},
|
||||
"source": [
|
||||
"#### 4.1 Define our Function and JSON schema"
|
||||
]
|
||||
},
|
||||
{
|
||||
"cell_type": "markdown",
|
||||
"metadata": {},
|
||||
"source": [
|
||||
"Since we want the model to output a dictionary of labels and recognized entities:\n",
|
||||
"\n",
|
||||
"```python\n",
|
||||
"{ \n",
|
||||
" \"gpe\": [\"Germany\", \"Europe\"], \n",
|
||||
" \"date\": [\"1440\"], \n",
|
||||
" \"person\": [\"Johannes Gutenberg\"], \n",
|
||||
" \"product\": [\"movable-type printing press\"], \n",
|
||||
" \"event\": [\"Renaissance\"], \n",
|
||||
" \"quantity\": [\"3,600 pages\"], \n",
|
||||
" \"time\": [\"workday\"] \n",
|
||||
"} \n",
|
||||
"```\n",
|
||||
"we need to define the corresponding `JSON` schema to be passed to the `functions` parameter: "
|
||||
]
|
||||
},
|
||||
{
|
||||
"cell_type": "code",
|
||||
"execution_count": 10,
|
||||
"metadata": {},
|
||||
"outputs": [],
|
||||
"source": [
|
||||
"def generate_functions(labels: dict) -> list:\n",
|
||||
" return [\n",
|
||||
" {\n",
|
||||
" \"name\": \"enrich_entities\",\n",
|
||||
" \"description\": \"Enrich Text with Knowledge Base Links\",\n",
|
||||
" \"parameters\": {\n",
|
||||
" \"type\": \"object\",\n",
|
||||
" \"properties\": {\n",
|
||||
" \"r'^(?:' + '|'.join({labels}) + ')$'\": \n",
|
||||
" {\n",
|
||||
" \"type\": \"array\",\n",
|
||||
" \"items\": {\n",
|
||||
" \"type\": \"string\"\n",
|
||||
" }\n",
|
||||
" }\n",
|
||||
" },\n",
|
||||
" \"additionalProperties\": False\n",
|
||||
" },\n",
|
||||
" }\n",
|
||||
" ]"
|
||||
]
|
||||
},
|
||||
{
|
||||
"cell_type": "markdown",
|
||||
"metadata": {},
|
||||
"source": [
|
||||
"#### 4.2 Chat Completion"
|
||||
]
|
||||
},
|
||||
{
|
||||
"cell_type": "markdown",
|
||||
"metadata": {},
|
||||
"source": [
|
||||
"Now, we invoke the model. It's important to note that we direct the API to use a specific function by setting the `function_call` parameter to `{\"name\": \"enrich_entities\"}`."
|
||||
]
|
||||
},
|
||||
{
|
||||
"cell_type": "code",
|
||||
"execution_count": 11,
|
||||
"metadata": {},
|
||||
"outputs": [],
|
||||
"source": [
|
||||
"@retry(wait=wait_random_exponential(min=1, max=10), stop=stop_after_attempt(5))\n",
|
||||
"def run_openai_task(labels, text):\n",
|
||||
" messages = [\n",
|
||||
" {\"role\": \"system\", \"content\": system_message(labels=labels)},\n",
|
||||
" {\"role\": \"assistant\", \"content\": assisstant_message()},\n",
|
||||
" {\"role\": \"user\", \"content\": user_message(text=text)}\n",
|
||||
" ]\n",
|
||||
"\n",
|
||||
" response = openai.ChatCompletion.create(\n",
|
||||
" model=\"gpt-3.5-turbo-0613\",\n",
|
||||
" messages=messages,\n",
|
||||
" functions=generate_functions(labels),\n",
|
||||
" function_call={\"name\": \"enrich_entities\"}, \n",
|
||||
" temperature=0,\n",
|
||||
" frequency_penalty=0,\n",
|
||||
" presence_penalty=0,\n",
|
||||
" )\n",
|
||||
"\n",
|
||||
" response_message = response[\"choices\"][0][\"message\"]\n",
|
||||
" \n",
|
||||
" available_functions = {\"enrich_entities\": enrich_entities} \n",
|
||||
" function_name = response_message[\"function_call\"][\"name\"]\n",
|
||||
" \n",
|
||||
" function_to_call = available_functions[function_name]\n",
|
||||
" logging.info(f\"function_to_call: {function_to_call}\")\n",
|
||||
"\n",
|
||||
" function_args = json.loads(response_message[\"function_call\"][\"arguments\"])\n",
|
||||
" logging.info(f\"function_args: {function_args}\")\n",
|
||||
"\n",
|
||||
" function_response = function_to_call(text, function_args)\n",
|
||||
"\n",
|
||||
" return {\"model_response\": response, \n",
|
||||
" \"function_response\": function_response}"
|
||||
]
|
||||
},
|
||||
{
|
||||
"cell_type": "markdown",
|
||||
"metadata": {},
|
||||
"source": [
|
||||
"### 5. Let's Enrich a Text with Wikipedia links"
|
||||
]
|
||||
},
|
||||
{
|
||||
"cell_type": "markdown",
|
||||
"metadata": {},
|
||||
"source": [
|
||||
"#### 5.1 Run OpenAI Task"
|
||||
]
|
||||
},
|
||||
{
|
||||
"cell_type": "code",
|
||||
"execution_count": 12,
|
||||
"metadata": {},
|
||||
"outputs": [
|
||||
{
|
||||
"name": "stderr",
|
||||
"output_type": "stream",
|
||||
"text": [
|
||||
" 2023-10-20 18:05:51,729 - INFO - function_to_call: <function enrich_entities at 0x0000021D30C462A0>\n",
|
||||
" 2023-10-20 18:05:51,730 - INFO - function_args: {'person': ['John Lennon', 'Paul McCartney', 'George Harrison', 'Ringo Starr'], 'org': ['The Beatles'], 'gpe': ['Liverpool'], 'date': ['1960']}\n",
|
||||
" 2023-10-20 18:06:09,858 - INFO - entity_link_dict: {'John Lennon': 'https://en.wikipedia.org/wiki/John_Lennon', 'Paul McCartney': 'https://en.wikipedia.org/wiki/Paul_McCartney', 'George Harrison': 'https://en.wikipedia.org/wiki/George_Harrison', 'Ringo Starr': 'https://en.wikipedia.org/wiki/Ringo_Starr', 'The Beatles': 'https://en.wikipedia.org/wiki/The_Beatles', 'Liverpool': 'https://en.wikipedia.org/wiki/Liverpool'}\n"
|
||||
]
|
||||
}
|
||||
],
|
||||
"source": [
|
||||
"text = \"\"\"The Beatles were an English rock band formed in Liverpool in 1960, comprising John Lennon, Paul McCartney, George Harrison, and Ringo Starr.\"\"\"\n",
|
||||
"result = run_openai_task(labels, text)"
|
||||
]
|
||||
},
|
||||
{
|
||||
"cell_type": "markdown",
|
||||
"metadata": {},
|
||||
"source": [
|
||||
"#### 5.2 Function Response"
|
||||
]
|
||||
},
|
||||
{
|
||||
"cell_type": "code",
|
||||
"execution_count": 13,
|
||||
"metadata": {},
|
||||
"outputs": [
|
||||
{
|
||||
"data": {
|
||||
"text/markdown": [
|
||||
"**Text:** The Beatles were an English rock band formed in Liverpool in 1960, comprising John Lennon, Paul McCartney, George Harrison, and Ringo Starr. \n",
|
||||
" **Enriched_Text:** [The Beatles](https://en.wikipedia.org/wiki/The_Beatles) were an English rock band formed in [Liverpool](https://en.wikipedia.org/wiki/Liverpool) in 1960, comprising [John Lennon](https://en.wikipedia.org/wiki/John_Lennon), [Paul McCartney](https://en.wikipedia.org/wiki/Paul_McCartney), [George Harrison](https://en.wikipedia.org/wiki/George_Harrison), and [Ringo Starr](https://en.wikipedia.org/wiki/Ringo_Starr)."
|
||||
],
|
||||
"text/plain": [
|
||||
"<IPython.core.display.Markdown object>"
|
||||
]
|
||||
},
|
||||
"metadata": {},
|
||||
"output_type": "display_data"
|
||||
}
|
||||
],
|
||||
"source": [
|
||||
"display(Markdown(f\"\"\"**Text:** {text} \n",
|
||||
" **Enriched_Text:** {result['function_response']}\"\"\"))"
|
||||
]
|
||||
},
|
||||
{
|
||||
"cell_type": "markdown",
|
||||
"metadata": {},
|
||||
"source": [
|
||||
"#### 5.3 Token Usage"
|
||||
]
|
||||
},
|
||||
{
|
||||
"cell_type": "markdown",
|
||||
"metadata": {},
|
||||
"source": [
|
||||
"To estimate the inference costs, we can parse the response's \"usage\" field. Detailed token costs per model are available in the [OpenAI Pricing Guide](https://openai.com/pricing):"
|
||||
]
|
||||
},
|
||||
{
|
||||
"cell_type": "code",
|
||||
"execution_count": 14,
|
||||
"metadata": {},
|
||||
"outputs": [
|
||||
{
|
||||
"name": "stdout",
|
||||
"output_type": "stream",
|
||||
"text": [
|
||||
"Token Usage\n",
|
||||
" Prompt: 331 tokens\n",
|
||||
" Completion: 47 tokens\n",
|
||||
" Cost estimation: $0.00059\n"
|
||||
]
|
||||
}
|
||||
],
|
||||
"source": [
|
||||
"# estimate inference cost assuming gpt-3.5-turbo (4K context)\n",
|
||||
"i_tokens = result[\"model_response\"][\"usage\"][\"prompt_tokens\"] \n",
|
||||
"o_tokens = result[\"model_response\"][\"usage\"][\"completion_tokens\"] \n",
|
||||
"\n",
|
||||
"i_cost = (i_tokens / 1000) * 0.0015\n",
|
||||
"o_cost = (o_tokens / 1000) * 0.002\n",
|
||||
"\n",
|
||||
"print(f\"\"\"Token Usage\n",
|
||||
" Prompt: {i_tokens} tokens\n",
|
||||
" Completion: {o_tokens} tokens\n",
|
||||
" Cost estimation: ${round(i_cost + o_cost, 5)}\"\"\")"
|
||||
]
|
||||
}
|
||||
],
|
||||
"metadata": {
|
||||
"kernelspec": {
|
||||
"display_name": "Python 3",
|
||||
"language": "python",
|
||||
"name": "python3"
|
||||
},
|
||||
"language_info": {
|
||||
"codemirror_mode": {
|
||||
"name": "ipython",
|
||||
"version": 3
|
||||
},
|
||||
"file_extension": ".py",
|
||||
"mimetype": "text/x-python",
|
||||
"name": "python",
|
||||
"nbconvert_exporter": "python",
|
||||
"pygments_lexer": "ipython3",
|
||||
"version": "3.11.1"
|
||||
}
|
||||
},
|
||||
"nbformat": 4,
|
||||
"nbformat_minor": 2
|
||||
}
|
File diff suppressed because one or more lines are too long
@ -0,0 +1,287 @@
|
||||
{
|
||||
"cells": [
|
||||
{
|
||||
"attachments": {},
|
||||
"cell_type": "markdown",
|
||||
"metadata": {},
|
||||
"source": [
|
||||
"# Azure chat completions example (preview)\n",
|
||||
"\n",
|
||||
"> Note: There is a newer version of the openai library available. See https://github.com/openai/openai-python/discussions/742\n",
|
||||
"\n",
|
||||
"This example will cover chat completions using the Azure OpenAI service."
|
||||
]
|
||||
},
|
||||
{
|
||||
"attachments": {},
|
||||
"cell_type": "markdown",
|
||||
"metadata": {},
|
||||
"source": [
|
||||
"## Setup\n"
|
||||
]
|
||||
},
|
||||
{
|
||||
"cell_type": "markdown",
|
||||
"metadata": {},
|
||||
"source": [
|
||||
"First, we install the necessary dependencies."
|
||||
]
|
||||
},
|
||||
{
|
||||
"cell_type": "code",
|
||||
"execution_count": null,
|
||||
"metadata": {},
|
||||
"outputs": [],
|
||||
"source": [
|
||||
"! pip install \"openai>=0.28.1,<1.0.0\""
|
||||
]
|
||||
},
|
||||
{
|
||||
"cell_type": "markdown",
|
||||
"metadata": {},
|
||||
"source": [
|
||||
"For the following sections to work properly we first have to setup some things. Let's start with the `api_base` and `api_version`. To find your `api_base` go to https://portal.azure.com, find your resource and then under \"Resource Management\" -> \"Keys and Endpoints\" look for the \"Endpoint\" value."
|
||||
]
|
||||
},
|
||||
{
|
||||
"cell_type": "code",
|
||||
"execution_count": null,
|
||||
"metadata": {},
|
||||
"outputs": [],
|
||||
"source": [
|
||||
"import os\n",
|
||||
"import openai"
|
||||
]
|
||||
},
|
||||
{
|
||||
"cell_type": "code",
|
||||
"execution_count": null,
|
||||
"metadata": {},
|
||||
"outputs": [],
|
||||
"source": [
|
||||
"openai.api_version = '2023-05-15'\n",
|
||||
"openai.api_base = '' # Please add your endpoint here"
|
||||
]
|
||||
},
|
||||
{
|
||||
"attachments": {},
|
||||
"cell_type": "markdown",
|
||||
"metadata": {},
|
||||
"source": [
|
||||
"We next have to setup the `api_type` and `api_key`. We can either get the key from the portal or we can get it through Microsoft Active Directory Authentication. Depending on this the `api_type` is either `azure` or `azure_ad`."
|
||||
]
|
||||
},
|
||||
{
|
||||
"attachments": {},
|
||||
"cell_type": "markdown",
|
||||
"metadata": {},
|
||||
"source": [
|
||||
"### Setup: Portal\n",
|
||||
"Let's first look at getting the key from the portal. Go to https://portal.azure.com, find your resource and then under \"Resource Management\" -> \"Keys and Endpoints\" look for one of the \"Keys\" values."
|
||||
]
|
||||
},
|
||||
{
|
||||
"cell_type": "code",
|
||||
"execution_count": null,
|
||||
"metadata": {},
|
||||
"outputs": [],
|
||||
"source": [
|
||||
"openai.api_type = 'azure'\n",
|
||||
"openai.api_key = os.environ[\"OPENAI_API_KEY\"]\n"
|
||||
]
|
||||
},
|
||||
{
|
||||
"attachments": {},
|
||||
"cell_type": "markdown",
|
||||
"metadata": {},
|
||||
"source": [
|
||||
"> Note: In this example, we configured the library to use the Azure API by setting the variables in code. For development, consider setting the environment variables instead:\n",
|
||||
"\n",
|
||||
"```\n",
|
||||
"OPENAI_API_BASE\n",
|
||||
"OPENAI_API_KEY\n",
|
||||
"OPENAI_API_TYPE\n",
|
||||
"OPENAI_API_VERSION\n",
|
||||
"```"
|
||||
]
|
||||
},
|
||||
{
|
||||
"attachments": {},
|
||||
"cell_type": "markdown",
|
||||
"metadata": {},
|
||||
"source": [
|
||||
"### (Optional) Setup: Microsoft Active Directory Authentication\n",
|
||||
"Let's now see how we can get a key via Microsoft Active Directory Authentication. Uncomment the following code if you want to use Active Directory Authentication instead of keys from the portal."
|
||||
]
|
||||
},
|
||||
{
|
||||
"cell_type": "code",
|
||||
"execution_count": null,
|
||||
"metadata": {},
|
||||
"outputs": [],
|
||||
"source": [
|
||||
"# from azure.identity import DefaultAzureCredential\n",
|
||||
"\n",
|
||||
"# default_credential = DefaultAzureCredential()\n",
|
||||
"# token = default_credential.get_token(\"https://cognitiveservices.azure.com/.default\")\n",
|
||||
"\n",
|
||||
"# openai.api_type = 'azure_ad'\n",
|
||||
"# openai.api_key = token.token"
|
||||
]
|
||||
},
|
||||
{
|
||||
"attachments": {},
|
||||
"cell_type": "markdown",
|
||||
"metadata": {},
|
||||
"source": [
|
||||
"A token is valid for a period of time, after which it will expire. To ensure a valid token is sent with every request, you can refresh an expiring token by hooking into requests.auth:"
|
||||
]
|
||||
},
|
||||
{
|
||||
"cell_type": "code",
|
||||
"execution_count": null,
|
||||
"metadata": {},
|
||||
"outputs": [],
|
||||
"source": [
|
||||
"import typing\n",
|
||||
"import time\n",
|
||||
"import requests\n",
|
||||
"if typing.TYPE_CHECKING:\n",
|
||||
" from azure.core.credentials import TokenCredential\n",
|
||||
"\n",
|
||||
"class TokenRefresh(requests.auth.AuthBase):\n",
|
||||
"\n",
|
||||
" def __init__(self, credential: \"TokenCredential\", scopes: typing.List[str]) -> None:\n",
|
||||
" self.credential = credential\n",
|
||||
" self.scopes = scopes\n",
|
||||
" self.cached_token: typing.Optional[str] = None\n",
|
||||
"\n",
|
||||
" def __call__(self, req):\n",
|
||||
" if not self.cached_token or self.cached_token.expires_on - time.time() < 300:\n",
|
||||
" self.cached_token = self.credential.get_token(*self.scopes)\n",
|
||||
" req.headers[\"Authorization\"] = f\"Bearer {self.cached_token.token}\"\n",
|
||||
" return req\n",
|
||||
"\n",
|
||||
"session = requests.Session()\n",
|
||||
"session.auth = TokenRefresh(default_credential, [\"https://cognitiveservices.azure.com/.default\"])\n",
|
||||
"\n",
|
||||
"openai.requestssession = session"
|
||||
]
|
||||
},
|
||||
{
|
||||
"attachments": {},
|
||||
"cell_type": "markdown",
|
||||
"metadata": {},
|
||||
"source": [
|
||||
"## Deployments\n",
|
||||
"In this section we are going to create a deployment using the `gpt-35-turbo` model that we can then use to create chat completions."
|
||||
]
|
||||
},
|
||||
{
|
||||
"attachments": {},
|
||||
"cell_type": "markdown",
|
||||
"metadata": {},
|
||||
"source": [
|
||||
"### Deployments: Create manually\n",
|
||||
"Let's create a deployment using the `gpt-35-turbo` model. Go to https://portal.azure.com, find your resource and then under \"Resource Management\" -> \"Model deployments\" create a new `gpt-35-turbo` deployment. "
|
||||
]
|
||||
},
|
||||
{
|
||||
"cell_type": "code",
|
||||
"execution_count": null,
|
||||
"metadata": {},
|
||||
"outputs": [],
|
||||
"source": [
|
||||
"deployment_id = '' # Fill in the deployment id from the portal here"
|
||||
]
|
||||
},
|
||||
{
|
||||
"attachments": {},
|
||||
"cell_type": "markdown",
|
||||
"metadata": {},
|
||||
"source": [
|
||||
"### Create chat completion\n",
|
||||
"Now let's send a sample chat completion to the deployment."
|
||||
]
|
||||
},
|
||||
{
|
||||
"cell_type": "code",
|
||||
"execution_count": null,
|
||||
"metadata": {},
|
||||
"outputs": [],
|
||||
"source": [
|
||||
"# For all possible arguments see https://platform.openai.com/docs/api-reference/chat-completions/create\n",
|
||||
"response = openai.ChatCompletion.create(\n",
|
||||
" deployment_id=deployment_id,\n",
|
||||
" messages=[\n",
|
||||
" {\"role\": \"system\", \"content\": \"You are a helpful assistant.\"},\n",
|
||||
" {\"role\": \"user\", \"content\": \"Knock knock.\"},\n",
|
||||
" {\"role\": \"assistant\", \"content\": \"Who's there?\"},\n",
|
||||
" {\"role\": \"user\", \"content\": \"Orange.\"},\n",
|
||||
" ],\n",
|
||||
" temperature=0,\n",
|
||||
")\n",
|
||||
"\n",
|
||||
"print(f\"{response.choices[0].message.role}: {response.choices[0].message.content}\")"
|
||||
]
|
||||
},
|
||||
{
|
||||
"attachments": {},
|
||||
"cell_type": "markdown",
|
||||
"metadata": {},
|
||||
"source": [
|
||||
"We can also stream the response.\n"
|
||||
]
|
||||
},
|
||||
{
|
||||
"cell_type": "code",
|
||||
"execution_count": null,
|
||||
"metadata": {},
|
||||
"outputs": [],
|
||||
"source": [
|
||||
"response = openai.ChatCompletion.create(\n",
|
||||
" deployment_id=deployment_id,\n",
|
||||
" messages=[\n",
|
||||
" {\"role\": \"system\", \"content\": \"You are a helpful assistant.\"},\n",
|
||||
" {\"role\": \"user\", \"content\": \"Knock knock.\"},\n",
|
||||
" {\"role\": \"assistant\", \"content\": \"Who's there?\"},\n",
|
||||
" {\"role\": \"user\", \"content\": \"Orange.\"},\n",
|
||||
" ],\n",
|
||||
" temperature=0,\n",
|
||||
" stream=True\n",
|
||||
")\n",
|
||||
"\n",
|
||||
"for chunk in response:\n",
|
||||
" if len(chunk.choices) > 0:\n",
|
||||
" delta = chunk.choices[0].delta\n",
|
||||
"\n",
|
||||
" if \"role\" in delta.keys():\n",
|
||||
" print(delta.role + \": \", end=\"\", flush=True)\n",
|
||||
" if \"content\" in delta.keys():\n",
|
||||
" print(delta.content, end=\"\", flush=True)"
|
||||
]
|
||||
}
|
||||
],
|
||||
"metadata": {
|
||||
"kernelspec": {
|
||||
"display_name": "Python 3",
|
||||
"language": "python",
|
||||
"name": "python3"
|
||||
},
|
||||
"language_info": {
|
||||
"codemirror_mode": {
|
||||
"name": "ipython",
|
||||
"version": 3
|
||||
},
|
||||
"file_extension": ".py",
|
||||
"mimetype": "text/x-python",
|
||||
"name": "python",
|
||||
"nbconvert_exporter": "python",
|
||||
"pygments_lexer": "ipython3",
|
||||
"version": "3.11.3"
|
||||
},
|
||||
"orig_nbformat": 4
|
||||
},
|
||||
"nbformat": 4,
|
||||
"nbformat_minor": 2
|
||||
}
|
File diff suppressed because one or more lines are too long
@ -0,0 +1,247 @@
|
||||
{
|
||||
"cells": [
|
||||
{
|
||||
"attachments": {},
|
||||
"cell_type": "markdown",
|
||||
"metadata": {},
|
||||
"source": [
|
||||
"# Azure completions example\n",
|
||||
"\n",
|
||||
"> Note: There is a newer version of the openai library available. See https://github.com/openai/openai-python/discussions/742\n",
|
||||
"\n",
|
||||
"This example will cover completions using the Azure OpenAI service."
|
||||
]
|
||||
},
|
||||
{
|
||||
"attachments": {},
|
||||
"cell_type": "markdown",
|
||||
"metadata": {},
|
||||
"source": [
|
||||
"## Setup\n"
|
||||
]
|
||||
},
|
||||
{
|
||||
"cell_type": "markdown",
|
||||
"metadata": {},
|
||||
"source": [
|
||||
"First, we install the necessary dependencies."
|
||||
]
|
||||
},
|
||||
{
|
||||
"cell_type": "code",
|
||||
"execution_count": null,
|
||||
"metadata": {},
|
||||
"outputs": [],
|
||||
"source": [
|
||||
"! pip install \"openai>=0.28.1,<1.0.0\""
|
||||
]
|
||||
},
|
||||
{
|
||||
"cell_type": "markdown",
|
||||
"metadata": {},
|
||||
"source": [
|
||||
"For the following sections to work properly we first have to setup some things. Let's start with the `api_base` and `api_version`. To find your `api_base` go to https://portal.azure.com, find your resource and then under \"Resource Management\" -> \"Keys and Endpoints\" look for the \"Endpoint\" value."
|
||||
]
|
||||
},
|
||||
{
|
||||
"cell_type": "code",
|
||||
"execution_count": null,
|
||||
"metadata": {},
|
||||
"outputs": [],
|
||||
"source": [
|
||||
"import os\n",
|
||||
"import openai"
|
||||
]
|
||||
},
|
||||
{
|
||||
"cell_type": "code",
|
||||
"execution_count": null,
|
||||
"metadata": {},
|
||||
"outputs": [],
|
||||
"source": [
|
||||
"openai.api_version = '2023-05-15'\n",
|
||||
"openai.api_base = '' # Please add your endpoint here"
|
||||
]
|
||||
},
|
||||
{
|
||||
"attachments": {},
|
||||
"cell_type": "markdown",
|
||||
"metadata": {},
|
||||
"source": [
|
||||
"We next have to setup the `api_type` and `api_key`. We can either get the key from the portal or we can get it through Microsoft Active Directory Authentication. Depending on this the `api_type` is either `azure` or `azure_ad`."
|
||||
]
|
||||
},
|
||||
{
|
||||
"attachments": {},
|
||||
"cell_type": "markdown",
|
||||
"metadata": {},
|
||||
"source": [
|
||||
"### Setup: Portal\n",
|
||||
"Let's first look at getting the key from the portal. Go to https://portal.azure.com, find your resource and then under \"Resource Management\" -> \"Keys and Endpoints\" look for one of the \"Keys\" values."
|
||||
]
|
||||
},
|
||||
{
|
||||
"cell_type": "code",
|
||||
"execution_count": null,
|
||||
"metadata": {},
|
||||
"outputs": [],
|
||||
"source": [
|
||||
"openai.api_type = 'azure'\n",
|
||||
"openai.api_key = os.environ[\"OPENAI_API_KEY\"]"
|
||||
]
|
||||
},
|
||||
{
|
||||
"attachments": {},
|
||||
"cell_type": "markdown",
|
||||
"metadata": {},
|
||||
"source": [
|
||||
"> Note: In this example, we configured the library to use the Azure API by setting the variables in code. For development, consider setting the environment variables instead:\n",
|
||||
"\n",
|
||||
"```\n",
|
||||
"OPENAI_API_BASE\n",
|
||||
"OPENAI_API_KEY\n",
|
||||
"OPENAI_API_TYPE\n",
|
||||
"OPENAI_API_VERSION\n",
|
||||
"```"
|
||||
]
|
||||
},
|
||||
{
|
||||
"attachments": {},
|
||||
"cell_type": "markdown",
|
||||
"metadata": {},
|
||||
"source": [
|
||||
"### (Optional) Setup: Microsoft Active Directory Authentication\n",
|
||||
"Let's now see how we can get a key via Microsoft Active Directory Authentication. Uncomment the following code if you want to use Active Directory Authentication instead of keys from the portal."
|
||||
]
|
||||
},
|
||||
{
|
||||
"cell_type": "code",
|
||||
"execution_count": null,
|
||||
"metadata": {},
|
||||
"outputs": [],
|
||||
"source": [
|
||||
"# from azure.identity import DefaultAzureCredential\n",
|
||||
"\n",
|
||||
"# default_credential = DefaultAzureCredential()\n",
|
||||
"# token = default_credential.get_token(\"https://cognitiveservices.azure.com/.default\")\n",
|
||||
"\n",
|
||||
"# openai.api_type = 'azure_ad'\n",
|
||||
"# openai.api_key = token.token"
|
||||
]
|
||||
},
|
||||
{
|
||||
"attachments": {},
|
||||
"cell_type": "markdown",
|
||||
"metadata": {},
|
||||
"source": [
|
||||
"A token is valid for a period of time, after which it will expire. To ensure a valid token is sent with every request, you can refresh an expiring token by hooking into requests.auth:"
|
||||
]
|
||||
},
|
||||
{
|
||||
"cell_type": "code",
|
||||
"execution_count": null,
|
||||
"metadata": {},
|
||||
"outputs": [],
|
||||
"source": [
|
||||
"import typing\n",
|
||||
"import time\n",
|
||||
"import requests\n",
|
||||
"if typing.TYPE_CHECKING:\n",
|
||||
" from azure.core.credentials import TokenCredential\n",
|
||||
"\n",
|
||||
"class TokenRefresh(requests.auth.AuthBase):\n",
|
||||
"\n",
|
||||
" def __init__(self, credential: \"TokenCredential\", scopes: typing.List[str]) -> None:\n",
|
||||
" self.credential = credential\n",
|
||||
" self.scopes = scopes\n",
|
||||
" self.cached_token: typing.Optional[str] = None\n",
|
||||
"\n",
|
||||
" def __call__(self, req):\n",
|
||||
" if not self.cached_token or self.cached_token.expires_on - time.time() < 300:\n",
|
||||
" self.cached_token = self.credential.get_token(*self.scopes)\n",
|
||||
" req.headers[\"Authorization\"] = f\"Bearer {self.cached_token.token}\"\n",
|
||||
" return req\n",
|
||||
"\n",
|
||||
"session = requests.Session()\n",
|
||||
"session.auth = TokenRefresh(default_credential, [\"https://cognitiveservices.azure.com/.default\"])\n",
|
||||
"\n",
|
||||
"openai.requestssession = session"
|
||||
]
|
||||
},
|
||||
{
|
||||
"attachments": {},
|
||||
"cell_type": "markdown",
|
||||
"metadata": {},
|
||||
"source": [
|
||||
"## Deployments\n",
|
||||
"In this section we are going to create a deployment using the `text-davinci-002` model that we can then use to create completions."
|
||||
]
|
||||
},
|
||||
{
|
||||
"attachments": {},
|
||||
"cell_type": "markdown",
|
||||
"metadata": {},
|
||||
"source": [
|
||||
"### Deployments: Create manually\n",
|
||||
"Create a new deployment by going to your Resource in your portal under \"Resource Management\" -> \"Model deployments\". Select `text-davinci-002` as the model."
|
||||
]
|
||||
},
|
||||
{
|
||||
"cell_type": "code",
|
||||
"execution_count": null,
|
||||
"metadata": {},
|
||||
"outputs": [],
|
||||
"source": [
|
||||
"deployment_id = '' # Fill in the deployment id from the portal here"
|
||||
]
|
||||
},
|
||||
{
|
||||
"attachments": {},
|
||||
"cell_type": "markdown",
|
||||
"metadata": {},
|
||||
"source": [
|
||||
"### Completions\n",
|
||||
"Now let's send a sample completion to the deployment."
|
||||
]
|
||||
},
|
||||
{
|
||||
"cell_type": "code",
|
||||
"execution_count": null,
|
||||
"metadata": {},
|
||||
"outputs": [],
|
||||
"source": [
|
||||
"prompt = \"The food was delicious and the waiter\"\n",
|
||||
"completion = openai.Completion.create(deployment_id=deployment_id,\n",
|
||||
" prompt=prompt, stop=\".\", temperature=0)\n",
|
||||
" \n",
|
||||
"print(f\"{prompt}{completion['choices'][0]['text']}.\")"
|
||||
]
|
||||
}
|
||||
],
|
||||
"metadata": {
|
||||
"kernelspec": {
|
||||
"display_name": "Python 3 (ipykernel)",
|
||||
"language": "python",
|
||||
"name": "python3"
|
||||
},
|
||||
"language_info": {
|
||||
"codemirror_mode": {
|
||||
"name": "ipython",
|
||||
"version": 3
|
||||
},
|
||||
"file_extension": ".py",
|
||||
"mimetype": "text/x-python",
|
||||
"name": "python",
|
||||
"nbconvert_exporter": "python",
|
||||
"pygments_lexer": "ipython3",
|
||||
"version": "3.11.3"
|
||||
},
|
||||
"vscode": {
|
||||
"interpreter": {
|
||||
"hash": "3a5103089ab7e7c666b279eeded403fcec76de49a40685dbdfe9f9c78ad97c17"
|
||||
}
|
||||
}
|
||||
},
|
||||
"nbformat": 4,
|
||||
"nbformat_minor": 2
|
||||
}
|
@ -0,0 +1,279 @@
|
||||
{
|
||||
"cells": [
|
||||
{
|
||||
"attachments": {},
|
||||
"cell_type": "markdown",
|
||||
"metadata": {},
|
||||
"source": [
|
||||
"# Azure embeddings example\n",
|
||||
"\n",
|
||||
"> Note: There is a newer version of the openai library available. See https://github.com/openai/openai-python/discussions/742\n",
|
||||
"\n",
|
||||
"This example will cover embeddings using the Azure OpenAI service."
|
||||
]
|
||||
},
|
||||
{
|
||||
"attachments": {},
|
||||
"cell_type": "markdown",
|
||||
"metadata": {},
|
||||
"source": [
|
||||
"## Setup"
|
||||
]
|
||||
},
|
||||
{
|
||||
"cell_type": "markdown",
|
||||
"metadata": {},
|
||||
"source": [
|
||||
"First, we install the necessary dependencies."
|
||||
]
|
||||
},
|
||||
{
|
||||
"cell_type": "markdown",
|
||||
"metadata": {},
|
||||
"source": [
|
||||
"! pip install \"openai>=0.28.1,<1.0.0\""
|
||||
]
|
||||
},
|
||||
{
|
||||
"cell_type": "markdown",
|
||||
"metadata": {},
|
||||
"source": [
|
||||
"For the following sections to work properly we first have to setup some things. Let's start with the `api_base` and `api_version`. To find your `api_base` go to https://portal.azure.com, find your resource and then under \"Resource Management\" -> \"Keys and Endpoints\" look for the \"Endpoint\" value."
|
||||
]
|
||||
},
|
||||
{
|
||||
"cell_type": "code",
|
||||
"execution_count": null,
|
||||
"metadata": {},
|
||||
"outputs": [],
|
||||
"source": [
|
||||
"import os\n",
|
||||
"import openai"
|
||||
]
|
||||
},
|
||||
{
|
||||
"cell_type": "code",
|
||||
"execution_count": null,
|
||||
"metadata": {},
|
||||
"outputs": [],
|
||||
"source": [
|
||||
"openai.api_version = '2023-05-15'\n",
|
||||
"openai.api_base = '' # Please add your endpoint here"
|
||||
]
|
||||
},
|
||||
{
|
||||
"attachments": {},
|
||||
"cell_type": "markdown",
|
||||
"metadata": {},
|
||||
"source": [
|
||||
"We next have to setup the `api_type` and `api_key`. We can either get the key from the portal or we can get it through Microsoft Active Directory Authentication. Depending on this the `api_type` is either `azure` or `azure_ad`."
|
||||
]
|
||||
},
|
||||
{
|
||||
"attachments": {},
|
||||
"cell_type": "markdown",
|
||||
"metadata": {},
|
||||
"source": [
|
||||
"### Setup: Portal\n",
|
||||
"Let's first look at getting the key from the portal. Go to https://portal.azure.com, find your resource and then under \"Resource Management\" -> \"Keys and Endpoints\" look for one of the \"Keys\" values."
|
||||
]
|
||||
},
|
||||
{
|
||||
"cell_type": "code",
|
||||
"execution_count": null,
|
||||
"metadata": {},
|
||||
"outputs": [],
|
||||
"source": [
|
||||
"openai.api_type = 'azure'\n",
|
||||
"openai.api_key = os.environ[\"OPENAI_API_KEY\"]"
|
||||
]
|
||||
},
|
||||
{
|
||||
"attachments": {},
|
||||
"cell_type": "markdown",
|
||||
"metadata": {},
|
||||
"source": [
|
||||
"> Note: In this example, we configured the library to use the Azure API by setting the variables in code. For development, consider setting the environment variables instead:\n",
|
||||
"\n",
|
||||
"```\n",
|
||||
"OPENAI_API_BASE\n",
|
||||
"OPENAI_API_KEY\n",
|
||||
"OPENAI_API_TYPE\n",
|
||||
"OPENAI_API_VERSION\n",
|
||||
"```"
|
||||
]
|
||||
},
|
||||
{
|
||||
"attachments": {},
|
||||
"cell_type": "markdown",
|
||||
"metadata": {},
|
||||
"source": [
|
||||
"### (Optional) Setup: Microsoft Active Directory Authentication\n",
|
||||
"Let's now see how we can get a key via Microsoft Active Directory Authentication. Uncomment the following code if you want to use Active Directory Authentication instead of keys from the portal."
|
||||
]
|
||||
},
|
||||
{
|
||||
"cell_type": "code",
|
||||
"execution_count": null,
|
||||
"metadata": {},
|
||||
"outputs": [],
|
||||
"source": [
|
||||
"# from azure.identity import DefaultAzureCredential\n",
|
||||
"\n",
|
||||
"# default_credential = DefaultAzureCredential()\n",
|
||||
"# token = default_credential.get_token(\"https://cognitiveservices.azure.com/.default\")\n",
|
||||
"\n",
|
||||
"# openai.api_type = 'azure_ad'\n",
|
||||
"# openai.api_key = token.token"
|
||||
]
|
||||
},
|
||||
{
|
||||
"attachments": {},
|
||||
"cell_type": "markdown",
|
||||
"metadata": {},
|
||||
"source": [
|
||||
"A token is valid for a period of time, after which it will expire. To ensure a valid token is sent with every request, you can refresh an expiring token by hooking into requests.auth:"
|
||||
]
|
||||
},
|
||||
{
|
||||
"cell_type": "code",
|
||||
"execution_count": null,
|
||||
"metadata": {},
|
||||
"outputs": [],
|
||||
"source": [
|
||||
"import typing\n",
|
||||
"import time\n",
|
||||
"import requests\n",
|
||||
"if typing.TYPE_CHECKING:\n",
|
||||
" from azure.core.credentials import TokenCredential\n",
|
||||
"\n",
|
||||
"class TokenRefresh(requests.auth.AuthBase):\n",
|
||||
"\n",
|
||||
" def __init__(self, credential: \"TokenCredential\", scopes: typing.List[str]) -> None:\n",
|
||||
" self.credential = credential\n",
|
||||
" self.scopes = scopes\n",
|
||||
" self.cached_token: typing.Optional[str] = None\n",
|
||||
"\n",
|
||||
" def __call__(self, req):\n",
|
||||
" if not self.cached_token or self.cached_token.expires_on - time.time() < 300:\n",
|
||||
" self.cached_token = self.credential.get_token(*self.scopes)\n",
|
||||
" req.headers[\"Authorization\"] = f\"Bearer {self.cached_token.token}\"\n",
|
||||
" return req\n",
|
||||
"\n",
|
||||
"session = requests.Session()\n",
|
||||
"session.auth = TokenRefresh(default_credential, [\"https://cognitiveservices.azure.com/.default\"])\n",
|
||||
"\n",
|
||||
"openai.requestssession = session"
|
||||
]
|
||||
},
|
||||
{
|
||||
"attachments": {},
|
||||
"cell_type": "markdown",
|
||||
"metadata": {},
|
||||
"source": [
|
||||
"## Deployments\n",
|
||||
"In this section we are going to create a deployment that we can use to create embeddings."
|
||||
]
|
||||
},
|
||||
{
|
||||
"attachments": {},
|
||||
"cell_type": "markdown",
|
||||
"metadata": {},
|
||||
"source": [
|
||||
"### Deployments: Create manually\n",
|
||||
"Let's create a deployment using the `text-similarity-curie-001` model. Create a new deployment by going to your Resource in your portal under \"Resource Management\" -> \"Model deployments\"."
|
||||
]
|
||||
},
|
||||
{
|
||||
"cell_type": "code",
|
||||
"execution_count": null,
|
||||
"metadata": {},
|
||||
"outputs": [],
|
||||
"source": [
|
||||
"deployment_id = '' # Fill in the deployment id from the portal here"
|
||||
]
|
||||
},
|
||||
{
|
||||
"attachments": {},
|
||||
"cell_type": "markdown",
|
||||
"metadata": {},
|
||||
"source": [
|
||||
"### Deployments: Listing\n",
|
||||
"Now because creating a new deployment takes a long time, let's look in the subscription for an already finished deployment that succeeded."
|
||||
]
|
||||
},
|
||||
{
|
||||
"cell_type": "code",
|
||||
"execution_count": null,
|
||||
"metadata": {},
|
||||
"outputs": [],
|
||||
"source": [
|
||||
"print('While deployment running, selecting a completed one that supports embeddings.')\n",
|
||||
"deployment_id = None\n",
|
||||
"result = openai.Deployment.list()\n",
|
||||
"for deployment in result.data:\n",
|
||||
" if deployment[\"status\"] != \"succeeded\":\n",
|
||||
" continue\n",
|
||||
" \n",
|
||||
" model = openai.Model.retrieve(deployment[\"model\"])\n",
|
||||
" if model[\"capabilities\"][\"embeddings\"] != True:\n",
|
||||
" continue\n",
|
||||
" \n",
|
||||
" deployment_id = deployment[\"id\"]\n",
|
||||
" break\n",
|
||||
"\n",
|
||||
"if not deployment_id:\n",
|
||||
" print('No deployment with status: succeeded found.')\n",
|
||||
"else:\n",
|
||||
" print(f'Found a succeeded deployment that supports embeddings with id: {deployment_id}.')"
|
||||
]
|
||||
},
|
||||
{
|
||||
"attachments": {},
|
||||
"cell_type": "markdown",
|
||||
"metadata": {},
|
||||
"source": [
|
||||
"### Embeddings\n",
|
||||
"Now let's send a sample embedding to the deployment."
|
||||
]
|
||||
},
|
||||
{
|
||||
"cell_type": "code",
|
||||
"execution_count": null,
|
||||
"metadata": {},
|
||||
"outputs": [],
|
||||
"source": [
|
||||
"embeddings = openai.Embedding.create(deployment_id=deployment_id,\n",
|
||||
" input=\"The food was delicious and the waiter...\")\n",
|
||||
" \n",
|
||||
"print(embeddings)"
|
||||
]
|
||||
}
|
||||
],
|
||||
"metadata": {
|
||||
"kernelspec": {
|
||||
"display_name": "Python 3",
|
||||
"language": "python",
|
||||
"name": "python3"
|
||||
},
|
||||
"language_info": {
|
||||
"codemirror_mode": {
|
||||
"name": "ipython",
|
||||
"version": 3
|
||||
},
|
||||
"file_extension": ".py",
|
||||
"mimetype": "text/x-python",
|
||||
"name": "python",
|
||||
"nbconvert_exporter": "python",
|
||||
"pygments_lexer": "ipython3",
|
||||
"version": "3.11.3"
|
||||
},
|
||||
"vscode": {
|
||||
"interpreter": {
|
||||
"hash": "3a5103089ab7e7c666b279eeded403fcec76de49a40685dbdfe9f9c78ad97c17"
|
||||
}
|
||||
}
|
||||
},
|
||||
"nbformat": 4,
|
||||
"nbformat_minor": 2
|
||||
}
|
@ -0,0 +1,449 @@
|
||||
{
|
||||
"cells": [
|
||||
{
|
||||
"attachments": {},
|
||||
"cell_type": "markdown",
|
||||
"metadata": {},
|
||||
"source": [
|
||||
"# Azure functions example\n",
|
||||
"\n",
|
||||
"> Note: There is a newer version of the openai library available. See https://github.com/openai/openai-python/discussions/742\n",
|
||||
"\n",
|
||||
"This notebook shows how to use the function calling capability with the Azure OpenAI service. Functions allow a caller of chat completions to define capabilities that the model can use to extend its\n",
|
||||
"functionality into external tools and data sources.\n",
|
||||
"\n",
|
||||
"You can read more about chat functions on OpenAI's blog: https://openai.com/blog/function-calling-and-other-api-updates\n",
|
||||
"\n",
|
||||
"**NOTE**: Chat functions require model versions beginning with gpt-4 and gpt-35-turbo's `-0613` labels. They are not supported by older versions of the models."
|
||||
]
|
||||
},
|
||||
{
|
||||
"attachments": {},
|
||||
"cell_type": "markdown",
|
||||
"metadata": {},
|
||||
"source": [
|
||||
"## Setup\n",
|
||||
"\n",
|
||||
"First, we install the necessary dependencies."
|
||||
]
|
||||
},
|
||||
{
|
||||
"cell_type": "code",
|
||||
"execution_count": null,
|
||||
"metadata": {},
|
||||
"outputs": [],
|
||||
"source": [
|
||||
"! pip install \"openai>=0.28.1,<1.0.0\"\n",
|
||||
"# (Optional) If you want to use Microsoft Active Directory\n",
|
||||
"! pip install azure-identity"
|
||||
]
|
||||
},
|
||||
{
|
||||
"cell_type": "code",
|
||||
"execution_count": 3,
|
||||
"metadata": {},
|
||||
"outputs": [],
|
||||
"source": [
|
||||
"import os\n",
|
||||
"import openai"
|
||||
]
|
||||
},
|
||||
{
|
||||
"attachments": {},
|
||||
"cell_type": "markdown",
|
||||
"metadata": {},
|
||||
"source": [
|
||||
"\n",
|
||||
"Additionally, to properly access the Azure OpenAI Service, we need to create the proper resources at the [Azure Portal](https://portal.azure.com) (you can check a detailed guide on how to do this in the [Microsoft Docs](https://learn.microsoft.com/en-us/azure/cognitive-services/openai/how-to/create-resource?pivots=web-portal))\n",
|
||||
"\n",
|
||||
"Once the resource is created, the first thing we need to use is its endpoint. You can get the endpoint by looking at the *\"Keys and Endpoints\"* section under the *\"Resource Management\"* section. Having this, we will set up the SDK using this information:"
|
||||
]
|
||||
},
|
||||
{
|
||||
"cell_type": "code",
|
||||
"execution_count": 27,
|
||||
"metadata": {},
|
||||
"outputs": [],
|
||||
"source": [
|
||||
"openai.api_base = \"\" # Add your endpoint here\n",
|
||||
"\n",
|
||||
"# functions is only supported by the 2023-07-01-preview API version\n",
|
||||
"openai.api_version = \"2023-07-01-preview\""
|
||||
]
|
||||
},
|
||||
{
|
||||
"attachments": {},
|
||||
"cell_type": "markdown",
|
||||
"metadata": {},
|
||||
"source": [
|
||||
"### Authentication\n",
|
||||
"\n",
|
||||
"The Azure OpenAI service supports multiple authentication mechanisms that include API keys and Azure credentials."
|
||||
]
|
||||
},
|
||||
{
|
||||
"cell_type": "code",
|
||||
"execution_count": 4,
|
||||
"metadata": {},
|
||||
"outputs": [],
|
||||
"source": [
|
||||
"use_azure_active_directory = False"
|
||||
]
|
||||
},
|
||||
{
|
||||
"attachments": {},
|
||||
"cell_type": "markdown",
|
||||
"metadata": {},
|
||||
"source": [
|
||||
"\n",
|
||||
"#### Authentication using API key\n",
|
||||
"\n",
|
||||
"To set up the OpenAI SDK to use an *Azure API Key*, we need to set up the `api_type` to `azure` and set `api_key` to a key associated with your endpoint (you can find this key in *\"Keys and Endpoints\"* under *\"Resource Management\"* in the [Azure Portal](https://portal.azure.com))"
|
||||
]
|
||||
},
|
||||
{
|
||||
"cell_type": "code",
|
||||
"execution_count": null,
|
||||
"metadata": {},
|
||||
"outputs": [],
|
||||
"source": [
|
||||
"if not use_azure_active_directory:\n",
|
||||
" openai.api_type = \"azure\"\n",
|
||||
" openai.api_key = os.environ[\"OPENAI_API_KEY\"]"
|
||||
]
|
||||
},
|
||||
{
|
||||
"attachments": {},
|
||||
"cell_type": "markdown",
|
||||
"metadata": {},
|
||||
"source": [
|
||||
"> Note: In this example, we configured the library to use the Azure API by setting the variables in code. For development, consider setting the environment variables instead:\n",
|
||||
"\n",
|
||||
"```\n",
|
||||
"OPENAI_API_BASE\n",
|
||||
"OPENAI_API_KEY\n",
|
||||
"OPENAI_API_TYPE\n",
|
||||
"OPENAI_API_VERSION\n",
|
||||
"```"
|
||||
]
|
||||
},
|
||||
{
|
||||
"attachments": {},
|
||||
"cell_type": "markdown",
|
||||
"metadata": {},
|
||||
"source": [
|
||||
"#### Authentication using Microsoft Active Directory\n",
|
||||
"Let's now see how we can get a key via Microsoft Active Directory Authentication."
|
||||
]
|
||||
},
|
||||
{
|
||||
"cell_type": "code",
|
||||
"execution_count": null,
|
||||
"metadata": {},
|
||||
"outputs": [],
|
||||
"source": [
|
||||
"from azure.identity import DefaultAzureCredential\n",
|
||||
"\n",
|
||||
"if use_azure_active_directory:\n",
|
||||
" default_credential = DefaultAzureCredential()\n",
|
||||
" token = default_credential.get_token(\"https://cognitiveservices.azure.com/.default\")\n",
|
||||
"\n",
|
||||
" openai.api_type = \"azure_ad\"\n",
|
||||
" openai.api_key = token.token"
|
||||
]
|
||||
},
|
||||
{
|
||||
"attachments": {},
|
||||
"cell_type": "markdown",
|
||||
"metadata": {},
|
||||
"source": [
|
||||
"A token is valid for a period of time, after which it will expire. To ensure a valid token is sent with every request, you can refresh an expiring token by hooking into requests.auth:"
|
||||
]
|
||||
},
|
||||
{
|
||||
"cell_type": "code",
|
||||
"execution_count": null,
|
||||
"metadata": {},
|
||||
"outputs": [],
|
||||
"source": [
|
||||
"import typing\n",
|
||||
"import time\n",
|
||||
"import requests\n",
|
||||
"\n",
|
||||
"if typing.TYPE_CHECKING:\n",
|
||||
" from azure.core.credentials import TokenCredential\n",
|
||||
"\n",
|
||||
"class TokenRefresh(requests.auth.AuthBase):\n",
|
||||
"\n",
|
||||
" def __init__(self, credential: \"TokenCredential\", scopes: typing.List[str]) -> None:\n",
|
||||
" self.credential = credential\n",
|
||||
" self.scopes = scopes\n",
|
||||
" self.cached_token: typing.Optional[str] = None\n",
|
||||
"\n",
|
||||
" def __call__(self, req):\n",
|
||||
" if not self.cached_token or self.cached_token.expires_on - time.time() < 300:\n",
|
||||
" self.cached_token = self.credential.get_token(*self.scopes)\n",
|
||||
" req.headers[\"Authorization\"] = f\"Bearer {self.cached_token.token}\"\n",
|
||||
" return req\n",
|
||||
"\n",
|
||||
"if use_azure_active_directory:\n",
|
||||
" session = requests.Session()\n",
|
||||
" session.auth = TokenRefresh(default_credential, [\"https://cognitiveservices.azure.com/.default\"])\n",
|
||||
"\n",
|
||||
" openai.requestssession = session"
|
||||
]
|
||||
},
|
||||
{
|
||||
"attachments": {},
|
||||
"cell_type": "markdown",
|
||||
"metadata": {},
|
||||
"source": [
|
||||
"## Functions\n",
|
||||
"\n",
|
||||
"With setup and authentication complete, you can now use functions with the Azure OpenAI service. This will be split into a few steps:\n",
|
||||
"\n",
|
||||
"1. Define the function(s)\n",
|
||||
"2. Pass function definition(s) into chat completions API\n",
|
||||
"3. Call function with arguments from the response\n",
|
||||
"4. Feed function response back into chat completions API"
|
||||
]
|
||||
},
|
||||
{
|
||||
"attachments": {},
|
||||
"cell_type": "markdown",
|
||||
"metadata": {},
|
||||
"source": [
|
||||
"#### 1. Define the function(s)\n",
|
||||
"\n",
|
||||
"A list of functions can be defined, each containing the name of the function, an optional description, and the parameters the function accepts (described as a JSON schema)."
|
||||
]
|
||||
},
|
||||
{
|
||||
"cell_type": "code",
|
||||
"execution_count": 21,
|
||||
"metadata": {},
|
||||
"outputs": [],
|
||||
"source": [
|
||||
"functions = [\n",
|
||||
" {\n",
|
||||
" \"name\": \"get_current_weather\",\n",
|
||||
" \"description\": \"Get the current weather\",\n",
|
||||
" \"parameters\": {\n",
|
||||
" \"type\": \"object\",\n",
|
||||
" \"properties\": {\n",
|
||||
" \"location\": {\n",
|
||||
" \"type\": \"string\",\n",
|
||||
" \"description\": \"The city and state, e.g. San Francisco, CA\",\n",
|
||||
" },\n",
|
||||
" \"format\": {\n",
|
||||
" \"type\": \"string\",\n",
|
||||
" \"enum\": [\"celsius\", \"fahrenheit\"],\n",
|
||||
" \"description\": \"The temperature unit to use. Infer this from the users location.\",\n",
|
||||
" },\n",
|
||||
" },\n",
|
||||
" \"required\": [\"location\"],\n",
|
||||
" },\n",
|
||||
" }\n",
|
||||
"]"
|
||||
]
|
||||
},
|
||||
{
|
||||
"attachments": {},
|
||||
"cell_type": "markdown",
|
||||
"metadata": {},
|
||||
"source": [
|
||||
"#### 2. Pass function definition(s) into chat completions API\n",
|
||||
"\n",
|
||||
"Now we can pass the function into the chat completions API. If the model determines it should call the function, a `finish_reason` of \"function_call\" will be populated on the choice and the details of which function to call and its arguments will be present in the `message`. Optionally, you can set the `function_call` keyword argument to force the model to call a particular function (e.g. `function_call={\"name\": get_current_weather}`). By default, this is set to `auto`, allowing the model to choose whether to call the function or not. "
|
||||
]
|
||||
},
|
||||
{
|
||||
"cell_type": "code",
|
||||
"execution_count": null,
|
||||
"metadata": {},
|
||||
"outputs": [
|
||||
{
|
||||
"name": "stdout",
|
||||
"output_type": "stream",
|
||||
"text": [
|
||||
"{\n",
|
||||
" \"choices\": [\n",
|
||||
" {\n",
|
||||
" \"content_filter_results\": {},\n",
|
||||
" \"finish_reason\": \"function_call\",\n",
|
||||
" \"index\": 0,\n",
|
||||
" \"message\": {\n",
|
||||
" \"function_call\": {\n",
|
||||
" \"arguments\": \"{\\n \\\"location\\\": \\\"Seattle, WA\\\"\\n}\",\n",
|
||||
" \"name\": \"get_current_weather\"\n",
|
||||
" },\n",
|
||||
" \"role\": \"assistant\"\n",
|
||||
" }\n",
|
||||
" }\n",
|
||||
" ],\n",
|
||||
" \"created\": 1689702512,\n",
|
||||
" \"id\": \"chatcmpl-7dj6GkYdM7Vw9eGn02bc2qqjN70Ps\",\n",
|
||||
" \"model\": \"gpt-4\",\n",
|
||||
" \"object\": \"chat.completion\",\n",
|
||||
" \"prompt_annotations\": [\n",
|
||||
" {\n",
|
||||
" \"content_filter_results\": {\n",
|
||||
" \"hate\": {\n",
|
||||
" \"filtered\": false,\n",
|
||||
" \"severity\": \"safe\"\n",
|
||||
" },\n",
|
||||
" \"self_harm\": {\n",
|
||||
" \"filtered\": false,\n",
|
||||
" \"severity\": \"safe\"\n",
|
||||
" },\n",
|
||||
" \"sexual\": {\n",
|
||||
" \"filtered\": false,\n",
|
||||
" \"severity\": \"safe\"\n",
|
||||
" },\n",
|
||||
" \"violence\": {\n",
|
||||
" \"filtered\": false,\n",
|
||||
" \"severity\": \"safe\"\n",
|
||||
" }\n",
|
||||
" },\n",
|
||||
" \"prompt_index\": 0\n",
|
||||
" }\n",
|
||||
" ],\n",
|
||||
" \"usage\": {\n",
|
||||
" \"completion_tokens\": 18,\n",
|
||||
" \"prompt_tokens\": 115,\n",
|
||||
" \"total_tokens\": 133\n",
|
||||
" }\n",
|
||||
"}\n"
|
||||
]
|
||||
}
|
||||
],
|
||||
"source": [
|
||||
"messages = [\n",
|
||||
" {\"role\": \"system\", \"content\": \"Don't make assumptions about what values to plug into functions. Ask for clarification if a user request is ambiguous.\"},\n",
|
||||
" {\"role\": \"user\", \"content\": \"What's the weather like today in Seattle?\"}\n",
|
||||
"]\n",
|
||||
"\n",
|
||||
"chat_completion = openai.ChatCompletion.create(\n",
|
||||
" deployment_id=\"gpt-35-turbo-0613\",\n",
|
||||
" messages=messages,\n",
|
||||
" functions=functions,\n",
|
||||
")\n",
|
||||
"print(chat_completion)"
|
||||
]
|
||||
},
|
||||
{
|
||||
"attachments": {},
|
||||
"cell_type": "markdown",
|
||||
"metadata": {},
|
||||
"source": [
|
||||
"#### 3. Call function with arguments from the response\n",
|
||||
"\n",
|
||||
"The name of the function call will be one that was provided initially and the arguments will include JSON matching the schema included in the function definition."
|
||||
]
|
||||
},
|
||||
{
|
||||
"cell_type": "code",
|
||||
"execution_count": null,
|
||||
"metadata": {},
|
||||
"outputs": [
|
||||
{
|
||||
"name": "stdout",
|
||||
"output_type": "stream",
|
||||
"text": [
|
||||
"get_current_weather\n",
|
||||
"{\n",
|
||||
" \"location\": \"Seattle, WA\"\n",
|
||||
"}\n"
|
||||
]
|
||||
}
|
||||
],
|
||||
"source": [
|
||||
"import json\n",
|
||||
"\n",
|
||||
"def get_current_weather(request):\n",
|
||||
" \"\"\"\n",
|
||||
" This function is for illustrative purposes.\n",
|
||||
" The location and unit should be used to determine weather\n",
|
||||
" instead of returning a hardcoded response.\n",
|
||||
" \"\"\"\n",
|
||||
" location = request.get(\"location\")\n",
|
||||
" unit = request.get(\"unit\")\n",
|
||||
" return {\"temperature\": \"22\", \"unit\": \"celsius\", \"description\": \"Sunny\"}\n",
|
||||
"\n",
|
||||
"function_call = chat_completion.choices[0].message.function_call\n",
|
||||
"print(function_call.name)\n",
|
||||
"print(function_call.arguments)\n",
|
||||
"\n",
|
||||
"if function_call.name == \"get_current_weather\":\n",
|
||||
" response = get_current_weather(json.loads(function_call.arguments))"
|
||||
]
|
||||
},
|
||||
{
|
||||
"attachments": {},
|
||||
"cell_type": "markdown",
|
||||
"metadata": {},
|
||||
"source": [
|
||||
"#### 4. Feed function response back into chat completions API\n",
|
||||
"\n",
|
||||
"The response from the function should be serialized into a new message with the role set to \"function\". Now the model will use the response data to formulate its answer."
|
||||
]
|
||||
},
|
||||
{
|
||||
"cell_type": "code",
|
||||
"execution_count": null,
|
||||
"metadata": {},
|
||||
"outputs": [
|
||||
{
|
||||
"name": "stdout",
|
||||
"output_type": "stream",
|
||||
"text": [
|
||||
"Today in Seattle, the weather is sunny with a temperature of 22 degrees celsius.\n"
|
||||
]
|
||||
}
|
||||
],
|
||||
"source": [
|
||||
"messages.append(\n",
|
||||
" {\n",
|
||||
" \"role\": \"function\",\n",
|
||||
" \"name\": \"get_current_weather\",\n",
|
||||
" \"content\": json.dumps(response)\n",
|
||||
" }\n",
|
||||
")\n",
|
||||
"\n",
|
||||
"function_completion = openai.ChatCompletion.create(\n",
|
||||
" deployment_id=\"gpt-35-turbo-0613\",\n",
|
||||
" messages=messages,\n",
|
||||
" functions=functions,\n",
|
||||
")\n",
|
||||
"\n",
|
||||
"print(function_completion.choices[0].message.content.strip())"
|
||||
]
|
||||
}
|
||||
],
|
||||
"metadata": {
|
||||
"kernelspec": {
|
||||
"display_name": "Python 3 (ipykernel)",
|
||||
"language": "python",
|
||||
"name": "python3"
|
||||
},
|
||||
"language_info": {
|
||||
"codemirror_mode": {
|
||||
"name": "ipython",
|
||||
"version": 3
|
||||
},
|
||||
"file_extension": ".py",
|
||||
"mimetype": "text/x-python",
|
||||
"name": "python",
|
||||
"nbconvert_exporter": "python",
|
||||
"pygments_lexer": "ipython3",
|
||||
"version": "3.10.0"
|
||||
},
|
||||
"vscode": {
|
||||
"interpreter": {
|
||||
"hash": "3a5103089ab7e7c666b279eeded403fcec76de49a40685dbdfe9f9c78ad97c17"
|
||||
}
|
||||
}
|
||||
},
|
||||
"nbformat": 4,
|
||||
"nbformat_minor": 2
|
||||
}
|
@ -0,0 +1,276 @@
|
||||
{
|
||||
"cells": [
|
||||
{
|
||||
"cell_type": "markdown",
|
||||
"metadata": {},
|
||||
"source": [
|
||||
"# Azure audio whisper (preview) example\n",
|
||||
"\n",
|
||||
"> Note: There is a newer version of the openai library available. See https://github.com/openai/openai-python/discussions/742\n",
|
||||
"\n",
|
||||
"The example shows how to use the Azure OpenAI Whisper model to transcribe audio files."
|
||||
]
|
||||
},
|
||||
{
|
||||
"cell_type": "markdown",
|
||||
"metadata": {},
|
||||
"source": [
|
||||
"## Setup\n",
|
||||
"\n",
|
||||
"First, we install the necessary dependencies."
|
||||
]
|
||||
},
|
||||
{
|
||||
"cell_type": "code",
|
||||
"execution_count": null,
|
||||
"metadata": {},
|
||||
"outputs": [],
|
||||
"source": [
|
||||
"! pip install \"openai>=0.28.1,<1.0.0\"\n",
|
||||
"! pip install python-dotenv"
|
||||
]
|
||||
},
|
||||
{
|
||||
"cell_type": "markdown",
|
||||
"metadata": {},
|
||||
"source": [
|
||||
"Next, we'll import our libraries and configure the Python OpenAI SDK to work with the Azure OpenAI service."
|
||||
]
|
||||
},
|
||||
{
|
||||
"cell_type": "markdown",
|
||||
"metadata": {},
|
||||
"source": [
|
||||
"> Note: In this example, we configured the library to use the Azure API by setting the variables in code. For development, consider setting the environment variables instead:\n",
|
||||
"\n",
|
||||
"```\n",
|
||||
"OPENAI_API_BASE\n",
|
||||
"OPENAI_API_KEY\n",
|
||||
"OPENAI_API_TYPE\n",
|
||||
"OPENAI_API_VERSION\n",
|
||||
"```"
|
||||
]
|
||||
},
|
||||
{
|
||||
"cell_type": "code",
|
||||
"execution_count": 1,
|
||||
"metadata": {},
|
||||
"outputs": [
|
||||
{
|
||||
"data": {
|
||||
"text/plain": [
|
||||
"True"
|
||||
]
|
||||
},
|
||||
"execution_count": 1,
|
||||
"metadata": {},
|
||||
"output_type": "execute_result"
|
||||
}
|
||||
],
|
||||
"source": [
|
||||
"import os\n",
|
||||
"import dotenv\n",
|
||||
"import openai\n",
|
||||
"\n",
|
||||
"\n",
|
||||
"dotenv.load_dotenv()"
|
||||
]
|
||||
},
|
||||
{
|
||||
"attachments": {},
|
||||
"cell_type": "markdown",
|
||||
"metadata": {},
|
||||
"source": [
|
||||
"\n",
|
||||
"To properly access the Azure OpenAI Service, we need to create the proper resources at the [Azure Portal](https://portal.azure.com) (you can check a detailed guide on how to do this in the [Microsoft Docs](https://learn.microsoft.com/en-us/azure/cognitive-services/openai/how-to/create-resource?pivots=web-portal))\n",
|
||||
"\n",
|
||||
"Once the resource is created, the first thing we need to use is its endpoint. You can get the endpoint by looking at the *\"Keys and Endpoints\"* section under the *\"Resource Management\"* section. Having this, we will set up the SDK using this information:"
|
||||
]
|
||||
},
|
||||
{
|
||||
"cell_type": "code",
|
||||
"execution_count": 2,
|
||||
"metadata": {},
|
||||
"outputs": [],
|
||||
"source": [
|
||||
"openai.api_base = os.environ[\"OPENAI_API_BASE\"]\n",
|
||||
"\n",
|
||||
"# Min API version that supports Whisper\n",
|
||||
"openai.api_version = \"2023-09-01-preview\"\n",
|
||||
"\n",
|
||||
"# Enter the deployment_id to use for the Whisper model\n",
|
||||
"deployment_id = \"<deployment-id-for-your-whisper-model>\""
|
||||
]
|
||||
},
|
||||
{
|
||||
"attachments": {},
|
||||
"cell_type": "markdown",
|
||||
"metadata": {},
|
||||
"source": [
|
||||
"### Authentication\n",
|
||||
"\n",
|
||||
"The Azure OpenAI service supports multiple authentication mechanisms that include API keys and Azure credentials."
|
||||
]
|
||||
},
|
||||
{
|
||||
"cell_type": "code",
|
||||
"execution_count": 3,
|
||||
"metadata": {},
|
||||
"outputs": [],
|
||||
"source": [
|
||||
"# set to True if using Azure Active Directory authentication\n",
|
||||
"use_azure_active_directory = False"
|
||||
]
|
||||
},
|
||||
{
|
||||
"attachments": {},
|
||||
"cell_type": "markdown",
|
||||
"metadata": {},
|
||||
"source": [
|
||||
"\n",
|
||||
"#### Authentication using API key\n",
|
||||
"\n",
|
||||
"To set up the OpenAI SDK to use an *Azure API Key*, we need to set up the `api_type` to `azure` and set `api_key` to a key associated with your endpoint (you can find this key in *\"Keys and Endpoints\"* under *\"Resource Management\"* in the [Azure Portal](https://portal.azure.com))"
|
||||
]
|
||||
},
|
||||
{
|
||||
"cell_type": "code",
|
||||
"execution_count": 4,
|
||||
"metadata": {},
|
||||
"outputs": [],
|
||||
"source": [
|
||||
"if not use_azure_active_directory:\n",
|
||||
" openai.api_type = 'azure'\n",
|
||||
" openai.api_key = os.environ[\"OPENAI_API_KEY\"]"
|
||||
]
|
||||
},
|
||||
{
|
||||
"attachments": {},
|
||||
"cell_type": "markdown",
|
||||
"metadata": {},
|
||||
"source": [
|
||||
"#### Authentication using Azure Active Directory\n",
|
||||
"Let's now see how we can get a key via Microsoft Active Directory Authentication."
|
||||
]
|
||||
},
|
||||
{
|
||||
"cell_type": "code",
|
||||
"execution_count": null,
|
||||
"metadata": {},
|
||||
"outputs": [],
|
||||
"source": [
|
||||
"from azure.identity import DefaultAzureCredential\n",
|
||||
"\n",
|
||||
"if use_azure_active_directory:\n",
|
||||
" default_credential = DefaultAzureCredential()\n",
|
||||
" token = default_credential.get_token(\"https://cognitiveservices.azure.com/.default\")\n",
|
||||
"\n",
|
||||
" openai.api_type = 'azure_ad'\n",
|
||||
" openai.api_key = token.token"
|
||||
]
|
||||
},
|
||||
{
|
||||
"attachments": {},
|
||||
"cell_type": "markdown",
|
||||
"metadata": {},
|
||||
"source": [
|
||||
"A token is valid for a period of time, after which it will expire. To ensure a valid token is sent with every request, you can refresh an expiring token by hooking into requests.auth:"
|
||||
]
|
||||
},
|
||||
{
|
||||
"cell_type": "code",
|
||||
"execution_count": null,
|
||||
"metadata": {},
|
||||
"outputs": [],
|
||||
"source": [
|
||||
"import typing\n",
|
||||
"import time\n",
|
||||
"import requests\n",
|
||||
"\n",
|
||||
"if typing.TYPE_CHECKING:\n",
|
||||
" from azure.core.credentials import TokenCredential\n",
|
||||
"\n",
|
||||
"class TokenRefresh(requests.auth.AuthBase):\n",
|
||||
"\n",
|
||||
" def __init__(self, credential: \"TokenCredential\", scopes: typing.List[str]) -> None:\n",
|
||||
" self.credential = credential\n",
|
||||
" self.scopes = scopes\n",
|
||||
" self.cached_token: typing.Optional[str] = None\n",
|
||||
"\n",
|
||||
" def __call__(self, req):\n",
|
||||
" if not self.cached_token or self.cached_token.expires_on - time.time() < 300:\n",
|
||||
" self.cached_token = self.credential.get_token(*self.scopes)\n",
|
||||
" req.headers[\"Authorization\"] = f\"Bearer {self.cached_token.token}\"\n",
|
||||
" return req\n",
|
||||
"\n",
|
||||
"if use_azure_active_directory:\n",
|
||||
" session = requests.Session()\n",
|
||||
" session.auth = TokenRefresh(default_credential, [\"https://cognitiveservices.azure.com/.default\"])\n",
|
||||
"\n",
|
||||
" openai.requestssession = session"
|
||||
]
|
||||
},
|
||||
{
|
||||
"cell_type": "markdown",
|
||||
"metadata": {},
|
||||
"source": [
|
||||
"## Audio transcription\n",
|
||||
"\n",
|
||||
"Audio transcription, or speech-to-text, is the process of converting spoken words into text. Use the `openai.Audio.transcribe` method to transcribe an audio file stream to text.\n",
|
||||
"\n",
|
||||
"You can get sample audio files from the [Azure AI Speech SDK repository at GitHub](https://github.com/Azure-Samples/cognitive-services-speech-sdk/tree/master/sampledata/audiofiles)."
|
||||
]
|
||||
},
|
||||
{
|
||||
"cell_type": "code",
|
||||
"execution_count": 9,
|
||||
"metadata": {},
|
||||
"outputs": [],
|
||||
"source": [
|
||||
"# download sample audio file\n",
|
||||
"import requests\n",
|
||||
"\n",
|
||||
"sample_audio_url = \"https://github.com/Azure-Samples/cognitive-services-speech-sdk/raw/master/sampledata/audiofiles/wikipediaOcelot.wav\"\n",
|
||||
"audio_file = requests.get(sample_audio_url)\n",
|
||||
"with open(\"wikipediaOcelot.wav\", \"wb\") as f:\n",
|
||||
" f.write(audio_file.content)"
|
||||
]
|
||||
},
|
||||
{
|
||||
"cell_type": "code",
|
||||
"execution_count": null,
|
||||
"metadata": {},
|
||||
"outputs": [],
|
||||
"source": [
|
||||
"transcription = openai.Audio.transcribe(\n",
|
||||
" file=open(\"wikipediaOcelot.wav\", \"rb\"),\n",
|
||||
" model=\"whisper-1\",\n",
|
||||
" deployment_id=deployment_id,\n",
|
||||
")\n",
|
||||
"print(transcription.text)"
|
||||
]
|
||||
}
|
||||
],
|
||||
"metadata": {
|
||||
"kernelspec": {
|
||||
"display_name": "venv",
|
||||
"language": "python",
|
||||
"name": "python3"
|
||||
},
|
||||
"language_info": {
|
||||
"codemirror_mode": {
|
||||
"name": "ipython",
|
||||
"version": 3
|
||||
},
|
||||
"file_extension": ".py",
|
||||
"mimetype": "text/x-python",
|
||||
"name": "python",
|
||||
"nbconvert_exporter": "python",
|
||||
"pygments_lexer": "ipython3",
|
||||
"version": "3.10.0"
|
||||
},
|
||||
"orig_nbformat": 4
|
||||
},
|
||||
"nbformat": 4,
|
||||
"nbformat_minor": 2
|
||||
}
|
File diff suppressed because one or more lines are too long
@ -0,0 +1,228 @@
|
||||
{
|
||||
"cells": [
|
||||
{
|
||||
"attachments": {},
|
||||
"cell_type": "markdown",
|
||||
"metadata": {},
|
||||
"source": [
|
||||
"# Azure audio whisper (preview) example\n",
|
||||
"\n",
|
||||
"The example shows how to use the Azure OpenAI Whisper model to transcribe audio files.\n"
|
||||
]
|
||||
},
|
||||
{
|
||||
"cell_type": "markdown",
|
||||
"metadata": {},
|
||||
"source": [
|
||||
"## Setup\n",
|
||||
"\n",
|
||||
"First, we install the necessary dependencies and import the libraries we will be using."
|
||||
]
|
||||
},
|
||||
{
|
||||
"cell_type": "code",
|
||||
"execution_count": null,
|
||||
"metadata": {},
|
||||
"outputs": [],
|
||||
"source": [
|
||||
"! pip install \"openai>=1.0.0,<2.0.0\"\n",
|
||||
"! pip install python-dotenv"
|
||||
]
|
||||
},
|
||||
{
|
||||
"cell_type": "code",
|
||||
"execution_count": null,
|
||||
"metadata": {},
|
||||
"outputs": [],
|
||||
"source": [
|
||||
"import os\n",
|
||||
"import openai\n",
|
||||
"import dotenv\n",
|
||||
"\n",
|
||||
"dotenv.load_dotenv()"
|
||||
]
|
||||
},
|
||||
{
|
||||
"cell_type": "markdown",
|
||||
"metadata": {},
|
||||
"source": [
|
||||
"### Authentication\n",
|
||||
"\n",
|
||||
"The Azure OpenAI service supports multiple authentication mechanisms that include API keys and Azure Active Directory token credentials."
|
||||
]
|
||||
},
|
||||
{
|
||||
"cell_type": "code",
|
||||
"execution_count": 4,
|
||||
"metadata": {},
|
||||
"outputs": [],
|
||||
"source": [
|
||||
"use_azure_active_directory = False # Set this flag to True if you are using Azure Active Directory"
|
||||
]
|
||||
},
|
||||
{
|
||||
"cell_type": "markdown",
|
||||
"metadata": {},
|
||||
"source": [
|
||||
"#### Authentication using API key\n",
|
||||
"\n",
|
||||
"To set up the OpenAI SDK to use an *Azure API Key*, we need to set `api_key` to a key associated with your endpoint (you can find this key in *\"Keys and Endpoints\"* under *\"Resource Management\"* in the [Azure Portal](https://portal.azure.com)). You'll also find the endpoint for your resource here."
|
||||
]
|
||||
},
|
||||
{
|
||||
"cell_type": "code",
|
||||
"execution_count": 5,
|
||||
"metadata": {},
|
||||
"outputs": [],
|
||||
"source": [
|
||||
"if not use_azure_active_directory:\n",
|
||||
" endpoint = os.environ[\"AZURE_OPENAI_ENDPOINT\"]\n",
|
||||
" api_key = os.environ[\"AZURE_OPENAI_API_KEY\"]\n",
|
||||
"\n",
|
||||
" client = openai.AzureOpenAI(\n",
|
||||
" azure_endpoint=endpoint,\n",
|
||||
" api_key=api_key,\n",
|
||||
" api_version=\"2023-09-01-preview\"\n",
|
||||
" )"
|
||||
]
|
||||
},
|
||||
{
|
||||
"cell_type": "markdown",
|
||||
"metadata": {},
|
||||
"source": [
|
||||
"#### Authentication using Azure Active Directory\n",
|
||||
"Let's now see how we can autheticate via Azure Active Directory. We'll start by installing the `azure-identity` library. This library will provide the token credentials we need to authenticate and help us build a token credential provider through the `get_bearer_token_provider` helper function. It's recommended to use `get_bearer_token_provider` over providing a static token to `AzureOpenAI` because this API will automatically cache and refresh tokens for you. \n",
|
||||
"\n",
|
||||
"For more information on how to set up Azure Active Directory authentication with Azure OpenAI, see the [documentation](https://learn.microsoft.com/azure/ai-services/openai/how-to/managed-identity)."
|
||||
]
|
||||
},
|
||||
{
|
||||
"cell_type": "code",
|
||||
"execution_count": null,
|
||||
"metadata": {},
|
||||
"outputs": [],
|
||||
"source": [
|
||||
"! pip install \"azure-identity>=1.15.0\""
|
||||
]
|
||||
},
|
||||
{
|
||||
"cell_type": "code",
|
||||
"execution_count": 5,
|
||||
"metadata": {},
|
||||
"outputs": [],
|
||||
"source": [
|
||||
"from azure.identity import DefaultAzureCredential, get_bearer_token_provider\n",
|
||||
"\n",
|
||||
"if use_azure_active_directory:\n",
|
||||
" endpoint = os.environ[\"AZURE_OPENAI_ENDPOINT\"]\n",
|
||||
" api_key = os.environ[\"AZURE_OPENAI_API_KEY\"]\n",
|
||||
"\n",
|
||||
" client = openai.AzureOpenAI(\n",
|
||||
" azure_endpoint=endpoint,\n",
|
||||
" azure_ad_token_provider=get_bearer_token_provider(DefaultAzureCredential(), \"https://cognitiveservices.azure.com/.default\"),\n",
|
||||
" api_version=\"2023-09-01-preview\"\n",
|
||||
" )"
|
||||
]
|
||||
},
|
||||
{
|
||||
"cell_type": "markdown",
|
||||
"metadata": {},
|
||||
"source": [
|
||||
"> Note: the AzureOpenAI infers the following arguments from their corresponding environment variables if they are not provided:\n",
|
||||
"\n",
|
||||
"- `api_key` from `AZURE_OPENAI_API_KEY`\n",
|
||||
"- `azure_ad_token` from `AZURE_OPENAI_AD_TOKEN`\n",
|
||||
"- `api_version` from `OPENAI_API_VERSION`\n",
|
||||
"- `azure_endpoint` from `AZURE_OPENAI_ENDPOINT`\n"
|
||||
]
|
||||
},
|
||||
{
|
||||
"attachments": {},
|
||||
"cell_type": "markdown",
|
||||
"metadata": {},
|
||||
"source": [
|
||||
"## Deployments\n",
|
||||
"\n",
|
||||
"In this section we are going to create a deployment using the `whisper-1` model to transcribe audio files."
|
||||
]
|
||||
},
|
||||
{
|
||||
"cell_type": "markdown",
|
||||
"metadata": {},
|
||||
"source": [
|
||||
"### Deployments: Create in the Azure OpenAI Studio\n",
|
||||
"Let's deploy a model to use with whisper. Go to https://portal.azure.com, find your Azure OpenAI resource, and then navigate to the Azure OpenAI Studio. Click on the \"Deployments\" tab and then create a deployment for the model you want to use for whisper. The deployment name that you give the model will be used in the code below."
|
||||
]
|
||||
},
|
||||
{
|
||||
"cell_type": "code",
|
||||
"execution_count": 6,
|
||||
"metadata": {},
|
||||
"outputs": [],
|
||||
"source": [
|
||||
"deployment = \"whisper-deployment\" # Fill in the deployment name from the portal here"
|
||||
]
|
||||
},
|
||||
{
|
||||
"cell_type": "markdown",
|
||||
"metadata": {},
|
||||
"source": [
|
||||
"## Audio transcription\n",
|
||||
"\n",
|
||||
"Audio transcription, or speech-to-text, is the process of converting spoken words into text. Use the `openai.Audio.transcribe` method to transcribe an audio file stream to text.\n",
|
||||
"\n",
|
||||
"You can get sample audio files from the [Azure AI Speech SDK repository at GitHub](https://github.com/Azure-Samples/cognitive-services-speech-sdk/tree/master/sampledata/audiofiles)."
|
||||
]
|
||||
},
|
||||
{
|
||||
"cell_type": "code",
|
||||
"execution_count": 7,
|
||||
"metadata": {},
|
||||
"outputs": [],
|
||||
"source": [
|
||||
"# download sample audio file\n",
|
||||
"import requests\n",
|
||||
"\n",
|
||||
"sample_audio_url = \"https://github.com/Azure-Samples/cognitive-services-speech-sdk/raw/master/sampledata/audiofiles/wikipediaOcelot.wav\"\n",
|
||||
"audio_file = requests.get(sample_audio_url)\n",
|
||||
"with open(\"wikipediaOcelot.wav\", \"wb\") as f:\n",
|
||||
" f.write(audio_file.content)"
|
||||
]
|
||||
},
|
||||
{
|
||||
"cell_type": "code",
|
||||
"execution_count": null,
|
||||
"metadata": {},
|
||||
"outputs": [],
|
||||
"source": [
|
||||
"transcription = client.audio.transcriptions.create(\n",
|
||||
" file=open(\"wikipediaOcelot.wav\", \"rb\"),\n",
|
||||
" model=deployment,\n",
|
||||
")\n",
|
||||
"print(transcription.text)"
|
||||
]
|
||||
}
|
||||
],
|
||||
"metadata": {
|
||||
"kernelspec": {
|
||||
"display_name": "venv",
|
||||
"language": "python",
|
||||
"name": "python3"
|
||||
},
|
||||
"language_info": {
|
||||
"codemirror_mode": {
|
||||
"name": "ipython",
|
||||
"version": 3
|
||||
},
|
||||
"file_extension": ".py",
|
||||
"mimetype": "text/x-python",
|
||||
"name": "python",
|
||||
"nbconvert_exporter": "python",
|
||||
"pygments_lexer": "ipython3",
|
||||
"version": "3.10.0"
|
||||
},
|
||||
"orig_nbformat": 4
|
||||
},
|
||||
"nbformat": 4,
|
||||
"nbformat_minor": 2
|
||||
}
|
@ -0,0 +1,885 @@
|
||||
{
|
||||
"cells": [
|
||||
{
|
||||
"cell_type": "markdown",
|
||||
"id": "46589cdf-1ab6-4028-b07c-08b75acd98e5",
|
||||
"metadata": {},
|
||||
"source": [
|
||||
"# Philosophy with Vector Embeddings, OpenAI and Astra DB\n",
|
||||
"\n",
|
||||
"### AstraPy version"
|
||||
]
|
||||
},
|
||||
{
|
||||
"cell_type": "markdown",
|
||||
"id": "b3496d07-f473-4008-9133-1a54b818c8d3",
|
||||
"metadata": {},
|
||||
"source": [
|
||||
"In this quickstart you will learn how to build a \"philosophy quote finder & generator\" using OpenAI's vector embeddings and DataStax [Astra DB](https://docs.datastax.com/en/astra/home/astra.html) as the vector store for data persistence.\n",
|
||||
"\n",
|
||||
"The basic workflow of this notebook is outlined below. You will evaluate and store the vector embeddings for a number of quotes by famous philosophers, use them to build a powerful search engine and, after that, even a generator of new quotes!\n",
|
||||
"\n",
|
||||
"The notebook exemplifies some of the standard usage patterns of vector search -- while showing how easy is it to get started with [Astra DB](https://docs.datastax.com/en/astra/home/astra.html).\n",
|
||||
"\n",
|
||||
"For a background on using vector search and text embeddings to build a question-answering system, please check out this excellent hands-on notebook: [Question answering using embeddings](https://github.com/openai/openai-cookbook/blob/main/examples/Question_answering_using_embeddings.ipynb).\n",
|
||||
"\n",
|
||||
"Table of contents:\n",
|
||||
"- Setup\n",
|
||||
"- Create vector collection\n",
|
||||
"- Connect to OpenAI\n",
|
||||
"- Load quotes into the Vector Store\n",
|
||||
"- Use case 1: **quote search engine**\n",
|
||||
"- Use case 2: **quote generator**\n",
|
||||
"- Cleanup"
|
||||
]
|
||||
},
|
||||
{
|
||||
"cell_type": "markdown",
|
||||
"id": "cddf17cc-eef4-4021-b72a-4d3832a9b4a7",
|
||||
"metadata": {},
|
||||
"source": [
|
||||
"### How it works\n",
|
||||
"\n",
|
||||
"**Indexing**\n",
|
||||
"\n",
|
||||
"Each quote is made into an embedding vector with OpenAI's `Embedding`. These are saved in the Vector Store for later use in searching. Some metadata, including the author's name and a few other pre-computed tags, are stored alongside, to allow for search customization.\n",
|
||||
"\n",
|
||||
"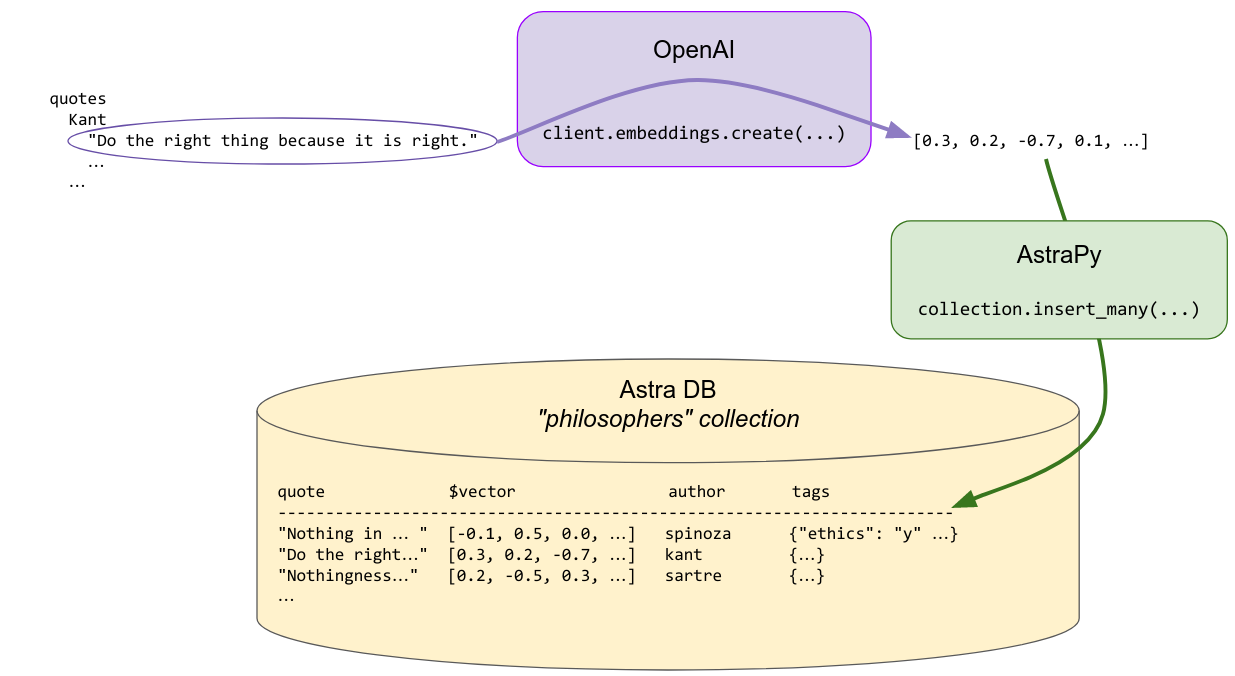\n",
|
||||
"\n",
|
||||
"**Search**\n",
|
||||
"\n",
|
||||
"To find a quote similar to the provided search quote, the latter is made into an embedding vector on the fly, and this vector is used to query the store for similar vectors ... i.e. similar quotes that were previously indexed. The search can optionally be constrained by additional metadata (\"find me quotes by Spinoza similar to this one ...\").\n",
|
||||
"\n",
|
||||
"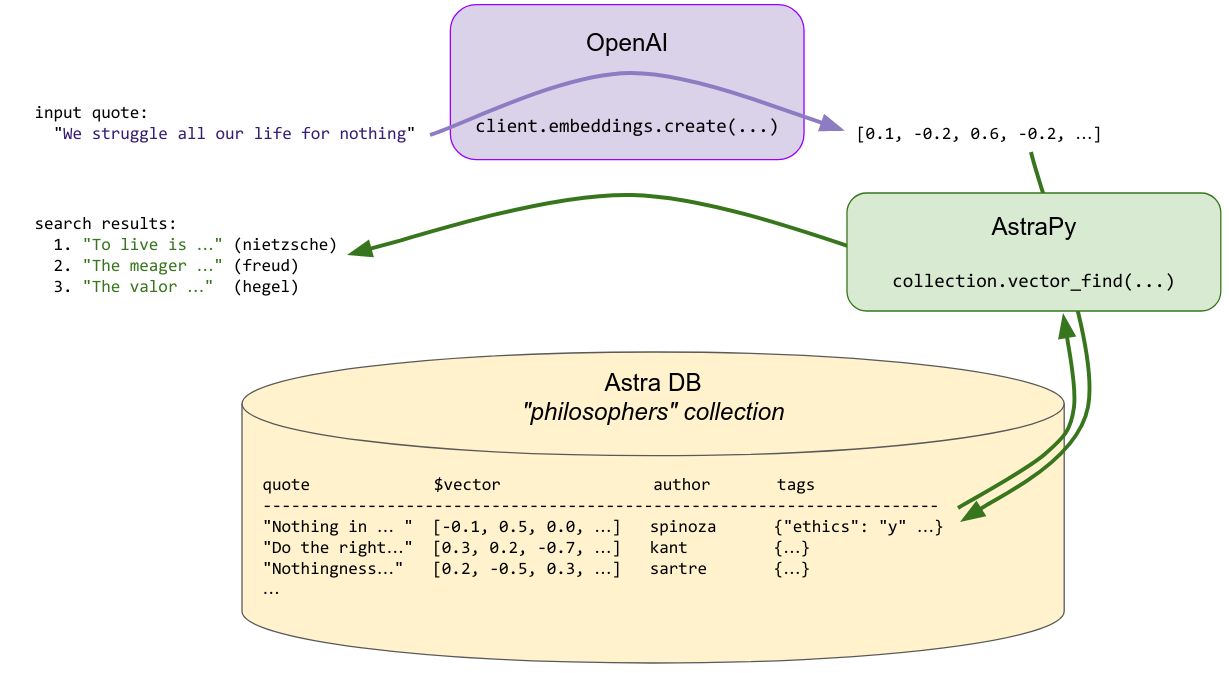\n",
|
||||
"\n",
|
||||
"The key point here is that \"quotes similar in content\" translates, in vector space, to vectors that are metrically close to each other: thus, vector similarity search effectively implements semantic similarity. _This is the key reason vector embeddings are so powerful._\n",
|
||||
"\n",
|
||||
"The sketch below tries to convey this idea. Each quote, once it's made into a vector, is a point in space. Well, in this case it's on a sphere, since OpenAI's embedding vectors, as most others, are normalized to _unit length_. Oh, and the sphere is actually not three-dimensional, rather 1536-dimensional!\n",
|
||||
"\n",
|
||||
"So, in essence, a similarity search in vector space returns the vectors that are closest to the query vector:\n",
|
||||
"\n",
|
||||
"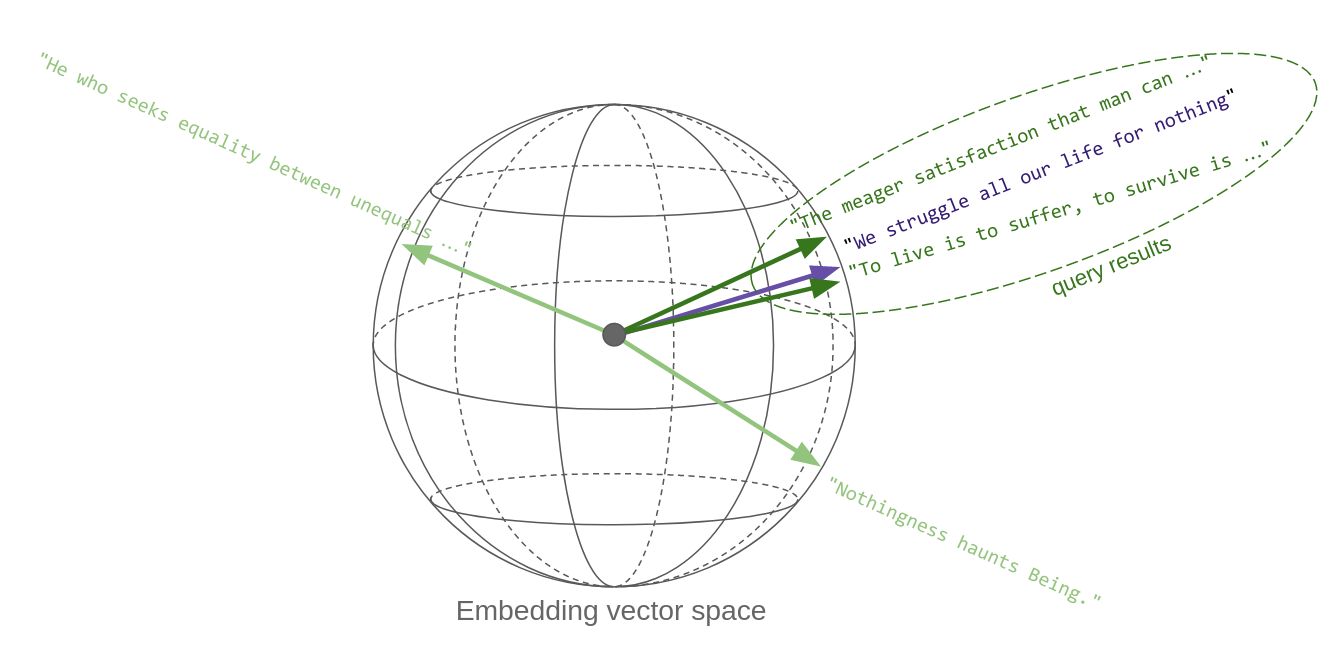\n",
|
||||
"\n",
|
||||
"**Generation**\n",
|
||||
"\n",
|
||||
"Given a suggestion (a topic or a tentative quote), the search step is performed, and the first returned results (quotes) are fed into an LLM prompt which asks the generative model to invent a new text along the lines of the passed examples _and_ the initial suggestion.\n",
|
||||
"\n",
|
||||
"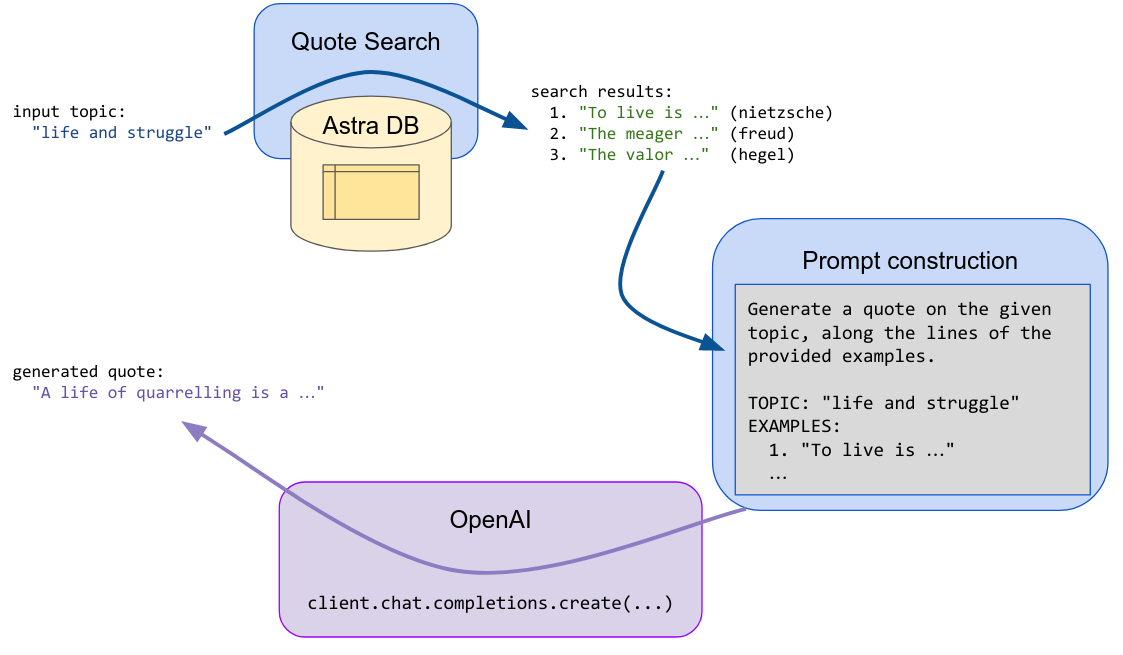"
|
||||
]
|
||||
},
|
||||
{
|
||||
"cell_type": "markdown",
|
||||
"id": "10493f44-565d-4f23-8bfd-1a7335392c2b",
|
||||
"metadata": {},
|
||||
"source": [
|
||||
"## Setup"
|
||||
]
|
||||
},
|
||||
{
|
||||
"cell_type": "markdown",
|
||||
"id": "44a14f95-4683-4d0c-a251-0df7b43ca975",
|
||||
"metadata": {},
|
||||
"source": [
|
||||
"Install and import the necessary dependencies:"
|
||||
]
|
||||
},
|
||||
{
|
||||
"cell_type": "code",
|
||||
"execution_count": 1,
|
||||
"id": "39afdb74-56e4-44ff-9c72-ab2669780113",
|
||||
"metadata": {
|
||||
"scrolled": true
|
||||
},
|
||||
"outputs": [],
|
||||
"source": [
|
||||
"!pip install --quiet \"astrapy>=0.6.0\" \"openai>=1.0.0\" datasets"
|
||||
]
|
||||
},
|
||||
{
|
||||
"cell_type": "code",
|
||||
"execution_count": 2,
|
||||
"id": "9ca6f5c6-30b4-4518-a816-5c732a60e339",
|
||||
"metadata": {},
|
||||
"outputs": [],
|
||||
"source": [
|
||||
"from getpass import getpass\n",
|
||||
"from collections import Counter\n",
|
||||
"\n",
|
||||
"from astrapy.db import AstraDB\n",
|
||||
"import openai\n",
|
||||
"from datasets import load_dataset"
|
||||
]
|
||||
},
|
||||
{
|
||||
"cell_type": "markdown",
|
||||
"id": "9cb99e33-5cb7-416f-8dca-da18e0cb108d",
|
||||
"metadata": {},
|
||||
"source": [
|
||||
"### Connection parameters"
|
||||
]
|
||||
},
|
||||
{
|
||||
"cell_type": "markdown",
|
||||
"id": "65a8edc1-4633-491b-9ed3-11163ec24e46",
|
||||
"metadata": {},
|
||||
"source": [
|
||||
"Please retrieve your database credentials on your Astra dashboard ([info](https://docs.datastax.com/en/astra/astra-db-vector/)): you will supply them momentarily.\n",
|
||||
"\n",
|
||||
"Example values:\n",
|
||||
"\n",
|
||||
"- API Endpoint: `https://01234567-89ab-cdef-0123-456789abcdef-us-east1.apps.astra.datastax.com`\n",
|
||||
"- Token: `AstraCS:6gBhNmsk135...`"
|
||||
]
|
||||
},
|
||||
{
|
||||
"cell_type": "code",
|
||||
"execution_count": 3,
|
||||
"id": "ca5a2f5d-3ff2-43d6-91c0-4a52c0ecd06a",
|
||||
"metadata": {},
|
||||
"outputs": [
|
||||
{
|
||||
"name": "stdin",
|
||||
"output_type": "stream",
|
||||
"text": [
|
||||
"Please enter your API Endpoint: https://4f835778-ec78-42b0-9ae3-29e3cf45b596-us-east1.apps.astra.datastax.com\n",
|
||||
"Please enter your Token ········\n"
|
||||
]
|
||||
}
|
||||
],
|
||||
"source": [
|
||||
"ASTRA_DB_API_ENDPOINT = input(\"Please enter your API Endpoint:\")\n",
|
||||
"ASTRA_DB_APPLICATION_TOKEN = getpass(\"Please enter your Token\")"
|
||||
]
|
||||
},
|
||||
{
|
||||
"cell_type": "markdown",
|
||||
"id": "f8c4e5ec-2ab2-4d41-b3ec-c946469fed8b",
|
||||
"metadata": {},
|
||||
"source": [
|
||||
"### Instantiate an Astra DB client"
|
||||
]
|
||||
},
|
||||
{
|
||||
"cell_type": "code",
|
||||
"execution_count": 4,
|
||||
"id": "1b526e55-ad2c-413d-94b1-cf651afefd02",
|
||||
"metadata": {},
|
||||
"outputs": [],
|
||||
"source": [
|
||||
"astra_db = AstraDB(\n",
|
||||
" api_endpoint=ASTRA_DB_API_ENDPOINT,\n",
|
||||
" token=ASTRA_DB_APPLICATION_TOKEN,\n",
|
||||
")"
|
||||
]
|
||||
},
|
||||
{
|
||||
"cell_type": "markdown",
|
||||
"id": "60829851-bd48-4461-9243-974f76304933",
|
||||
"metadata": {},
|
||||
"source": [
|
||||
"## Create vector collection"
|
||||
]
|
||||
},
|
||||
{
|
||||
"cell_type": "markdown",
|
||||
"id": "cbcd19dc-0580-42c2-8d45-1cef52050a59",
|
||||
"metadata": {},
|
||||
"source": [
|
||||
"The only parameter to specify, other than the collection name, is the dimension of the vectors you'll store. Other parameters, notably the similarity metric to use for searches, are optional."
|
||||
]
|
||||
},
|
||||
{
|
||||
"cell_type": "code",
|
||||
"execution_count": 5,
|
||||
"id": "8db837dc-cd49-41e2-8b5d-edb17ccc470e",
|
||||
"metadata": {},
|
||||
"outputs": [],
|
||||
"source": [
|
||||
"coll_name = \"philosophers_astra_db\"\n",
|
||||
"collection = astra_db.create_collection(coll_name, dimension=1536)"
|
||||
]
|
||||
},
|
||||
{
|
||||
"cell_type": "markdown",
|
||||
"id": "da86f91a-88a6-4997-b0f8-9da0816f8ece",
|
||||
"metadata": {},
|
||||
"source": [
|
||||
"## Connect to OpenAI"
|
||||
]
|
||||
},
|
||||
{
|
||||
"cell_type": "markdown",
|
||||
"id": "a6b664b5-fd84-492e-a7bd-4dda3863b48a",
|
||||
"metadata": {},
|
||||
"source": [
|
||||
"### Set up your secret key"
|
||||
]
|
||||
},
|
||||
{
|
||||
"cell_type": "code",
|
||||
"execution_count": 6,
|
||||
"id": "37fe7653-dd64-4494-83e1-5702ec41725c",
|
||||
"metadata": {},
|
||||
"outputs": [
|
||||
{
|
||||
"name": "stdin",
|
||||
"output_type": "stream",
|
||||
"text": [
|
||||
"Please enter your OpenAI API Key: ········\n"
|
||||
]
|
||||
}
|
||||
],
|
||||
"source": [
|
||||
"OPENAI_API_KEY = getpass(\"Please enter your OpenAI API Key: \")"
|
||||
]
|
||||
},
|
||||
{
|
||||
"cell_type": "markdown",
|
||||
"id": "847f2821-7f3f-4dcd-8e0c-49aa397e36f4",
|
||||
"metadata": {},
|
||||
"source": [
|
||||
"### A test call for embeddings\n",
|
||||
"\n",
|
||||
"Quickly check how one can get the embedding vectors for a list of input texts:"
|
||||
]
|
||||
},
|
||||
{
|
||||
"cell_type": "code",
|
||||
"execution_count": 7,
|
||||
"id": "6bf89454-9a55-4202-ab6b-ea15b2048f3d",
|
||||
"metadata": {},
|
||||
"outputs": [],
|
||||
"source": [
|
||||
"client = openai.OpenAI(api_key=OPENAI_API_KEY)\n",
|
||||
"embedding_model_name = \"text-embedding-ada-002\"\n",
|
||||
"\n",
|
||||
"result = client.embeddings.create(\n",
|
||||
" input=[\n",
|
||||
" \"This is a sentence\",\n",
|
||||
" \"A second sentence\"\n",
|
||||
" ],\n",
|
||||
" model=embedding_model_name,\n",
|
||||
")"
|
||||
]
|
||||
},
|
||||
{
|
||||
"cell_type": "markdown",
|
||||
"id": "e2841934-7b2a-4a00-b112-b0865c9ec593",
|
||||
"metadata": {},
|
||||
"source": [
|
||||
"_Note: the above is the syntax for OpenAI v1.0+. If using previous versions, the code to get the embeddings will look different._"
|
||||
]
|
||||
},
|
||||
{
|
||||
"cell_type": "code",
|
||||
"execution_count": 8,
|
||||
"id": "50a8e6f0-0aa7-4ffc-94e9-702b68566815",
|
||||
"metadata": {},
|
||||
"outputs": [
|
||||
{
|
||||
"name": "stdout",
|
||||
"output_type": "stream",
|
||||
"text": [
|
||||
"len(result.data) = 2\n",
|
||||
"result.data[1].embedding = [-0.0108176339417696, 0.0013546717818826437, 0.00362232...\n",
|
||||
"len(result.data[1].embedding) = 1536\n"
|
||||
]
|
||||
}
|
||||
],
|
||||
"source": [
|
||||
"print(f\"len(result.data) = {len(result.data)}\")\n",
|
||||
"print(f\"result.data[1].embedding = {str(result.data[1].embedding)[:55]}...\")\n",
|
||||
"print(f\"len(result.data[1].embedding) = {len(result.data[1].embedding)}\")"
|
||||
]
|
||||
},
|
||||
{
|
||||
"cell_type": "markdown",
|
||||
"id": "d7f09c42-fff3-4aa2-922b-043739b4b06a",
|
||||
"metadata": {},
|
||||
"source": [
|
||||
"## Load quotes into the Vector Store"
|
||||
]
|
||||
},
|
||||
{
|
||||
"cell_type": "markdown",
|
||||
"id": "cf0f3d58-74c2-458b-903d-3d12e61b7846",
|
||||
"metadata": {},
|
||||
"source": [
|
||||
"Get a dataset with the quotes. _(We adapted and augmented the data from [this Kaggle dataset](https://www.kaggle.com/datasets/mertbozkurt5/quotes-by-philosophers), ready to use in this demo.)_"
|
||||
]
|
||||
},
|
||||
{
|
||||
"cell_type": "code",
|
||||
"execution_count": 9,
|
||||
"id": "aa68f038-3240-4e22-b7c6-a5f214eda381",
|
||||
"metadata": {},
|
||||
"outputs": [],
|
||||
"source": [
|
||||
"philo_dataset = load_dataset(\"datastax/philosopher-quotes\")[\"train\"]"
|
||||
]
|
||||
},
|
||||
{
|
||||
"cell_type": "markdown",
|
||||
"id": "ab6b08b1-e3db-4c7c-9d7c-2ada7c8bc71d",
|
||||
"metadata": {},
|
||||
"source": [
|
||||
"A quick inspection:"
|
||||
]
|
||||
},
|
||||
{
|
||||
"cell_type": "code",
|
||||
"execution_count": 10,
|
||||
"id": "10b629cf-efd7-434a-9dc6-7f38f35f7cc8",
|
||||
"metadata": {},
|
||||
"outputs": [
|
||||
{
|
||||
"name": "stdout",
|
||||
"output_type": "stream",
|
||||
"text": [
|
||||
"An example entry:\n",
|
||||
"{'author': 'aristotle', 'quote': 'Love well, be loved and do something of value.', 'tags': 'love;ethics'}\n"
|
||||
]
|
||||
}
|
||||
],
|
||||
"source": [
|
||||
"print(\"An example entry:\")\n",
|
||||
"print(philo_dataset[16])"
|
||||
]
|
||||
},
|
||||
{
|
||||
"cell_type": "markdown",
|
||||
"id": "9badaa4d-80ea-462c-bb00-1909c6435eea",
|
||||
"metadata": {},
|
||||
"source": [
|
||||
"Check the dataset size:"
|
||||
]
|
||||
},
|
||||
{
|
||||
"cell_type": "code",
|
||||
"execution_count": 11,
|
||||
"id": "1b33ac73-f8f2-4b64-8a27-178ac76886a9",
|
||||
"metadata": {},
|
||||
"outputs": [
|
||||
{
|
||||
"name": "stdout",
|
||||
"output_type": "stream",
|
||||
"text": [
|
||||
"Total: 450 quotes. By author:\n",
|
||||
" aristotle : 50 quotes\n",
|
||||
" schopenhauer : 50 quotes\n",
|
||||
" spinoza : 50 quotes\n",
|
||||
" hegel : 50 quotes\n",
|
||||
" freud : 50 quotes\n",
|
||||
" nietzsche : 50 quotes\n",
|
||||
" sartre : 50 quotes\n",
|
||||
" plato : 50 quotes\n",
|
||||
" kant : 50 quotes\n"
|
||||
]
|
||||
}
|
||||
],
|
||||
"source": [
|
||||
"author_count = Counter(entry[\"author\"] for entry in philo_dataset)\n",
|
||||
"print(f\"Total: {len(philo_dataset)} quotes. By author:\")\n",
|
||||
"for author, count in author_count.most_common():\n",
|
||||
" print(f\" {author:<20}: {count} quotes\")"
|
||||
]
|
||||
},
|
||||
{
|
||||
"cell_type": "markdown",
|
||||
"id": "062157d1-d262-4735-b06c-f3112575b4cc",
|
||||
"metadata": {},
|
||||
"source": [
|
||||
"### Write to the vector collection\n",
|
||||
"\n",
|
||||
"You will compute the embeddings for the quotes and save them into the Vector Store, along with the text itself and the metadata you'll use later.\n",
|
||||
"\n",
|
||||
"To optimize speed and reduce the calls, you'll perform batched calls to the embedding OpenAI service.\n",
|
||||
"\n",
|
||||
"To store the quote objects, you will use the `insert_many` method of the collection (one call per batch). When preparing the documents for insertion you will choose suitable field names -- keep in mind, however, that the embedding vector must be the fixed special `$vector` field."
|
||||
]
|
||||
},
|
||||
{
|
||||
"cell_type": "code",
|
||||
"execution_count": 12,
|
||||
"id": "6ab84ccb-3363-4bdc-9484-0d68c25a58ff",
|
||||
"metadata": {},
|
||||
"outputs": [
|
||||
{
|
||||
"name": "stdout",
|
||||
"output_type": "stream",
|
||||
"text": [
|
||||
"Starting to store entries: [20][20][20][20][20][20][20][20][20][20][20][20][20][20][20][20][20][20][20][20][20][20][10]\n",
|
||||
"Finished storing entries.\n"
|
||||
]
|
||||
}
|
||||
],
|
||||
"source": [
|
||||
"BATCH_SIZE = 20\n",
|
||||
"\n",
|
||||
"num_batches = ((len(philo_dataset) + BATCH_SIZE - 1) // BATCH_SIZE)\n",
|
||||
"\n",
|
||||
"quotes_list = philo_dataset[\"quote\"]\n",
|
||||
"authors_list = philo_dataset[\"author\"]\n",
|
||||
"tags_list = philo_dataset[\"tags\"]\n",
|
||||
"\n",
|
||||
"print(\"Starting to store entries: \", end=\"\")\n",
|
||||
"for batch_i in range(num_batches):\n",
|
||||
" b_start = batch_i * BATCH_SIZE\n",
|
||||
" b_end = (batch_i + 1) * BATCH_SIZE\n",
|
||||
" # compute the embedding vectors for this batch\n",
|
||||
" b_emb_results = client.embeddings.create(\n",
|
||||
" input=quotes_list[b_start : b_end],\n",
|
||||
" model=embedding_model_name,\n",
|
||||
" )\n",
|
||||
" # prepare the documents for insertion\n",
|
||||
" b_docs = []\n",
|
||||
" for entry_idx, emb_result in zip(range(b_start, b_end), b_emb_results.data):\n",
|
||||
" if tags_list[entry_idx]:\n",
|
||||
" tags = {\n",
|
||||
" tag: True\n",
|
||||
" for tag in tags_list[entry_idx].split(\";\")\n",
|
||||
" }\n",
|
||||
" else:\n",
|
||||
" tags = {}\n",
|
||||
" b_docs.append({\n",
|
||||
" \"quote\": quotes_list[entry_idx],\n",
|
||||
" \"$vector\": emb_result.embedding,\n",
|
||||
" \"author\": authors_list[entry_idx],\n",
|
||||
" \"tags\": tags,\n",
|
||||
" })\n",
|
||||
" # write to the vector collection\n",
|
||||
" collection.insert_many(b_docs)\n",
|
||||
" print(f\"[{len(b_docs)}]\", end=\"\")\n",
|
||||
"\n",
|
||||
"print(\"\\nFinished storing entries.\")"
|
||||
]
|
||||
},
|
||||
{
|
||||
"cell_type": "markdown",
|
||||
"id": "db3ee629-b6b9-4a77-8c58-c3b93403a6a6",
|
||||
"metadata": {},
|
||||
"source": [
|
||||
"## Use case 1: **quote search engine**"
|
||||
]
|
||||
},
|
||||
{
|
||||
"cell_type": "markdown",
|
||||
"id": "db3b12b3-2557-4826-af5a-16e6cd9a4531",
|
||||
"metadata": {},
|
||||
"source": [
|
||||
"For the quote-search functionality, you need first to make the input quote into a vector, and then use it to query the store (besides handling the optional metadata into the search call, that is).\n",
|
||||
"\n",
|
||||
"Encapsulate the search-engine functionality into a function for ease of re-use. At its core is the `vector_find` method of the collection:"
|
||||
]
|
||||
},
|
||||
{
|
||||
"cell_type": "code",
|
||||
"execution_count": 13,
|
||||
"id": "d6fcf182-3ab7-4d28-9472-dce35cc38182",
|
||||
"metadata": {},
|
||||
"outputs": [],
|
||||
"source": [
|
||||
"def find_quote_and_author(query_quote, n, author=None, tags=None):\n",
|
||||
" query_vector = client.embeddings.create(\n",
|
||||
" input=[query_quote],\n",
|
||||
" model=embedding_model_name,\n",
|
||||
" ).data[0].embedding\n",
|
||||
" filter_clause = {}\n",
|
||||
" if author:\n",
|
||||
" filter_clause[\"author\"] = author\n",
|
||||
" if tags:\n",
|
||||
" filter_clause[\"tags\"] = {}\n",
|
||||
" for tag in tags:\n",
|
||||
" filter_clause[\"tags\"][tag] = True\n",
|
||||
" #\n",
|
||||
" results = collection.vector_find(\n",
|
||||
" query_vector,\n",
|
||||
" limit=n,\n",
|
||||
" filter=filter_clause,\n",
|
||||
" fields=[\"quote\", \"author\"]\n",
|
||||
" )\n",
|
||||
" return [\n",
|
||||
" (result[\"quote\"], result[\"author\"])\n",
|
||||
" for result in results\n",
|
||||
" ]"
|
||||
]
|
||||
},
|
||||
{
|
||||
"cell_type": "markdown",
|
||||
"id": "2539262d-100b-4e8d-864d-e9c612a73e91",
|
||||
"metadata": {},
|
||||
"source": [
|
||||
"### Putting search to test"
|
||||
]
|
||||
},
|
||||
{
|
||||
"cell_type": "markdown",
|
||||
"id": "3634165c-0882-4281-bc60-ab96261a500d",
|
||||
"metadata": {},
|
||||
"source": [
|
||||
"Passing just a quote:"
|
||||
]
|
||||
},
|
||||
{
|
||||
"cell_type": "code",
|
||||
"execution_count": 14,
|
||||
"id": "6722c2c0-3e54-4738-80ce-4d1149e95414",
|
||||
"metadata": {},
|
||||
"outputs": [
|
||||
{
|
||||
"data": {
|
||||
"text/plain": [
|
||||
"[('Life to the great majority is only a constant struggle for mere existence, with the certainty of losing it at last.',\n",
|
||||
" 'schopenhauer'),\n",
|
||||
" ('We give up leisure in order that we may have leisure, just as we go to war in order that we may have peace.',\n",
|
||||
" 'aristotle'),\n",
|
||||
" ('Perhaps the gods are kind to us, by making life more disagreeable as we grow older. In the end death seems less intolerable than the manifold burdens we carry',\n",
|
||||
" 'freud')]"
|
||||
]
|
||||
},
|
||||
"execution_count": 14,
|
||||
"metadata": {},
|
||||
"output_type": "execute_result"
|
||||
}
|
||||
],
|
||||
"source": [
|
||||
"find_quote_and_author(\"We struggle all our life for nothing\", 3)"
|
||||
]
|
||||
},
|
||||
{
|
||||
"cell_type": "markdown",
|
||||
"id": "50828e4c-9bb5-4489-9fe9-87da5fbe1f18",
|
||||
"metadata": {},
|
||||
"source": [
|
||||
"Search restricted to an author:"
|
||||
]
|
||||
},
|
||||
{
|
||||
"cell_type": "code",
|
||||
"execution_count": 15,
|
||||
"id": "da9c705f-5c12-42b3-a038-202f89a3c6da",
|
||||
"metadata": {},
|
||||
"outputs": [
|
||||
{
|
||||
"data": {
|
||||
"text/plain": [
|
||||
"[('To live is to suffer, to survive is to find some meaning in the suffering.',\n",
|
||||
" 'nietzsche'),\n",
|
||||
" ('What makes us heroic?--Confronting simultaneously our supreme suffering and our supreme hope.',\n",
|
||||
" 'nietzsche')]"
|
||||
]
|
||||
},
|
||||
"execution_count": 15,
|
||||
"metadata": {},
|
||||
"output_type": "execute_result"
|
||||
}
|
||||
],
|
||||
"source": [
|
||||
"find_quote_and_author(\"We struggle all our life for nothing\", 2, author=\"nietzsche\")"
|
||||
]
|
||||
},
|
||||
{
|
||||
"cell_type": "markdown",
|
||||
"id": "4a3857ea-6dfe-489a-9b86-4e5e0534960f",
|
||||
"metadata": {},
|
||||
"source": [
|
||||
"Search constrained to a tag (out of those saved earlier with the quotes):"
|
||||
]
|
||||
},
|
||||
{
|
||||
"cell_type": "code",
|
||||
"execution_count": 16,
|
||||
"id": "abcfaec9-8f42-4789-a5ed-1073fa2932c2",
|
||||
"metadata": {},
|
||||
"outputs": [
|
||||
{
|
||||
"data": {
|
||||
"text/plain": [
|
||||
"[('He who seeks equality between unequals seeks an absurdity.', 'spinoza'),\n",
|
||||
" ('The people are that part of the state that does not know what it wants.',\n",
|
||||
" 'hegel')]"
|
||||
]
|
||||
},
|
||||
"execution_count": 16,
|
||||
"metadata": {},
|
||||
"output_type": "execute_result"
|
||||
}
|
||||
],
|
||||
"source": [
|
||||
"find_quote_and_author(\"We struggle all our life for nothing\", 2, tags=[\"politics\"])"
|
||||
]
|
||||
},
|
||||
{
|
||||
"cell_type": "markdown",
|
||||
"id": "746fe38f-139f-44a6-a225-a63e40d3ddf5",
|
||||
"metadata": {},
|
||||
"source": [
|
||||
"### Cutting out irrelevant results\n",
|
||||
"\n",
|
||||
"The vector similarity search generally returns the vectors that are closest to the query, even if that means results that might be somewhat irrelevant if there's nothing better.\n",
|
||||
"\n",
|
||||
"To keep this issue under control, you can get the actual \"similarity\" between the query and each result, and then implement a cutoff on it, effectively discarding results that are beyond that threshold.\n",
|
||||
"Tuning this threshold correctly is not an easy problem: here, we'll just show you the way.\n",
|
||||
"\n",
|
||||
"To get a feeling on how this works, try the following query and play with the choice of quote and threshold to compare the results. Note that the similarity is returned as the special `$similarity` field in each result document - and it will be returned by default, unless you pass `include_similarity = False` to the search method.\n",
|
||||
"\n",
|
||||
"_Note (for the mathematically inclined): this value is **a rescaling between zero and one** of the cosine difference between the vectors, i.e. of the scalar product divided by the product of the norms of the two vectors. In other words, this is 0 for opposite-facing vectors and +1 for parallel vectors. For other measures of similarity (cosine is the default), check the `metric` parameter in `AstraDB.create_collection` and the [documentation on allowed values](https://docs.datastax.com/en/astra-serverless/docs/develop/dev-with-json.html#metric-types)._"
|
||||
]
|
||||
},
|
||||
{
|
||||
"cell_type": "code",
|
||||
"execution_count": 17,
|
||||
"id": "b9b43721-a3b0-4ac4-b730-7a6aeec52e70",
|
||||
"metadata": {},
|
||||
"outputs": [
|
||||
{
|
||||
"name": "stdout",
|
||||
"output_type": "stream",
|
||||
"text": [
|
||||
"3 quotes within the threshold:\n",
|
||||
" 0. [similarity=0.927] \"The assumption that animals are without rights, and the illusion that ...\"\n",
|
||||
" 1. [similarity=0.922] \"Animals are in possession of themselves; their soul is in possession o...\"\n",
|
||||
" 2. [similarity=0.920] \"At his best, man is the noblest of all animals; separated from law and...\"\n"
|
||||
]
|
||||
}
|
||||
],
|
||||
"source": [
|
||||
"quote = \"Animals are our equals.\"\n",
|
||||
"# quote = \"Be good.\"\n",
|
||||
"# quote = \"This teapot is strange.\"\n",
|
||||
"\n",
|
||||
"metric_threshold = 0.92\n",
|
||||
"\n",
|
||||
"quote_vector = client.embeddings.create(\n",
|
||||
" input=[quote],\n",
|
||||
" model=embedding_model_name,\n",
|
||||
").data[0].embedding\n",
|
||||
"\n",
|
||||
"results_full = collection.vector_find(\n",
|
||||
" quote_vector,\n",
|
||||
" limit=8,\n",
|
||||
" fields=[\"quote\"]\n",
|
||||
")\n",
|
||||
"results = [res for res in results_full if res[\"$similarity\"] >= metric_threshold]\n",
|
||||
"\n",
|
||||
"print(f\"{len(results)} quotes within the threshold:\")\n",
|
||||
"for idx, result in enumerate(results):\n",
|
||||
" print(f\" {idx}. [similarity={result['$similarity']:.3f}] \\\"{result['quote'][:70]}...\\\"\")"
|
||||
]
|
||||
},
|
||||
{
|
||||
"cell_type": "markdown",
|
||||
"id": "71871251-169f-4d3f-a687-65f836a9a8fe",
|
||||
"metadata": {},
|
||||
"source": [
|
||||
"## Use case 2: **quote generator**"
|
||||
]
|
||||
},
|
||||
{
|
||||
"cell_type": "markdown",
|
||||
"id": "b0a9cd63-a131-4819-bf41-c8ffa0b1e1ca",
|
||||
"metadata": {},
|
||||
"source": [
|
||||
"For this task you need another component from OpenAI, namely an LLM to generate the quote for us (based on input obtained by querying the Vector Store).\n",
|
||||
"\n",
|
||||
"You also need a template for the prompt that will be filled for the generate-quote LLM completion task."
|
||||
]
|
||||
},
|
||||
{
|
||||
"cell_type": "code",
|
||||
"execution_count": 18,
|
||||
"id": "a6dd366d-665a-45fd-917b-b6b5312b0865",
|
||||
"metadata": {},
|
||||
"outputs": [],
|
||||
"source": [
|
||||
"completion_model_name = \"gpt-3.5-turbo\"\n",
|
||||
"\n",
|
||||
"generation_prompt_template = \"\"\"\"Generate a single short philosophical quote on the given topic,\n",
|
||||
"similar in spirit and form to the provided actual example quotes.\n",
|
||||
"Do not exceed 20-30 words in your quote.\n",
|
||||
"\n",
|
||||
"REFERENCE TOPIC: \"{topic}\"\n",
|
||||
"\n",
|
||||
"ACTUAL EXAMPLES:\n",
|
||||
"{examples}\n",
|
||||
"\"\"\""
|
||||
]
|
||||
},
|
||||
{
|
||||
"cell_type": "markdown",
|
||||
"id": "53073a9e-16de-4e49-9e97-ff31b9b250c2",
|
||||
"metadata": {},
|
||||
"source": [
|
||||
"Like for search, this functionality is best wrapped into a handy function (which internally uses search):"
|
||||
]
|
||||
},
|
||||
{
|
||||
"cell_type": "code",
|
||||
"execution_count": 19,
|
||||
"id": "397e6ebd-b30e-413b-be63-81a62947a7b8",
|
||||
"metadata": {},
|
||||
"outputs": [],
|
||||
"source": [
|
||||
"def generate_quote(topic, n=2, author=None, tags=None):\n",
|
||||
" quotes = find_quote_and_author(query_quote=topic, n=n, author=author, tags=tags)\n",
|
||||
" if quotes:\n",
|
||||
" prompt = generation_prompt_template.format(\n",
|
||||
" topic=topic,\n",
|
||||
" examples=\"\\n\".join(f\" - {quote[0]}\" for quote in quotes),\n",
|
||||
" )\n",
|
||||
" # a little logging:\n",
|
||||
" print(\"** quotes found:\")\n",
|
||||
" for q, a in quotes:\n",
|
||||
" print(f\"** - {q} ({a})\")\n",
|
||||
" print(\"** end of logging\")\n",
|
||||
" #\n",
|
||||
" response = client.chat.completions.create(\n",
|
||||
" model=completion_model_name,\n",
|
||||
" messages=[{\"role\": \"user\", \"content\": prompt}],\n",
|
||||
" temperature=0.7,\n",
|
||||
" max_tokens=320,\n",
|
||||
" )\n",
|
||||
" return response.choices[0].message.content.replace('\"', '').strip()\n",
|
||||
" else:\n",
|
||||
" print(\"** no quotes found.\")\n",
|
||||
" return None"
|
||||
]
|
||||
},
|
||||
{
|
||||
"cell_type": "markdown",
|
||||
"id": "c13f8488-899b-4d4c-a069-73643a778200",
|
||||
"metadata": {},
|
||||
"source": [
|
||||
"_Note: similar to the case of the embedding computation, the code for the Chat Completion API would be slightly different for OpenAI prior to v1.0._"
|
||||
]
|
||||
},
|
||||
{
|
||||
"cell_type": "markdown",
|
||||
"id": "63bcc157-e5d4-43ef-8028-d4dcc8a72b9c",
|
||||
"metadata": {},
|
||||
"source": [
|
||||
"#### Putting quote generation to test"
|
||||
]
|
||||
},
|
||||
{
|
||||
"cell_type": "markdown",
|
||||
"id": "fe6b3f38-089d-486d-b32c-e665c725faa8",
|
||||
"metadata": {},
|
||||
"source": [
|
||||
"Just passing a text (a \"quote\", but one can actually just suggest a topic since its vector embedding will still end up at the right place in the vector space):"
|
||||
]
|
||||
},
|
||||
{
|
||||
"cell_type": "code",
|
||||
"execution_count": 20,
|
||||
"id": "806ba758-8988-410e-9eeb-b9c6799e6b25",
|
||||
"metadata": {},
|
||||
"outputs": [
|
||||
{
|
||||
"name": "stdout",
|
||||
"output_type": "stream",
|
||||
"text": [
|
||||
"** quotes found:\n",
|
||||
"** - Happiness is the reward of virtue. (aristotle)\n",
|
||||
"** - Our moral virtues benefit mainly other people; intellectual virtues, on the other hand, benefit primarily ourselves; therefore the former make us universally popular, the latter unpopular. (schopenhauer)\n",
|
||||
"** end of logging\n",
|
||||
"\n",
|
||||
"A new generated quote:\n",
|
||||
"True politics lies in the virtuous pursuit of justice, for it is through virtue that we build a better world for all.\n"
|
||||
]
|
||||
}
|
||||
],
|
||||
"source": [
|
||||
"q_topic = generate_quote(\"politics and virtue\")\n",
|
||||
"print(\"\\nA new generated quote:\")\n",
|
||||
"print(q_topic)"
|
||||
]
|
||||
},
|
||||
{
|
||||
"cell_type": "markdown",
|
||||
"id": "ca032d30-4538-4d0b-aea1-731fb32d2d4b",
|
||||
"metadata": {},
|
||||
"source": [
|
||||
"Use inspiration from just a single philosopher:"
|
||||
]
|
||||
},
|
||||
{
|
||||
"cell_type": "code",
|
||||
"execution_count": 21,
|
||||
"id": "7c2e2d4e-865f-4b2d-80cd-a695271415d9",
|
||||
"metadata": {},
|
||||
"outputs": [
|
||||
{
|
||||
"name": "stdout",
|
||||
"output_type": "stream",
|
||||
"text": [
|
||||
"** quotes found:\n",
|
||||
"** - Because Christian morality leaves animals out of account, they are at once outlawed in philosophical morals; they are mere 'things,' mere means to any ends whatsoever. They can therefore be used for vivisection, hunting, coursing, bullfights, and horse racing, and can be whipped to death as they struggle along with heavy carts of stone. Shame on such a morality that is worthy of pariahs, and that fails to recognize the eternal essence that exists in every living thing, and shines forth with inscrutable significance from all eyes that see the sun! (schopenhauer)\n",
|
||||
"** - The assumption that animals are without rights, and the illusion that our treatment of them has no moral significance, is a positively outrageous example of Western crudity and barbarity. Universal compassion is the only guarantee of morality. (schopenhauer)\n",
|
||||
"** end of logging\n",
|
||||
"\n",
|
||||
"A new generated quote:\n",
|
||||
"Excluding animals from ethical consideration reveals a moral blindness that allows for their exploitation and suffering. True morality embraces universal compassion.\n"
|
||||
]
|
||||
}
|
||||
],
|
||||
"source": [
|
||||
"q_topic = generate_quote(\"animals\", author=\"schopenhauer\")\n",
|
||||
"print(\"\\nA new generated quote:\")\n",
|
||||
"print(q_topic)"
|
||||
]
|
||||
},
|
||||
{
|
||||
"cell_type": "markdown",
|
||||
"id": "4bd8368a-9e23-49a5-8694-921728ea9656",
|
||||
"metadata": {},
|
||||
"source": [
|
||||
"## Cleanup\n",
|
||||
"\n",
|
||||
"If you want to remove all resources used for this demo, run this cell (_warning: this will irreversibly delete the collection and its data!_):"
|
||||
]
|
||||
},
|
||||
{
|
||||
"cell_type": "code",
|
||||
"execution_count": 22,
|
||||
"id": "1eb0fd16-7e15-4742-8fc5-94d9eeeda620",
|
||||
"metadata": {},
|
||||
"outputs": [
|
||||
{
|
||||
"data": {
|
||||
"text/plain": [
|
||||
"{'status': {'ok': 1}}"
|
||||
]
|
||||
},
|
||||
"execution_count": 22,
|
||||
"metadata": {},
|
||||
"output_type": "execute_result"
|
||||
}
|
||||
],
|
||||
"source": [
|
||||
"astra_db.delete_collection(coll_name)"
|
||||
]
|
||||
}
|
||||
],
|
||||
"metadata": {
|
||||
"kernelspec": {
|
||||
"display_name": "Python 3 (ipykernel)",
|
||||
"language": "python",
|
||||
"name": "python3"
|
||||
},
|
||||
"language_info": {
|
||||
"codemirror_mode": {
|
||||
"name": "ipython",
|
||||
"version": 3
|
||||
},
|
||||
"file_extension": ".py",
|
||||
"mimetype": "text/x-python",
|
||||
"name": "python",
|
||||
"nbconvert_exporter": "python",
|
||||
"pygments_lexer": "ipython3",
|
||||
"version": "3.9.18"
|
||||
}
|
||||
},
|
||||
"nbformat": 4,
|
||||
"nbformat_minor": 5
|
||||
}
|
Binary file not shown.
After Width: | Height: | Size: 557 KiB |
Loading…
Reference in New Issue