This input key was missed in the last update PR:
https://github.com/langchain-ai/langchain/pull/7391
The input/output formats are intended to be like this:
```
{"inputs": [<prompt>]}
{"outputs": [<output_text>]}
```
<!-- Thank you for contributing to LangChain!
Replace this entire comment with:
- **Description:** a description of the change,
- **Issue:** the issue # it fixes (if applicable),
- **Dependencies:** any dependencies required for this change,
- **Tag maintainer:** for a quicker response, tag the relevant
maintainer (see below),
- **Twitter handle:** we announce bigger features on Twitter. If your PR
gets announced, and you'd like a mention, we'll gladly shout you out!
Please make sure your PR is passing linting and testing before
submitting. Run `make format`, `make lint` and `make test` to check this
locally.
See contribution guidelines for more information on how to write/run
tests, lint, etc:
https://github.com/langchain-ai/langchain/blob/master/.github/CONTRIBUTING.md
If you're adding a new integration, please include:
1. a test for the integration, preferably unit tests that do not rely on
network access,
2. an example notebook showing its use. It lives in `docs/extras`
directory.
If no one reviews your PR within a few days, please @-mention one of
@baskaryan, @eyurtsev, @hwchase17.
-->
---------
Co-authored-by: Matvey Arye <mat@timescale.com>
## Description
This PR adds support for
[lm-format-enforcer](https://github.com/noamgat/lm-format-enforcer) to
LangChain.
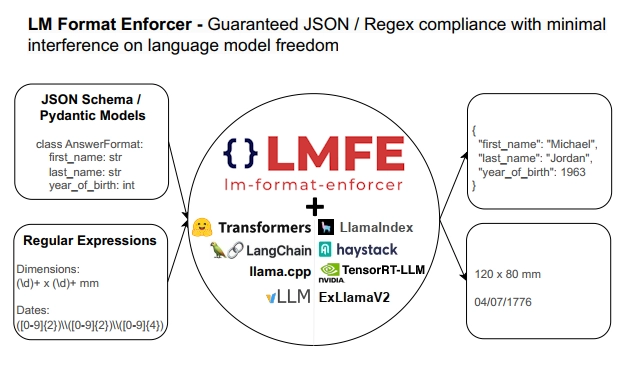
The library is similar to jsonformer / RELLM which are supported in
Langchain, but has several advantages such as
- Batching and Beam search support
- More complete JSON Schema support
- LLM has control over whitespace, improving quality
- Better runtime performance due to only calling the LLM's generate()
function once per generate() call.
The integration is loosely based on the jsonformer integration in terms
of project structure.
## Dependencies
No compile-time dependency was added, but if `lm-format-enforcer` is not
installed, a runtime error will occur if it is trying to be used.
## Tests
Due to the integration modifying the internal parameters of the
underlying huggingface transformer LLM, it is not possible to test
without building a real LM, which requires internet access. So, similar
to the jsonformer and RELLM integrations, the testing is via the
notebook.
## Twitter Handle
[@noamgat](https://twitter.com/noamgat)
Looking forward to hearing feedback!
---------
Co-authored-by: Bagatur <baskaryan@gmail.com>
This PR addresses what seems like a unnecessary Python version
restriction in the pyroject.toml specs within both Cassandra (/Astra DB)
templates. With "^3.11" I got some version incompatibilities with the
latest "langchain add [...]" commands, so these are now relaxed in line
with the other templates I could inspect.
Incidentally, in the "entomology" template, the need for an explicit
"setup" step for the user to carry on has been removed, replaced by a
check-and-execute-if-necessary instruction on app startup.
Thank you for your attention!
Best to review one commit at a time, since two of the commits are 100%
autogenerated changes from running `ruff format`:
- Install and use `ruff format` instead of black for code formatting.
- Output of `ruff format .` in the `langchain` package.
- Use `ruff format` in experimental package.
- Format changes in experimental package by `ruff format`.
- Manual formatting fixes to make `ruff .` pass.
I always take 20-30 seconds to re-discover where the
`convert_to_openai_function` wrapper lives in our codebase. Chat
langchain [has no
clue](https://smith.langchain.com/public/3989d687-18c7-4108-958e-96e88803da86/r)
what to do either. There's the older `create_openai_fn_chain` , but we
haven't been recommending it in LCEL. The example we show in the
[cookbook](https://python.langchain.com/docs/expression_language/how_to/binding#attaching-openai-functions)
is really verbose.
General function calling should be as simple as possible to do, so this
seems a bit more ergonomic to me (feel free to disagree). Another option
would be to directly coerce directly in the class's init (or when
calling invoke), if provided. I'm not 100% set against that. That
approach may be too easy but not simple. This PR feels like a decent
compromise between simple and easy.
```
from enum import Enum
from typing import Optional
from pydantic import BaseModel, Field
class Category(str, Enum):
"""The category of the issue."""
bug = "bug"
nit = "nit"
improvement = "improvement"
other = "other"
class IssueClassification(BaseModel):
"""Classify an issue."""
category: Category
other_description: Optional[str] = Field(
description="If classified as 'other', the suggested other category"
)
from langchain.chat_models import ChatOpenAI
llm = ChatOpenAI().bind_functions([IssueClassification])
llm.invoke("This PR adds a convenience wrapper to the bind argument")
# AIMessage(content='', additional_kwargs={'function_call': {'name': 'IssueClassification', 'arguments': '{\n "category": "improvement"\n}'}})
```
- Prefer lambda type annotations over inferred dict schema
- For sequences that start with RunnableAssign infer seq input type as
"input type of 2nd item in sequence - output type of runnable assign"
- **Description:**
Before:
`
To install modules needed for the common LLM providers, run:
`
After:
`
To install modules needed for the common LLM providers, run the
following command. Please bear in mind that this command is exclusively
compatible with the `bash` shell:
`
> This is required for the user so that the user will know if this
command is compatible with `zsh` or not.
---------
Co-authored-by: Bagatur <baskaryan@gmail.com>
Replace this entire comment with:
-Add MultiOn close function and update key value and add async
functionality
- solved the key value TabId not found.. (updated to use latest key
value)
@hwchase17
- **Description:** This pull request removes secrets present in raw
format,
- **Issue:** Fireworks api key was exposed when printing out the
langchain object
[#12165](https://github.com/langchain-ai/langchain/issues/12165)
- **Maintainer:** @eyurtsev
---------
Co-authored-by: Bagatur <baskaryan@gmail.com>
**Description:** Textract PDF Loader generating linearized output,
meaning it will replicate the structure of the source document as close
as possible based on the features passed into the call (e. g. LAYOUT,
FORMS, TABLES). With LAYOUT reading order for multi-column documents or
identification of lists and figures is supported and with TABLES it will
generate the table structure as well. FORMS will indicate "key: value"
with columms.
- **Issue:** the issue fixes#12068
- **Dependencies:** amazon-textract-textractor is added, which provides
the linearization
- **Tag maintainer:** @3coins
---------
Co-authored-by: Bagatur <baskaryan@gmail.com>
Ran the following bash script for all templates
```bash
#!/bin/bash
set -e
current_dir="$(pwd)"
for directory in */; do
if [ -d "$directory" ]; then
(cd "$directory" && poetry lock --no-update)
fi
done
cd "$current_dir"
```
Co-authored-by: Bagatur <baskaryan@gmail.com>
can get the correct token count instead of using gpt-2 model
**Description:**
Implement get_num_tokens within VertexLLM to use google's count_tokens
function.
(https://cloud.google.com/vertex-ai/docs/generative-ai/get-token-count).
So we don't need to download gpt-2 model from huggingface, also when we
do the mapreduce chain we can get correct token count.
**Tag maintainer:**
@lkuligin
**Twitter handle:**
My twitter: @abehsu1992626
---------
Co-authored-by: Bagatur <baskaryan@gmail.com>
Following this tutoral about using OpenAI Embeddings with FAISS
https://python.langchain.com/docs/integrations/vectorstores/faiss
```python
from langchain.embeddings.openai import OpenAIEmbeddings
from langchain.text_splitter import CharacterTextSplitter
from langchain.vectorstores import FAISS
from langchain.document_loaders import TextLoader
from langchain.document_loaders import TextLoader
loader = TextLoader("../../../extras/modules/state_of_the_union.txt")
documents = loader.load()
text_splitter = CharacterTextSplitter(chunk_size=1000, chunk_overlap=0)
docs = text_splitter.split_documents(documents)
embeddings = OpenAIEmbeddings()
```
This works fine
```python
db = FAISS.from_documents(docs, embeddings)
query = "What did the president say about Ketanji Brown Jackson"
docs = db.similarity_search(query)
```
But the async version is not
```python
db = await FAISS.afrom_documents(docs, embeddings) # NotImplementedError
query = "What did the president say about Ketanji Brown Jackson"
docs = await db.asimilarity_search(query) # this will use await asyncio.get_event_loop().run_in_executor under the hood and will not call OpenAIEmbeddings.aembed_query but call OpenAIEmbeddings.embed_query
```
So this PR add async/await supports for FAISS
---------
Co-authored-by: Eugene Yurtsev <eyurtsev@gmail.com>