# OpenAIWhisperParser
This PR creates a new parser, `OpenAIWhisperParser`, that uses the
[OpenAI Whisper
model](https://platform.openai.com/docs/guides/speech-to-text/quickstart)
to perform transcription of audio files to text (`Documents`). Please
see the notebook for usage.
Zep now supports persisting custom metadata with messages and hybrid
search across both message embeddings and structured metadata. This PR
implements custom metadata and enhancements to the
`ZepChatMessageHistory` and `ZepRetriever` classes to implement this
support.
Tag maintainers/contributors who might be interested:
VectorStores / Retrievers / Memory
- @dev2049
---------
Co-authored-by: Daniel Chalef <daniel.chalef@private.org>
# Check if generated Cypher code is wrapped in backticks
Some LLMs like the VertexAI like to explain how they generated the
Cypher statement and wrap the actual code in three backticks:
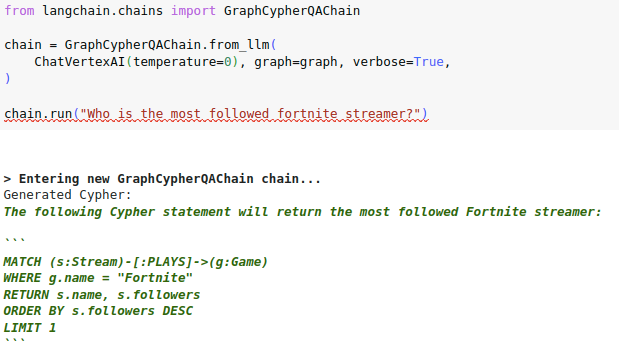
I have observed a similar pattern with OpenAI chat models in a
conversational settings, where multiple user and assistant message are
provided to the LLM to generate Cypher statements, where then the LLM
wants to maybe apologize for previous steps or explain its thoughts.
Interestingly, both OpenAI and VertexAI wrap the code in three backticks
if they are doing any explaining or apologizing. Checking if the
generated cypher is wrapped in backticks seems like a low-hanging fruit
to expand the cypher search to other LLMs and conversational settings.
# Token text splitter for sentence transformers
The current TokenTextSplitter only works with OpenAi models via the
`tiktoken` package. This is not clear from the name `TokenTextSplitter`.
In this (first PR) a token based text splitter for sentence transformer
models is added. In the future I think we should work towards injecting
a tokenizer into the TokenTextSplitter to make ti more flexible.
Could perhaps be reviewed by @dev2049
---------
Co-authored-by: Harrison Chase <hw.chase.17@gmail.com>
Raises exception if OutputParsers receive a response with both a valid
action and a final answer
Currently, if an OutputParser receives a response which includes both an
action and a final answer, they return a FinalAnswer object. This allows
the parser to accept responses which propose an action and hallucinate
an answer without the action being parsed or taken by the agent.
This PR changes the logic to:
1. store a variable checking whether a response contains the
`FINAL_ANSWER_ACTION` (this is the easier condition to check).
2. store a variable checking whether the response contains a valid
action
3. if both are present, raise a new exception stating that both are
present
4. if an action is present, return an AgentAction
5. if an answer is present, return an AgentAnswer
6. if neither is present, raise the relevant exception based around the
action format (these have been kept consistent with the prior exception
messages)
Disclaimer:
* Existing mock data included strings which did include an action and an
answer. This might indicate that prioritising returning AgentAnswer was
always correct, and I am patching out desired behaviour? @hwchase17 to
advice. Curious if there are allowed cases where this is not
hallucinating, and we do want the LLM to output an action which isn't
taken.
* I have not passed `send_to_llm` through this new exception
Fixes#5601
## Who can review?
Community members can review the PR once tests pass. Tag
maintainers/contributors who might be interested:
@hwchase17 - project lead
@vowelparrot
# Unstructured Excel Loader
Adds an `UnstructuredExcelLoader` class for `.xlsx` and `.xls` files.
Works with `unstructured>=0.6.7`. A plain text representation of the
Excel file will be available under the `page_content` attribute in the
doc. If you use the loader in `"elements"` mode, an HTML representation
of the Excel file will be available under the `text_as_html` metadata
key. Each sheet in the Excel document is its own document.
### Testing
```python
from langchain.document_loaders import UnstructuredExcelLoader
loader = UnstructuredExcelLoader(
"example_data/stanley-cups.xlsx",
mode="elements"
)
docs = loader.load()
```
## Who can review?
@hwchase17
@eyurtsev
# Chroma update_document full document embeddings bugfix
Chroma update_document takes a single document, but treats the
page_content sting of that document as a list when getting the new
document embedding.
This is a two-fold problem, where the resulting embedding for the
updated document is incorrect (it's only an embedding of the first
character in the new page_content) and it calls the embedding function
for every character in the new page_content string, using many tokens in
the process.
Fixes#5582
Co-authored-by: Caleb Ellington <calebellington@Calebs-MBP.hsd1.ca.comcast.net>
# Fix Qdrant ids creation
There has been a bug in how the ids were created in the Qdrant vector
store. They were previously calculated based on the texts. However,
there are some scenarios in which two documents may have the same piece
of text but different metadata, and that's a valid case. Deduplication
should be done outside of insertion.
It has been fixed and covered with the integration tests.
---------
Co-authored-by: Dev 2049 <dev.dev2049@gmail.com>
# Fixes SQLAlchemy truncating the result if you have a big/text column
with many chars.
SQLAlchemy truncates columns if you try to convert a Row or Sequence to
a string directly
For comparison:
- Before:
```[('Harrison', 'That is my Bio That is my Bio That is my Bio That is my Bio That is my Bio That is my Bio That is my Bio That is my Bio That is my Bio That is my Bio ... (2 characters truncated) ... hat is my Bio That is my Bio That is my Bio That is my Bio That is my Bio That is my Bio That is my Bio That is my Bio That is my Bio That is my Bio ')]```
- After:
```[('Harrison', 'That is my Bio That is my Bio That is my Bio That is
my Bio That is my Bio That is my Bio That is my Bio That is my Bio That
is my Bio That is my Bio That is my Bio That is my Bio That is my Bio
That is my Bio That is my Bio That is my Bio That is my Bio That is my
Bio That is my Bio That is my Bio ')]```
## Who can review?
Community members can review the PR once tests pass. Tag
maintainers/contributors who might be interested:
I'm not sure who to tag for chains, maybe @vowelparrot ?
Similar to #1813 for faiss, this PR is to extend functionality to pass
text and its vector pair to initialize and add embeddings to the
PGVector wrapper.
Community members can review the PR once tests pass. Tag
maintainers/contributors who might be interested:
- @dev2049
# Support Qdrant filters
Qdrant has an [extensive filtering
system](https://qdrant.tech/documentation/concepts/filtering/) with rich
type support. This PR makes it possible to use the filters in Langchain
by passing an additional param to both the
`similarity_search_with_score` and `similarity_search` methods.
## Who can review?
@dev2049 @hwchase17
---------
Co-authored-by: Dev 2049 <dev.dev2049@gmail.com>
when the LLMs output 'yes|no',BooleanOutputParser can parse it to
'True|False', fix the ValueError in parse().
<!--
when use the BooleanOutputParser in the chain_filter.py, the LLMs output
'yes|no',the function 'parse' will throw ValueError。
-->
Fixes # (issue)
#5396https://github.com/hwchase17/langchain/issues/5396
---------
Co-authored-by: gaofeng27692 <gaofeng27692@hundsun.com>
# Add maximal relevance search to SKLearnVectorStore
This PR implements the maximum relevance search in SKLearnVectorStore.
Twitter handle: jtolgyesi (I submitted also the original implementation
of SKLearnVectorStore)
## Before submitting
Unit tests are included.
Co-authored-by: Dev 2049 <dev.dev2049@gmail.com>
# Add batching to Qdrant
Several people requested a batching mechanism while uploading data to
Qdrant. It is important, as there are some limits for the maximum size
of the request payload, and without batching implemented in Langchain,
users need to implement it on their own. This PR exposes a new optional
`batch_size` parameter, so all the documents/texts are loaded in batches
of the expected size (64, by default).
The integration tests of Qdrant are extended to cover two cases:
1. Documents are sent in separate batches.
2. All the documents are sent in a single request.
# Handles the edge scenario in which the action input is a well formed
SQL query which ends with a quoted column
There may be a cleaner option here (or indeed other edge scenarios) but
this seems to robustly determine if the action input is likely to be a
well formed SQL query in which we don't want to arbitrarily trim off `"`
characters
Fixes#5423
## Who can review?
Community members can review the PR once tests pass. Tag
maintainers/contributors who might be interested:
For a quicker response, figure out the right person to tag with @
@hwchase17 - project lead
Agents / Tools / Toolkits
- @vowelparrot
# What does this PR do?
Bring support of `encode_kwargs` for ` HuggingFaceInstructEmbeddings`,
change the docstring example and add a test to illustrate with
`normalize_embeddings`.
Fixes#3605
(Similar to #3914)
Use case:
```python
from langchain.embeddings import HuggingFaceInstructEmbeddings
model_name = "hkunlp/instructor-large"
model_kwargs = {'device': 'cpu'}
encode_kwargs = {'normalize_embeddings': True}
hf = HuggingFaceInstructEmbeddings(
model_name=model_name,
model_kwargs=model_kwargs,
encode_kwargs=encode_kwargs
)
```
As the title says, I added more code splitters.
The implementation is trivial, so i don't add separate tests for each
splitter.
Let me know if any concerns.
Fixes # (issue)
https://github.com/hwchase17/langchain/issues/5170
## Who can review?
Community members can review the PR once tests pass. Tag
maintainers/contributors who might be interested:
@eyurtsev @hwchase17
---------
Signed-off-by: byhsu <byhsu@linkedin.com>
Co-authored-by: byhsu <byhsu@linkedin.com>
# Creates GitHubLoader (#5257)
GitHubLoader is a DocumentLoader that loads issues and PRs from GitHub.
Fixes#5257
---------
Co-authored-by: Dev 2049 <dev.dev2049@gmail.com>
# Added New Trello loader class and documentation
Simple Loader on top of py-trello wrapper.
With a board name you can pull cards and to do some field parameter
tweaks on load operation.
I included documentation and examples.
Included unit test cases using patch and a fixture for py-trello client
class.
---------
Co-authored-by: Dev 2049 <dev.dev2049@gmail.com>
# Add ToolException that a tool can throw
This is an optional exception that tool throws when execution error
occurs.
When this exception is thrown, the agent will not stop working,but will
handle the exception according to the handle_tool_error variable of the
tool,and the processing result will be returned to the agent as
observation,and printed in pink on the console.It can be used like this:
```python
from langchain.schema import ToolException
from langchain import LLMMathChain, SerpAPIWrapper, OpenAI
from langchain.agents import AgentType, initialize_agent
from langchain.chat_models import ChatOpenAI
from langchain.tools import BaseTool, StructuredTool, Tool, tool
from langchain.chat_models import ChatOpenAI
llm = ChatOpenAI(temperature=0)
llm_math_chain = LLMMathChain(llm=llm, verbose=True)
class Error_tool:
def run(self, s: str):
raise ToolException('The current search tool is not available.')
def handle_tool_error(error) -> str:
return "The following errors occurred during tool execution:"+str(error)
search_tool1 = Error_tool()
search_tool2 = SerpAPIWrapper()
tools = [
Tool.from_function(
func=search_tool1.run,
name="Search_tool1",
description="useful for when you need to answer questions about current events.You should give priority to using it.",
handle_tool_error=handle_tool_error,
),
Tool.from_function(
func=search_tool2.run,
name="Search_tool2",
description="useful for when you need to answer questions about current events",
return_direct=True,
)
]
agent = initialize_agent(tools, llm, agent=AgentType.ZERO_SHOT_REACT_DESCRIPTION, verbose=True,
handle_tool_errors=handle_tool_error)
agent.run("Who is Leo DiCaprio's girlfriend? What is her current age raised to the 0.43 power?")
```

## Who can review?
- @vowelparrot
---------
Co-authored-by: Dev 2049 <dev.dev2049@gmail.com>
# Implemented appending arbitrary messages to the base chat message
history, the in-memory and cosmos ones.
<!--
Thank you for contributing to LangChain! Your PR will appear in our next
release under the title you set. Please make sure it highlights your
valuable contribution.
Replace this with a description of the change, the issue it fixes (if
applicable), and relevant context. List any dependencies required for
this change.
After you're done, someone will review your PR. They may suggest
improvements. If no one reviews your PR within a few days, feel free to
@-mention the same people again, as notifications can get lost.
-->
As discussed this is the alternative way instead of #4480, with a
add_message method added that takes a BaseMessage as input, so that the
user can control what is in the base message like kwargs.
<!-- Remove if not applicable -->
Fixes # (issue)
## Before submitting
<!-- If you're adding a new integration, include an integration test and
an example notebook showing its use! -->
## Who can review?
Community members can review the PR once tests pass. Tag
maintainers/contributors who might be interested:
@hwchase17
---------
Co-authored-by: Harrison Chase <hw.chase.17@gmail.com>
# Fix for `update_document` Function in Chroma
## Summary
This pull request addresses an issue with the `update_document` function
in the Chroma class, as described in
[#5031](https://github.com/hwchase17/langchain/issues/5031#issuecomment-1562577947).
The issue was identified as an `AttributeError` raised when calling
`update_document` due to a missing corresponding method in the
`Collection` object. This fix refactors the `update_document` method in
`Chroma` to correctly interact with the `Collection` object.
## Changes
1. Fixed the `update_document` method in the `Chroma` class to correctly
call methods on the `Collection` object.
2. Added the corresponding test `test_chroma_update_document` in
`tests/integration_tests/vectorstores/test_chroma.py` to reflect the
updated method call.
3. Added an example and explanation of how to use the `update_document`
function in the Jupyter notebook tutorial for Chroma.
## Test Plan
All existing tests pass after this change. In addition, the
`test_chroma_update_document` test case now correctly checks the
functionality of `update_document`, ensuring that the function works as
expected and updates the content of documents correctly.
## Reviewers
@dev2049
This fix will ensure that users are able to use the `update_document`
function as expected, without encountering the previous
`AttributeError`. This will enhance the usability and reliability of the
Chroma class for all users.
Thank you for considering this pull request. I look forward to your
feedback and suggestions.
# Fix lost mimetype when using Blob.from_data method
The mimetype is lost due to a typo in the class attribue name
Fixes # - (no issue opened but I can open one if needed)
## Changes
* Fixed typo in name
* Added unit-tests to validate the output Blob
## Review
@eyurtsev
# Add path validation to DirectoryLoader
This PR introduces a minor adjustment to the DirectoryLoader by adding
validation for the path argument. Previously, if the provided path
didn't exist or wasn't a directory, DirectoryLoader would return an
empty document list due to the behavior of the `glob` method. This could
potentially cause confusion for users, as they might expect a
file-loading error instead.
So, I've added two validations to the load method of the
DirectoryLoader:
- Raise a FileNotFoundError if the provided path does not exist
- Raise a ValueError if the provided path is not a directory
Due to the relatively small scope of these changes, a new issue was not
created.
## Before submitting
<!-- If you're adding a new integration, please include:
1. a test for the integration - favor unit tests that does not rely on
network access.
2. an example notebook showing its use
See contribution guidelines for more information on how to write tests,
lint
etc:
https://github.com/hwchase17/langchain/blob/master/.github/CONTRIBUTING.md
-->
## Who can review?
Community members can review the PR once tests pass. Tag
maintainers/contributors who might be interested:
@eyurtsev
# Add SKLearnVectorStore
This PR adds SKLearnVectorStore, a simply vector store based on
NearestNeighbors implementations in the scikit-learn package. This
provides a simple drop-in vector store implementation with minimal
dependencies (scikit-learn is typically installed in a data scientist /
ml engineer environment). The vector store can be persisted and loaded
from json, bson and parquet format.
SKLearnVectorStore has soft (dynamic) dependency on the scikit-learn,
numpy and pandas packages. Persisting to bson requires the bson package,
persisting to parquet requires the pyarrow package.
## Before submitting
Integration tests are provided under
`tests/integration_tests/vectorstores/test_sklearn.py`
Sample usage notebook is provided under
`docs/modules/indexes/vectorstores/examples/sklear.ipynb`
Co-authored-by: Dev 2049 <dev.dev2049@gmail.com>
# Add Momento as a standard cache and chat message history provider
This PR adds Momento as a standard caching provider. Implements the
interface, adds integration tests, and documentation. We also add
Momento as a chat history message provider along with integration tests,
and documentation.
[Momento](https://www.gomomento.com/) is a fully serverless cache.
Similar to S3 or DynamoDB, it requires zero configuration,
infrastructure management, and is instantly available. Users sign up for
free and get 50GB of data in/out for free every month.
## Before submitting
✅ We have added documentation, notebooks, and integration tests
demonstrating usage.
Co-authored-by: Dev 2049 <dev.dev2049@gmail.com>
Add Multi-CSV/DF support in CSV and DataFrame Toolkits
* CSV and DataFrame toolkits now accept list of CSVs/DFs
* Add default prompts for many dataframes in `pandas_dataframe` toolkit
Fixes#1958
Potentially fixes#4423
## Testing
* Add single and multi-dataframe integration tests for
`pandas_dataframe` toolkit with permutations of `include_df_in_prompt`
* Add single and multi-CSV integration tests for csv toolkit
---------
Co-authored-by: Harrison Chase <hw.chase.17@gmail.com>
# Add C Transformers for GGML Models
I created Python bindings for the GGML models:
https://github.com/marella/ctransformers
Currently it supports GPT-2, GPT-J, GPT-NeoX, LLaMA, MPT, etc. See
[Supported
Models](https://github.com/marella/ctransformers#supported-models).
It provides a unified interface for all models:
```python
from langchain.llms import CTransformers
llm = CTransformers(model='/path/to/ggml-gpt-2.bin', model_type='gpt2')
print(llm('AI is going to'))
```
It can be used with models hosted on the Hugging Face Hub:
```py
llm = CTransformers(model='marella/gpt-2-ggml')
```
It supports streaming:
```py
from langchain.callbacks.streaming_stdout import StreamingStdOutCallbackHandler
llm = CTransformers(model='marella/gpt-2-ggml', callbacks=[StreamingStdOutCallbackHandler()])
```
Please see [README](https://github.com/marella/ctransformers#readme) for
more details.
---------
Co-authored-by: Dev 2049 <dev.dev2049@gmail.com>
zep-python's sync methods no longer need an asyncio wrapper. This was
causing issues with FastAPI deployment.
Zep also now supports putting and getting of arbitrary message metadata.
Bump zep-python version to v0.30
Remove nest-asyncio from Zep example notebooks.
Modify tests to include metadata.
---------
Co-authored-by: Daniel Chalef <daniel.chalef@private.org>
Co-authored-by: Daniel Chalef <131175+danielchalef@users.noreply.github.com>
# Bibtex integration
Wrap bibtexparser to retrieve a list of docs from a bibtex file.
* Get the metadata from the bibtex entries
* `page_content` get from the local pdf referenced in the `file` field
of the bibtex entry using `pymupdf`
* If no valid pdf file, `page_content` set to the `abstract` field of
the bibtex entry
* Support Zotero flavour using regex to get the file path
* Added usage example in
`docs/modules/indexes/document_loaders/examples/bibtex.ipynb`
---------
Co-authored-by: Sébastien M. Popoff <sebastien.popoff@espci.fr>
Co-authored-by: Dev 2049 <dev.dev2049@gmail.com>
# Add Joplin document loader
[Joplin](https://joplinapp.org/) is an open source note-taking app.
Joplin has a [REST API](https://joplinapp.org/api/references/rest_api/)
for accessing its local database. The proposed `JoplinLoader` uses the
API to retrieve all notes in the database and their metadata. Joplin
needs to be installed and running locally, and an access token is
required.
- The PR includes an integration test.
- The PR includes an example notebook.
---------
Co-authored-by: Dev 2049 <dev.dev2049@gmail.com>
## Description
The html structure of readthedocs can differ. Currently, the html tag is
hardcoded in the reader, and unable to fit into some cases. This pr
includes the following changes:
1. Replace `find_all` with `find` because we just want one tag.
2. Provide `custom_html_tag` to the loader.
3. Add tests for readthedoc loader
4. Refactor code
## Issues
See more in https://github.com/hwchase17/langchain/pull/2609. The
problem was not completely fixed in that pr.
---------
Signed-off-by: byhsu <byhsu@linkedin.com>
Co-authored-by: byhsu <byhsu@linkedin.com>
Co-authored-by: Dev 2049 <dev.dev2049@gmail.com>
# OpanAI finetuned model giving zero tokens cost
Very simple fix to the previously committed solution to allowing
finetuned Openai models.
Improves #5127
---------
Co-authored-by: Dev 2049 <dev.dev2049@gmail.com>
# Beam
Calls the Beam API wrapper to deploy and make subsequent calls to an
instance of the gpt2 LLM in a cloud deployment. Requires installation of
the Beam library and registration of Beam Client ID and Client Secret.
Additional calls can then be made through the instance of the large
language model in your code or by calling the Beam API.
---------
Co-authored-by: Dev 2049 <dev.dev2049@gmail.com>
# Vectara Integration
This PR provides integration with Vectara. Implemented here are:
* langchain/vectorstore/vectara.py
* tests/integration_tests/vectorstores/test_vectara.py
* langchain/retrievers/vectara_retriever.py
And two IPYNB notebooks to do more testing:
* docs/modules/chains/index_examples/vectara_text_generation.ipynb
* docs/modules/indexes/vectorstores/examples/vectara.ipynb
---------
Co-authored-by: Dev 2049 <dev.dev2049@gmail.com>
# Add MosaicML inference endpoints
This PR adds support in langchain for MosaicML inference endpoints. We
both serve a select few open source models, and allow customers to
deploy their own models using our inference service. Docs are here
(https://docs.mosaicml.com/en/latest/inference.html), and sign up form
is here (https://forms.mosaicml.com/demo?utm_source=langchain). I'm not
intimately familiar with the details of langchain, or the contribution
process, so please let me know if there is anything that needs fixing or
this is the wrong way to submit a new integration, thanks!
I'm also not sure what the procedure is for integration tests. I have
tested locally with my api key.
## Who can review?
@hwchase17
---------
Co-authored-by: Harrison Chase <hw.chase.17@gmail.com>
This PR introduces a new module, `elasticsearch_embeddings.py`, which
provides a wrapper around Elasticsearch embedding models. The new
ElasticsearchEmbeddings class allows users to generate embeddings for
documents and query texts using a [model deployed in an Elasticsearch
cluster](https://www.elastic.co/guide/en/machine-learning/current/ml-nlp-model-ref.html#ml-nlp-model-ref-text-embedding).
### Main features:
1. The ElasticsearchEmbeddings class initializes with an Elasticsearch
connection object and a model_id, providing an interface to interact
with the Elasticsearch ML client through
[infer_trained_model](https://elasticsearch-py.readthedocs.io/en/v8.7.0/api.html?highlight=trained%20model%20infer#elasticsearch.client.MlClient.infer_trained_model)
.
2. The `embed_documents()` method generates embeddings for a list of
documents, and the `embed_query()` method generates an embedding for a
single query text.
3. The class supports custom input text field names in case the deployed
model expects a different field name than the default `text_field`.
4. The implementation is compatible with any model deployed in
Elasticsearch that generates embeddings as output.
### Benefits:
1. Simplifies the process of generating embeddings using Elasticsearch
models.
2. Provides a clean and intuitive interface to interact with the
Elasticsearch ML client.
3. Allows users to easily integrate Elasticsearch-generated embeddings.
Related issue https://github.com/hwchase17/langchain/issues/3400
---------
Co-authored-by: Dev 2049 <dev.dev2049@gmail.com>
# Add AzureCognitiveServicesToolkit to call Azure Cognitive Services
API: achieve some multimodal capabilities
This PR adds a toolkit named AzureCognitiveServicesToolkit which bundles
the following tools:
- AzureCogsImageAnalysisTool: calls Azure Cognitive Services image
analysis API to extract caption, objects, tags, and text from images.
- AzureCogsFormRecognizerTool: calls Azure Cognitive Services form
recognizer API to extract text, tables, and key-value pairs from
documents.
- AzureCogsSpeech2TextTool: calls Azure Cognitive Services speech to
text API to transcribe speech to text.
- AzureCogsText2SpeechTool: calls Azure Cognitive Services text to
speech API to synthesize text to speech.
This toolkit can be used to process image, document, and audio inputs.
---------
Co-authored-by: Dev 2049 <dev.dev2049@gmail.com>
# Improve TextSplitter.split_documents, collect page_content and
metadata in one iteration
## Who can review?
Community members can review the PR once tests pass. Tag
maintainers/contributors who might be interested:
@eyurtsev In the case where documents is a generator that can only be
iterated once making this change is a huge help. Otherwise a silent
issue happens where metadata is empty for all documents when documents
is a generator. So we expand the argument from `List[Document]` to
`Union[Iterable[Document], Sequence[Document]]`
---------
Co-authored-by: Steven Tartakovsky <tartakovsky.developer@gmail.com>
Implementation is similar to search_distance and where_filter
# adds 'additional' support to Weaviate queries
Co-authored-by: Dev 2049 <dev.dev2049@gmail.com>
OpenLM is a zero-dependency OpenAI-compatible LLM provider that can call
different inference endpoints directly via HTTP. It implements the
OpenAI Completion class so that it can be used as a drop-in replacement
for the OpenAI API. This changeset utilizes BaseOpenAI for minimal added
code.
---------
Co-authored-by: Dev 2049 <dev.dev2049@gmail.com>
# Add Mastodon toots loader.
Loader works either with public toots, or Mastodon app credentials. Toot
text and user info is loaded.
I've also added integration test for this new loader as it works with
public data, and a notebook with example output run now.
---------
Co-authored-by: Dev 2049 <dev.dev2049@gmail.com>
# PowerBI major refinement in working of tool and tweaks in the rest
I've gained some experience with more complex sets and the earlier
implementation had too many tries by the agent to create DAX, so
refactored the code to run the LLM to create dax based on a question and
then immediately run the same against the dataset, with retries and a
prompt that includes the error for the retry. This works much better!
Also did some other refactoring of the inner workings, making things
clearer, more concise and faster.
# Row-wise cosine similarity between two equal-width matrices and return
the max top_k score and index, the score all greater than
threshold_score.
Co-authored-by: Dev 2049 <dev.dev2049@gmail.com>
Enhance the code to support SSL authentication for Elasticsearch when
using the VectorStore module, as previous versions did not provide this
capability.
@dev2049
---------
Co-authored-by: caidong <zhucaidong1992@gmail.com>
Co-authored-by: Dev 2049 <dev.dev2049@gmail.com>
This is a highly optimized update to the pull request
https://github.com/hwchase17/langchain/pull/3269
Summary:
1) Added ability to MRKL agent to self solve the ValueError(f"Could not
parse LLM output: `{llm_output}`") error, whenever llm (especially
gpt-3.5-turbo) does not follow the format of MRKL Agent, while returning
"Action:" & "Action Input:".
2) The way I am solving this error is by responding back to the llm with
the messages "Invalid Format: Missing 'Action:' after 'Thought:'" &
"Invalid Format: Missing 'Action Input:' after 'Action:'" whenever
Action: and Action Input: are not present in the llm output
respectively.
For a detailed explanation, look at the previous pull request.
New Updates:
1) Since @hwchase17 , requested in the previous PR to communicate the
self correction (error) message, using the OutputParserException, I have
added new ability to the OutputParserException class to store the
observation & previous llm_output in order to communicate it to the next
Agent's prompt. This is done, without breaking/modifying any of the
functionality OutputParserException previously performs (i.e.
OutputParserException can be used in the same way as before, without
passing any observation & previous llm_output too).
---------
Co-authored-by: Deepak S V <svdeepak99@users.noreply.github.com>
Update to pull request https://github.com/hwchase17/langchain/pull/3215
Summary:
1) Improved the sanitization of query (using regex), by removing python
command (since gpt-3.5-turbo sometimes assumes python console as a
terminal, and runs python command first which causes error). Also
sometimes 1 line python codes contain single backticks.
2) Added 7 new test cases.
For more details, view the previous pull request.
---------
Co-authored-by: Deepak S V <svdeepak99@users.noreply.github.com>
Let user inspect the token ids in addition to getting th enumber of tokens
---------
Co-authored-by: Zach Schillaci <40636930+zachschillaci27@users.noreply.github.com>
Extract the methods specific to running an LLM or Chain on a dataset to
separate utility functions.
This simplifies the client a bit and lets us separate concerns of LCP
details from running examples (e.g., for evals)
### Submit Multiple Files to the Unstructured API
Enables batching multiple files into a single Unstructured API requests.
Support for requests with multiple files was added to both
`UnstructuredAPIFileLoader` and `UnstructuredAPIFileIOLoader`. Note that
if you submit multiple files in "single" mode, the result will be
concatenated into a single document. We recommend using this feature in
"elements" mode.
### Testing
The following should load both documents, using two of the example docs
from the integration tests folder.
```python
from langchain.document_loaders import UnstructuredAPIFileLoader
file_paths = ["examples/layout-parser-paper.pdf", "examples/whatsapp_chat.txt"]
loader = UnstructuredAPIFileLoader(
file_paths=file_paths,
api_key="FAKE_API_KEY",
strategy="fast",
mode="elements",
)
docs = loader.load()
```
# Improve Evernote Document Loader
When exporting from Evernote you may export more than one note.
Currently the Evernote loader concatenates the content of all notes in
the export into a single document and only attaches the name of the
export file as metadata on the document.
This change ensures that each note is loaded as an independent document
and all available metadata on the note e.g. author, title, created,
updated are added as metadata on each document.
It also uses an existing optional dependency of `html2text` instead of
`pypandoc` to remove the need to download the pandoc application via
`download_pandoc()` to be able to use the `pypandoc` python bindings.
Fixes#4493
Co-authored-by: Mike McGarry <mike.mcgarry@finbourne.com>
Co-authored-by: Dev 2049 <dev.dev2049@gmail.com>
# Adds "IN" metadata filter for pgvector to all checking for set
presence
PGVector currently supports metadata filters of the form:
```
{"filter": {"key": "value"}}
```
which will return documents where the "key" metadata field is equal to
"value".
This PR adds support for metadata filters of the form:
```
{"filter": {"key": { "IN" : ["list", "of", "values"]}}}
```
Other vector stores support this via an "$in" syntax. I chose to use
"IN" to match postgres' syntax, though happy to switch.
Tested locally with PGVector and ChatVectorDBChain.
@dev2049
---------
Co-authored-by: jade@spanninglabs.com <jade@spanninglabs.com>
# Powerbi API wrapper bug fix + integration tests
- Bug fix by removing `TYPE_CHECKING` in in utilities/powerbi.py
- Added integration test for power bi api in
utilities/test_powerbi_api.py
- Added integration test for power bi agent in
agent/test_powerbi_agent.py
- Edited .env.examples to help set up power bi related environment
variables
- Updated demo notebook with working code in
docs../examples/powerbi.ipynb - AzureOpenAI -> ChatOpenAI
Notes:
Chat models (gpt3.5, gpt4) are much more capable than davinci at writing
DAX queries, so that is important to getting the agent to work properly.
Interestingly, gpt3.5-turbo needed the examples=DEFAULT_FEWSHOT_EXAMPLES
to write consistent DAX queries, so gpt4 seems necessary as the smart
llm.
Fixes#4325
## Before submitting
Azure-core and Azure-identity are necessary dependencies
check integration tests with the following:
`pytest tests/integration_tests/utilities/test_powerbi_api.py`
`pytest tests/integration_tests/agent/test_powerbi_agent.py`
You will need a power bi account with a dataset id + table name in order
to test. See .env.examples for details.
## Who can review?
@hwchase17
@vowelparrot
---------
Co-authored-by: aditya-pethe <adityapethe1@gmail.com>
# Add Spark SQL support
* Add Spark SQL support. It can connect to Spark via building a
local/remote SparkSession.
* Include a notebook example
I tried some complicated queries (window function, table joins), and the
tool works well.
Compared to the [Spark Dataframe
agent](https://python.langchain.com/en/latest/modules/agents/toolkits/examples/spark.html),
this tool is able to generate queries across multiple tables.
---------
# Your PR Title (What it does)
<!--
Thank you for contributing to LangChain! Your PR will appear in our next
release under the title you set. Please make sure it highlights your
valuable contribution.
Replace this with a description of the change, the issue it fixes (if
applicable), and relevant context. List any dependencies required for
this change.
After you're done, someone will review your PR. They may suggest
improvements. If no one reviews your PR within a few days, feel free to
@-mention the same people again, as notifications can get lost.
-->
<!-- Remove if not applicable -->
Fixes # (issue)
## Before submitting
<!-- If you're adding a new integration, include an integration test and
an example notebook showing its use! -->
## Who can review?
Community members can review the PR once tests pass. Tag
maintainers/contributors who might be interested:
<!-- For a quicker response, figure out the right person to tag with @
@hwchase17 - project lead
Tracing / Callbacks
- @agola11
Async
- @agola11
DataLoaders
- @eyurtsev
Models
- @hwchase17
- @agola11
Agents / Tools / Toolkits
- @vowelparrot
VectorStores / Retrievers / Memory
- @dev2049
-->
---------
Co-authored-by: Gengliang Wang <gengliang@apache.org>
Co-authored-by: Mike W <62768671+skcoirz@users.noreply.github.com>
Co-authored-by: Eugene Yurtsev <eyurtsev@gmail.com>
Co-authored-by: UmerHA <40663591+UmerHA@users.noreply.github.com>
Co-authored-by: 张城铭 <z@hyperf.io>
Co-authored-by: assert <zhangchengming@kkguan.com>
Co-authored-by: blob42 <spike@w530>
Co-authored-by: Yuekai Zhang <zhangyuekai@foxmail.com>
Co-authored-by: Richard He <he.yucheng@outlook.com>
Co-authored-by: Dev 2049 <dev.dev2049@gmail.com>
Co-authored-by: Leonid Ganeline <leo.gan.57@gmail.com>
Co-authored-by: Alexey Nominas <60900649+Chae4ek@users.noreply.github.com>
Co-authored-by: elBarkey <elbarkey@gmail.com>
Co-authored-by: Davis Chase <130488702+dev2049@users.noreply.github.com>
Co-authored-by: Jeffrey D <1289344+verygoodsoftwarenotvirus@users.noreply.github.com>
Co-authored-by: so2liu <yangliu35@outlook.com>
Co-authored-by: Viswanadh Rayavarapu <44315599+vishwa-rn@users.noreply.github.com>
Co-authored-by: Chakib Ben Ziane <contact@blob42.xyz>
Co-authored-by: Daniel Chalef <131175+danielchalef@users.noreply.github.com>
Co-authored-by: Daniel Chalef <daniel.chalef@private.org>
Co-authored-by: Jari Bakken <jari.bakken@gmail.com>
Co-authored-by: escafati <scafatieugenio@gmail.com>
# Zep Retriever - Vector Search Over Chat History with the Zep Long-term
Memory Service
More on Zep: https://github.com/getzep/zep
Note: This PR is related to and relies on
https://github.com/hwchase17/langchain/pull/4834. I did not want to
modify the `pyproject.toml` file to add the `zep-python` dependency a
second time.
Co-authored-by: Daniel Chalef <daniel.chalef@private.org>
# TextLoader auto detect encoding and enhanced exception handling
- Add an option to enable encoding detection on `TextLoader`.
- The detection is done using `chardet`
- The loading is done by trying all detected encodings by order of
confidence or raise an exception otherwise.
### New Dependencies:
- `chardet`
Fixes#4479
## Before submitting
<!-- If you're adding a new integration, include an integration test and
an example notebook showing its use! -->
## Who can review?
Community members can review the PR once tests pass. Tag
maintainers/contributors who might be interested:
- @eyurtsev
---------
Co-authored-by: blob42 <spike@w530>
# Add bs4 html parser
* Some minor refactors
* Extract the bs4 html parsing code from the bs html loader
* Move some tests from integration tests to unit tests
# Add generic document loader
* This PR adds a generic document loader which can assemble a loader
from a blob loader and a parser
* Adds a registry for parsers
* Populate registry with a default mimetype based parser
## Expected changes
- Parsing involves loading content via IO so can be sped up via:
* Threading in sync
* Async
- The actual parsing logic may be computatinoally involved: may need to
figure out to add multi-processing support
- May want to add suffix based parser since suffixes are easier to
specify in comparison to mime types
## Before submitting
No notebooks yet, we first need to get a few of the basic parsers up
(prior to advertising the interface)
# Remove unnecessary comment
Remove unnecessary comment accidentally included in #4800
## Before submitting
- no test
- no document
## Who can review?
Community members can review the PR once tests pass. Tag
maintainers/contributors who might be interested:
Previously, the client expected a strict 'prompt' or 'messages' format
and wouldn't permit running a chat model or llm on prompts or messages
(respectively).
Since many datasets may want to specify custom key: string , relax this
requirement.
Also, add support for running a chat model on raw prompts and LLM on
chat messages through their respective fallbacks.
# Your PR Title (What it does)
<!--
Thank you for contributing to LangChain! Your PR will appear in our next
release under the title you set. Please make sure it highlights your
valuable contribution.
Replace this with a description of the change, the issue it fixes (if
applicable), and relevant context. List any dependencies required for
this change.
After you're done, someone will review your PR. They may suggest
improvements. If no one reviews your PR within a few days, feel free to
@-mention the same people again, as notifications can get lost.
-->
<!-- Remove if not applicable -->
Fixes # (issue)
## Before submitting
<!-- If you're adding a new integration, include an integration test and
an example notebook showing its use! -->
## Who can review?
Community members can review the PR once tests pass. Tag
maintainers/contributors who might be interested:
<!-- For a quicker response, figure out the right person to tag with @
@hwchase17 - project lead
Tracing / Callbacks
- @agola11
Async
- @agola11
DataLoaders
- @eyurtsev
Models
- @hwchase17
- @agola11
Agents / Tools / Toolkits
- @vowelparrot
VectorStores / Retrievers / Memory
- @dev2049
-->
**Feature**: This PR adds `from_template_file` class method to
BaseStringMessagePromptTemplate. This is useful to help user to create
message prompt templates directly from template files, including
`ChatMessagePromptTemplate`, `HumanMessagePromptTemplate`,
`AIMessagePromptTemplate` & `SystemMessagePromptTemplate`.
**Tests**: Unit tests have been added in this PR.
Co-authored-by: charosen <charosen@bupt.cn>
Co-authored-by: Dev 2049 <dev.dev2049@gmail.com>
Adds some basic unit tests for the ConfluenceLoader that can be extended
later. Ports this [PR from
llama-hub](https://github.com/emptycrown/llama-hub/pull/208) and adapts
it to `langchain`.
@Jflick58 and @zywilliamli adding you here as potential reviewers
---------
Co-authored-by: Dev 2049 <dev.dev2049@gmail.com>
# Improve the Chroma get() method by adding the optional "include"
parameter.
The Chroma get() method excludes embeddings by default. You can
customize the response by specifying the "include" parameter to
selectively retrieve the desired data from the collection.
---------
Co-authored-by: Dev 2049 <dev.dev2049@gmail.com>
# Fix Telegram API loader + add tests.
I was testing this integration and it was broken with next error:
```python
message_threads = loader._get_message_threads(df)
KeyError: False
```
Also, this particular loader didn't have any tests / related group in
poetry, so I added those as well.
@hwchase17 / @eyurtsev please take a look on this fix PR.
---------
Co-authored-by: Dev 2049 <dev.dev2049@gmail.com>
# Cassandra support for chat history
### Description
- Store chat messages in cassandra
### Dependency
- cassandra-driver - Python Module
## Before submitting
- Added Integration Test
## Who can review?
@hwchase17
@agola11
# Your PR Title (What it does)
<!--
Thank you for contributing to LangChain! Your PR will appear in our next
release under the title you set. Please make sure it highlights your
valuable contribution.
Replace this with a description of the change, the issue it fixes (if
applicable), and relevant context. List any dependencies required for
this change.
After you're done, someone will review your PR. They may suggest
improvements. If no one reviews your PR within a few days, feel free to
@-mention the same people again, as notifications can get lost.
-->
<!-- Remove if not applicable -->
Fixes # (issue)
## Before submitting
<!-- If you're adding a new integration, include an integration test and
an example notebook showing its use! -->
## Who can review?
Community members can review the PR once tests pass. Tag
maintainers/contributors who might be interested:
<!-- For a quicker response, figure out the right person to tag with @
@hwchase17 - project lead
Tracing / Callbacks
- @agola11
Async
- @agola11
DataLoaders
- @eyurtsev
Models
- @hwchase17
- @agola11
Agents / Tools / Toolkits
- @vowelparrot
VectorStores / Retrievers / Memory
- @dev2049
-->
Co-authored-by: Jinto Jose <129657162+jj701@users.noreply.github.com>
# Fix DeepLake Overwrite Flag Issue
Fixes Issue #4682: essentially, setting overwrite to False in the
DeepLake constructor still triggers an overwrite, because the logic is
just checking for the presence of "overwrite" in kwargs. The fix is
simple--just add some checks to inspect if "overwrite" in kwargs AND
kwargs["overwrite"]==True.
Added a new test in
tests/integration_tests/vectorstores/test_deeplake.py to reflect the
desired behavior.
Co-authored-by: Anirudh Suresh <ani@Anirudhs-MBP.cable.rcn.com>
Co-authored-by: Anirudh Suresh <ani@Anirudhs-MacBook-Pro.local>
Co-authored-by: Dev 2049 <dev.dev2049@gmail.com>
# Add summarization task type for HuggingFace APIs
Add summarization task type for HuggingFace APIs.
This task type is described by [HuggingFace inference
API](https://huggingface.co/docs/api-inference/detailed_parameters#summarization-task)
My project utilizes LangChain to connect multiple LLMs, including
various HuggingFace models that support the summarization task.
Integrating this task type is highly convenient and beneficial.
Fixes#4720
# Add GraphQL Query Support
This PR introduces a GraphQL API Wrapper tool that allows LLM agents to
query GraphQL databases. The tool utilizes the httpx and gql Python
packages to interact with GraphQL APIs and provides a simple interface
for running queries with LLM agents.
@vowelparrot
---------
Co-authored-by: Dev 2049 <dev.dev2049@gmail.com>
### Adds a document loader for Docugami
Specifically:
1. Adds a data loader that talks to the [Docugami](http://docugami.com)
API to download processed documents as semantic XML
2. Parses the semantic XML into chunks, with additional metadata
capturing chunk semantics
3. Adds a detailed notebook showing how you can use additional metadata
returned by Docugami for techniques like the [self-querying
retriever](https://python.langchain.com/en/latest/modules/indexes/retrievers/examples/self_query_retriever.html)
4. Adds an integration test, and related documentation
Here is an example of a result that is not possible without the
capabilities added by Docugami (from the notebook):
<img width="1585" alt="image"
src="https://github.com/hwchase17/langchain/assets/749277/bb6c1ce3-13dc-4349-a53b-de16681fdd5b">
---------
Co-authored-by: Taqi Jaffri <tjaffri@docugami.com>
Co-authored-by: Taqi Jaffri <tjaffri@gmail.com>
# Improve video_id extraction in `YoutubeLoader`
`YoutubeLoader.from_youtube_url` can only deal with one specific url
format. I've introduced `YoutubeLoader.extract_video_id` which can
extract video id from common YT urls.
Fixes#4451
@eyurtsev
---------
Co-authored-by: Kamil Niski <kamil.niski@gmail.com>
# Respect User-Specified User-Agent in WebBaseLoader
This pull request modifies the `WebBaseLoader` class initializer from
the `langchain.document_loaders.web_base` module to preserve any
User-Agent specified by the user in the `header_template` parameter.
Previously, even if a User-Agent was specified in `header_template`, it
would always be overridden by a random User-Agent generated by the
`fake_useragent` library.
With this change, if a User-Agent is specified in `header_template`, it
will be used. Only in the case where no User-Agent is specified will a
random User-Agent be generated and used. This provides additional
flexibility when using the `WebBaseLoader` class, allowing users to
specify their own User-Agent if they have a specific need or preference,
while still providing a reasonable default for cases where no User-Agent
is specified.
This change has no impact on existing users who do not specify a
User-Agent, as the behavior in this case remains the same. However, for
users who do specify a User-Agent, their choice will now be respected
and used for all subsequent requests made using the `WebBaseLoader`
class.
Fixes#4167
## Before submitting
============================= test session starts
==============================
collecting ... collected 1 item
test_web_base.py::TestWebBaseLoader::test_respect_user_specified_user_agent
============================== 1 passed in 3.64s
===============================
PASSED [100%]
## Who can review?
Community members can review the PR once tests pass. Tag
maintainers/contributors who might be interested: @eyurtsev
---------
Co-authored-by: Eugene Yurtsev <eyurtsev@gmail.com>
[OpenWeatherMapAPIWrapper](f70e18a5b3/docs/modules/agents/tools/examples/openweathermap.ipynb)
works wonderfully, but the _tool_ itself can't be used in master branch.
- added OpenWeatherMap **tool** to the public api, to be loadable with
`load_tools` by using "openweathermap-api" tool name (that name is used
in the existing
[docs](aff33d52c5/docs/modules/agents/tools/getting_started.md),
at the bottom of the page)
- updated OpenWeatherMap tool's **description** to make the input format
match what the API expects (e.g. `London,GB` instead of `'London,GB'`)
- added [ecosystem documentation page for
OpenWeatherMap](f9c41594fe/docs/ecosystem/openweathermap.md)
- added tool usage example to [OpenWeatherMap's
notebook](f9c41594fe/docs/modules/agents/tools/examples/openweathermap.ipynb)
Let me know if there's something I missed or something needs to be
updated! Or feel free to make edits yourself if that makes it easier for
you 🙂
Currently, all Zapier tools are built using the pre-written base Zapier
prompt. These small changes (that retain default behavior) will allow a
user to create a Zapier tool using the ZapierNLARunTool while providing
their own base prompt.
Their prompt must contain input fields for zapier_description and
params, checked and enforced in the tool's root validator.
An example of when this may be useful: user has several, say 10, Zapier
tools enabled. Currently, the long generic default Zapier base prompt is
attached to every single tool, using an extreme number of tokens for no
real added benefit (repeated). User prompts LLM on how to use Zapier
tools once, then overrides the base prompt.
Or: user has a few specific Zapier tools and wants to maximize their
success rate. So, user writes prompts/descriptions for those tools
specific to their use case, and provides those to the ZapierNLARunTool.
A consideration - this is the simplest way to implement this I could
think of... though ideally custom prompting would be possible at the
Toolkit level as well. For now, this should be sufficient in solving the
concerns outlined above.
The error in #4087 was happening because of the use of csv.Dialect.*
which is just an empty base class. we need to make a choice on what is
our base dialect. I usually use excel so I put it as excel, if
maintainers have other preferences do let me know.
Open Questions:
1. What should be the default dialect?
2. Should we rework all tests to mock the open function rather than the
csv.DictReader?
3. Should we make a separate input for `dialect` like we have for
`encoding`?
---------
Co-authored-by: = <=>
### Refactor the BaseTracer
- Remove the 'session' abstraction from the BaseTracer
- Rename 'RunV2' object(s) to be called 'Run' objects (Rename previous
Run objects to be RunV1 objects)
- Ditto for sessions: TracerSession*V2 -> TracerSession*
- Remove now deprecated conversion from v1 run objects to v2 run objects
in LangChainTracerV2
- Add conversion from v2 run objects to v1 run objects in V1 tracer
## Change Chain argument in client to accept a chain factory
The `run_over_dataset` functionality seeks to treat each iteration of an
example as an independent trial.
Chains have memory, so it's easier to permit this type of behavior if we
accept a factory method rather than the chain object directly.
There's still corner cases / UX pains people will likely run into, like:
- Caching may cause issues
- if memory is persisted to a shared object (e.g., same redis queue) ,
this could impact what is retrieved
- If we're running the async methods with concurrency using local
models, if someone naively instantiates the chain and loads each time,
it could lead to tons of disk I/O or OOM
# Adds testing options to pytest
This PR adds the following options:
* `--only-core` will skip all extended tests, running all core tests.
* `--only-extended` will skip all core tests. Forcing alll extended
tests to be run.
Running `py.test` without specifying either option will remain
unaffected. Run
all tests that can be run within the unit_tests direction. Extended
tests will
run if required packages are installed.
## Before submitting
## Who can review?
### Add Invocation Params to Logged Run
Adds an llm type to each chat model as well as an override of the dict()
method to log the invocation parameters for each call
---------
Co-authored-by: Ankush Gola <ankush.gola@gmail.com>
### Add on_chat_message_start to callback manager and base tracer
Goal: trace messages directly to permit reloading as chat messages
(store in an integration-agnostic way)
Add an `on_chat_message_start` method. Fall back to `on_llm_start()` for
handlers that don't have it implemented.
Does so in a non-backwards-compat breaking way (for now)
# Parameterize Redis vectorstore index
Redis vectorstore allows for three different distance metrics: `L2`
(flat L2), `COSINE`, and `IP` (inner product). Currently, the
`Redis._create_index` method hard codes the distance metric to COSINE.
I've parameterized this as an argument in the `Redis.from_texts` method
-- pretty simple.
Fixes#4368
## Before submitting
I've added an integration test showing indexes can be instantiated with
all three values in the `REDIS_DISTANCE_METRICS` literal. An example
notebook seemed overkill here. Normal API documentation would be more
appropriate, but no standards are in place for that yet.
## Who can review?
Not sure who's responsible for the vectorstore module... Maybe @eyurtsev
/ @hwchase17 / @agola11 ?
# Add option to `load_huggingface_tool`
Expose a method to load a huggingface Tool from the HF hub
---------
Co-authored-by: Dev 2049 <dev.dev2049@gmail.com>
Thanks to @anna-charlotte and @jupyterjazz for the contribution! Made
few small changes to get it across the finish line
---------
Signed-off-by: anna-charlotte <charlotte.gerhaher@jina.ai>
Signed-off-by: jupyterjazz <saba.sturua@jina.ai>
Co-authored-by: anna-charlotte <charlotte.gerhaher@jina.ai>
Co-authored-by: jupyterjazz <saba.sturua@jina.ai>
Co-authored-by: Saba Sturua <45267439+jupyterjazz@users.noreply.github.com>
# Add action to test with all dependencies installed
PR adds a custom action for setting up poetry that allows specifying a
cache key:
https://github.com/actions/setup-python/issues/505#issuecomment-1273013236
This makes it possible to run 2 types of unit tests:
(1) unit tests with only core dependencies
(2) unit tests with extended dependencies (e.g., those that rely on an
optional pdf parsing library)
As part of this PR, we're moving some pdf parsing tests into the
unit-tests section and making sure that these unit tests get executed
when running with extended dependencies.
# ODF File Loader
Adds a data loader for handling Open Office ODT files. Requires
`unstructured>=0.6.3`.
### Testing
The following should work using the `fake.odt` example doc from the
[`unstructured` repo](https://github.com/Unstructured-IO/unstructured).
```python
from langchain.document_loaders import UnstructuredODTLoader
loader = UnstructuredODTLoader(file_path="fake.odt", mode="elements")
loader.load()
loader = UnstructuredODTLoader(file_path="fake.odt", mode="single")
loader.load()
```
Fixes#4153
If the sender of a message in a group chat isn't in your contact list,
they will appear with a ~ prefix in the exported chat. This PR adds
support for parsing such lines.