See PR title.
From what I can see, `poetry` will auto-include this. Please let me know
if I am missing something here.
---------
Co-authored-by: Bagatur <baskaryan@gmail.com>
CC @baskaryan @hwchase17 @jmorganca
Having a bit of trouble importing `langchain_experimental` from a
notebook, will figure it out tomorrow
~Ah and also is blocked by #13226~
---------
Co-authored-by: Lance Martin <lance@langchain.dev>
Co-authored-by: Bagatur <baskaryan@gmail.com>
It was :
`from langchain.schema.prompts import BasePromptTemplate`
but because of the breaking change in the ns, it is now
`from langchain.schema.prompt_template import BasePromptTemplate`
This bug prevents building the API Reference for the langchain_experimental
- **Description:** Updates to `AnthropicFunctions` to be compatible with
the OpenAI `function_call` functionality.
- **Issue:** The functionality to indicate `auto`, `none` and a forced
function_call was not completely implemented in the existing code.
- **Dependencies:** None
- **Tag maintainer:** @baskaryan , and any of the other maintainers if
needed.
- **Twitter handle:** None
I have specifically tested this functionality via AWS Bedrock with the
Claude-2 and Claude-Instant models.
- **Description:** Existing model used for Prompt Injection is quite
outdated but we fine-tuned and open-source a new model based on the same
model deberta-v3-base from Microsoft -
[laiyer/deberta-v3-base-prompt-injection](https://huggingface.co/laiyer/deberta-v3-base-prompt-injection).
It supports more up-to-date injections and less prone to
false-positives.
- **Dependencies:** No
- **Tag maintainer:** -
- **Twitter handle:** @alex_yaremchuk
---------
Co-authored-by: Bagatur <baskaryan@gmail.com>
- **Description:** The experimental package needs to be compatible with
the usage of importing agents
For example, if i use `from langchain.agents import
create_pandas_dataframe_agent`, running the program will prompt the
following information:
```
Traceback (most recent call last):
File "/Users/dongwm/test/main.py", line 1, in <module>
from langchain.agents import create_pandas_dataframe_agent
File "/Users/dongwm/test/venv/lib/python3.11/site-packages/langchain/agents/__init__.py", line 87, in __getattr__
raise ImportError(
ImportError: create_pandas_dataframe_agent has been moved to langchain experimental. See https://github.com/langchain-ai/langchain/discussions/11680 for more information.
Please update your import statement from: `langchain.agents.create_pandas_dataframe_agent` to `langchain_experimental.agents.create_pandas_dataframe_agent`.
```
But when I changed to `from langchain_experimental.agents import
create_pandas_dataframe_agent`, it was actually wrong:
```python
Traceback (most recent call last):
File "/Users/dongwm/test/main.py", line 2, in <module>
from langchain_experimental.agents import create_pandas_dataframe_agent
ImportError: cannot import name 'create_pandas_dataframe_agent' from 'langchain_experimental.agents' (/Users/dongwm/test/venv/lib/python3.11/site-packages/langchain_experimental/agents/__init__.py)
```
I should use `from langchain_experimental.agents.agent_toolkits import
create_pandas_dataframe_agent`. In order to solve the problem and make
it compatible, I added additional import code to the
langchain_experimental package. Now it can be like this Used `from
langchain_experimental.agents import create_pandas_dataframe_agent`
- **Twitter handle:** [lin_bob57617](https://twitter.com/lin_bob57617)
Fix some circular deps:
- move PromptValue into top level module bc both PromptTemplates and
OutputParsers import
- move tracer context vars to `tracers.context` and import them in
functions in `callbacks.manager`
- add core import tests
<!-- Thank you for contributing to LangChain!
Replace this entire comment with:
- **Description:** a description of the change,
- **Issue:** the issue # it fixes (if applicable),
- **Dependencies:** any dependencies required for this change,
- **Tag maintainer:** for a quicker response, tag the relevant
maintainer (see below),
- **Twitter handle:** we announce bigger features on Twitter. If your PR
gets announced, and you'd like a mention, we'll gladly shout you out!
Please make sure your PR is passing linting and testing before
submitting. Run `make format`, `make lint` and `make test` to check this
locally.
See contribution guidelines for more information on how to write/run
tests, lint, etc:
https://github.com/langchain-ai/langchain/blob/master/.github/CONTRIBUTING.md
If you're adding a new integration, please include:
1. a test for the integration, preferably unit tests that do not rely on
network access,
2. an example notebook showing its use. It lives in `docs/extras`
directory.
If no one reviews your PR within a few days, please @-mention one of
@baskaryan, @eyurtsev, @hwchase17.
-->
## Update 2023-09-08
This PR now supports further models in addition to Lllama-2 chat models.
See [this comment](#issuecomment-1668988543) for further details. The
title of this PR has been updated accordingly.
## Original PR description
This PR adds a generic `Llama2Chat` model, a wrapper for LLMs able to
serve Llama-2 chat models (like `LlamaCPP`,
`HuggingFaceTextGenInference`, ...). It implements `BaseChatModel`,
converts a list of chat messages into the [required Llama-2 chat prompt
format](https://huggingface.co/blog/llama2#how-to-prompt-llama-2) and
forwards the formatted prompt as `str` to the wrapped `LLM`. Usage
example:
```python
# uses a locally hosted Llama2 chat model
llm = HuggingFaceTextGenInference(
inference_server_url="http://127.0.0.1:8080/",
max_new_tokens=512,
top_k=50,
temperature=0.1,
repetition_penalty=1.03,
)
# Wrap llm to support Llama2 chat prompt format.
# Resulting model is a chat model
model = Llama2Chat(llm=llm)
messages = [
SystemMessage(content="You are a helpful assistant."),
MessagesPlaceholder(variable_name="chat_history"),
HumanMessagePromptTemplate.from_template("{text}"),
]
prompt = ChatPromptTemplate.from_messages(messages)
memory = ConversationBufferMemory(memory_key="chat_history", return_messages=True)
chain = LLMChain(llm=model, prompt=prompt, memory=memory)
# use chat model in a conversation
# ...
```
Also part of this PR are tests and a demo notebook.
- Tag maintainer: @hwchase17
- Twitter handle: `@mrt1nz`
---------
Co-authored-by: Erick Friis <erick@langchain.dev>
## Description
This PR adds support for
[lm-format-enforcer](https://github.com/noamgat/lm-format-enforcer) to
LangChain.
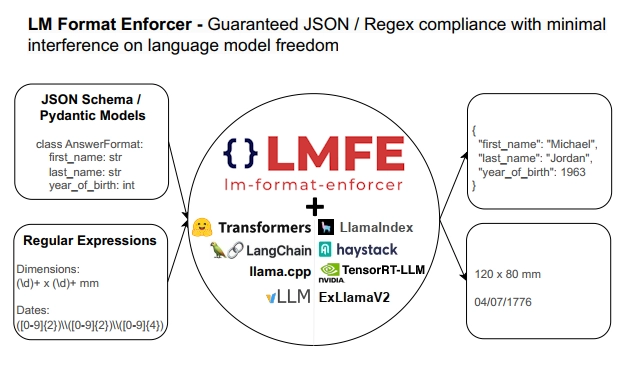
The library is similar to jsonformer / RELLM which are supported in
Langchain, but has several advantages such as
- Batching and Beam search support
- More complete JSON Schema support
- LLM has control over whitespace, improving quality
- Better runtime performance due to only calling the LLM's generate()
function once per generate() call.
The integration is loosely based on the jsonformer integration in terms
of project structure.
## Dependencies
No compile-time dependency was added, but if `lm-format-enforcer` is not
installed, a runtime error will occur if it is trying to be used.
## Tests
Due to the integration modifying the internal parameters of the
underlying huggingface transformer LLM, it is not possible to test
without building a real LM, which requires internet access. So, similar
to the jsonformer and RELLM integrations, the testing is via the
notebook.
## Twitter Handle
[@noamgat](https://twitter.com/noamgat)
Looking forward to hearing feedback!
---------
Co-authored-by: Bagatur <baskaryan@gmail.com>
Best to review one commit at a time, since two of the commits are 100%
autogenerated changes from running `ruff format`:
- Install and use `ruff format` instead of black for code formatting.
- Output of `ruff format .` in the `langchain` package.
- Use `ruff format` in experimental package.
- Format changes in experimental package by `ruff format`.
- Manual formatting fixes to make `ruff .` pass.
This PR replaces the previous `Intent` check with the new `Prompt
Safety` check. The logic and steps to enable chain moderation via the
Amazon Comprehend service, allowing you to detect and redact PII, Toxic,
and Prompt Safety information in the LLM prompt or answer remains
unchanged.
This implementation updates the code and configuration types with
respect to `Prompt Safety`.
### Usage sample
```python
from langchain_experimental.comprehend_moderation import (BaseModerationConfig,
ModerationPromptSafetyConfig,
ModerationPiiConfig,
ModerationToxicityConfig
)
pii_config = ModerationPiiConfig(
labels=["SSN"],
redact=True,
mask_character="X"
)
toxicity_config = ModerationToxicityConfig(
threshold=0.5
)
prompt_safety_config = ModerationPromptSafetyConfig(
threshold=0.5
)
moderation_config = BaseModerationConfig(
filters=[pii_config, toxicity_config, prompt_safety_config]
)
comp_moderation_with_config = AmazonComprehendModerationChain(
moderation_config=moderation_config, #specify the configuration
client=comprehend_client, #optionally pass the Boto3 Client
verbose=True
)
template = """Question: {question}
Answer:"""
prompt = PromptTemplate(template=template, input_variables=["question"])
responses = [
"Final Answer: A credit card number looks like 1289-2321-1123-2387. A fake SSN number looks like 323-22-9980. John Doe's phone number is (999)253-9876.",
"Final Answer: This is a really shitty way of constructing a birdhouse. This is fucking insane to think that any birds would actually create their motherfucking nests here."
]
llm = FakeListLLM(responses=responses)
llm_chain = LLMChain(prompt=prompt, llm=llm)
chain = (
prompt
| comp_moderation_with_config
| {llm_chain.input_keys[0]: lambda x: x['output'] }
| llm_chain
| { "input": lambda x: x['text'] }
| comp_moderation_with_config
)
try:
response = chain.invoke({"question": "A sample SSN number looks like this 123-456-7890. Can you give me some more samples?"})
except Exception as e:
print(str(e))
else:
print(response['output'])
```
### Output
```python
> Entering new AmazonComprehendModerationChain chain...
Running AmazonComprehendModerationChain...
Running pii Validation...
Running toxicity Validation...
Running prompt safety Validation...
> Finished chain.
> Entering new AmazonComprehendModerationChain chain...
Running AmazonComprehendModerationChain...
Running pii Validation...
Running toxicity Validation...
Running prompt safety Validation...
> Finished chain.
Final Answer: A credit card number looks like 1289-2321-1123-2387. A fake SSN number looks like XXXXXXXXXXXX John Doe's phone number is (999)253-9876.
```
---------
Co-authored-by: Jha <nikjha@amazon.com>
Co-authored-by: Anjan Biswas <anjanavb@amazon.com>
Co-authored-by: Anjan Biswas <84933469+anjanvb@users.noreply.github.com>
Type hinting `*args` as `List[Any]` means that each positional argument
should be a list. Type hinting `**kwargs` as `Dict[str, Any]` means that
each keyword argument should be a dict of strings.
This is almost never what we actually wanted, and doesn't seem to be
what we want in any of the cases I'm replacing here.
Minor lint dependency version upgrade to pick up latest functionality.
Ruff's new v0.1 version comes with lots of nice features, like
fix-safety guarantees and a preview mode for not-yet-stable features:
https://astral.sh/blog/ruff-v0.1.0