# Introduces embaas document extraction api endpoints
In this PR, we add support for embaas document extraction endpoints to
Text Embedding Models (with LLMs, in different PRs coming). We currently
offer the MTEB leaderboard top performers, will continue to add top
embedding models and soon add support for customers to deploy thier own
models. Additional Documentation + Infomation can be found
[here](https://embaas.io).
While developing this integration, I closely followed the patterns
established by other langchain integrations. Nonetheless, if there are
any aspects that require adjustments or if there's a better way to
present a new integration, let me know! :)
Additionally, I fixed some docs in the embeddings integration.
Related PR: #5976
#### Who can review?
DataLoaders
- @eyurtsev
Adds a new parameter `relative_chunk_overlap` for the
`SentenceTransformersTokenTextSplitter` constructor. The parameter sets
the chunk overlap using a relative factor, e.g. for a model where the
token limit is 100, a `relative_chunk_overlap=0.5` implies that
`chunk_overlap=50`
Tag maintainers/contributors who might be interested:
@hwchase17, @dev2049
#### What I do
Adding embedding api for
[DashScope](https://help.aliyun.com/product/610100.html), which is the
DAMO Academy's multilingual text unified vector model based on the LLM
base. It caters to multiple mainstream languages worldwide and offers
high-quality vector services, helping developers quickly transform text
data into high-quality vector data. Currently supported languages
include Chinese, English, Spanish, French, Portuguese, Indonesian, and
more.
#### Who can review?
Models
- @hwchase17
- @agola11
---------
Co-authored-by: Harrison Chase <hw.chase.17@gmail.com>
Inspired by the filtering capability available in ChromaDB, added the
same functionality to the FAISS vectorestore as well. Since FAISS does
not have an inbuilt method of filtering used the approach suggested in
this [thread](https://github.com/facebookresearch/faiss/issues/1079)
Langchain Issue inspiration:
https://github.com/hwchase17/langchain/issues/4572
- [x] Added filtering capability to semantic similarly and MMR
- [x] Added test cases for filtering in
`tests/integration_tests/vectorstores/test_faiss.py`
#### Who can review?
Tag maintainers/contributors who might be interested:
VectorStores / Retrievers / Memory
- @dev2049
- @hwchase17
Fixes (not reported) an error that may occur in some cases in the
RecursiveCharacterTextSplitter.
An empty `new_separators` array ([]) would end up in the else path of
the condition below and used in a function where it is expected to be
non empty.
```python
if new_separators is None:
...
else:
# _split_text() expects this array to be non-empty!
other_info = self._split_text(s, new_separators)
```
resulting in an `IndexError`
```python
def _split_text(self, text: str, separators: List[str]) -> List[str]:
"""Split incoming text and return chunks."""
final_chunks = []
# Get appropriate separator to use
> separator = separators[-1]
E IndexError: list index out of range
langchain/text_splitter.py:425: IndexError
```
#### Who can review?
@hwchase17 @eyurtsev
---------
Co-authored-by: Harrison Chase <hw.chase.17@gmail.com>
This PR updates the Vectara integration (@hwchase17 ):
* Adds reuse of requests.session to imrpove efficiency and speed.
* Utilizes Vectara's low-level API (instead of standard API) to better
match user's specific chunking with LangChain
* Now add_texts puts all the texts into a single Vectara document so
indexing is much faster.
* updated variables names from alpha to lambda_val (to be consistent
with Vectara docs) and added n_context_sentence so it's available to use
if needed.
* Updates to documentation and tests
---------
Co-authored-by: Harrison Chase <hw.chase.17@gmail.com>
# Unstructured XML Loader
Adds an `UnstructuredXMLLoader` class for .xml files. Works with
unstructured>=0.6.7. A plain text representation of the text with the
XML tags will be available under the `page_content` attribute in the
doc.
### Testing
```python
from langchain.document_loaders import UnstructuredXMLLoader
loader = UnstructuredXMLLoader(
"example_data/factbook.xml",
)
docs = loader.load()
```
## Who can review?
@hwchase17
@eyurtsev
Added AwaDB vector store, which is a wrapper over the AwaDB, that can be
used as a vector storage and has an efficient similarity search. Added
integration tests for the vector store
Added jupyter notebook with the example
Delete a unneeded empty file and resolve the
conflict(https://github.com/hwchase17/langchain/pull/5886)
Please check, Thanks!
@dev2049
@hwchase17
---------
<!--
Thank you for contributing to LangChain! Your PR will appear in our
release under the title you set. Please make sure it highlights your
valuable contribution.
Replace this with a description of the change, the issue it fixes (if
applicable), and relevant context. List any dependencies required for
this change.
After you're done, someone will review your PR. They may suggest
improvements. If no one reviews your PR within a few days, feel free to
@-mention the same people again, as notifications can get lost.
Finally, we'd love to show appreciation for your contribution - if you'd
like us to shout you out on Twitter, please also include your handle!
-->
<!-- Remove if not applicable -->
Fixes # (issue)
#### Before submitting
<!-- If you're adding a new integration, please include:
1. a test for the integration - favor unit tests that does not rely on
network access.
2. an example notebook showing its use
See contribution guidelines for more information on how to write tests,
lint
etc:
https://github.com/hwchase17/langchain/blob/master/.github/CONTRIBUTING.md
-->
#### Who can review?
Tag maintainers/contributors who might be interested:
<!-- For a quicker response, figure out the right person to tag with @
@hwchase17 - project lead
Tracing / Callbacks
- @agola11
Async
- @agola11
DataLoaders
- @eyurtsev
Models
- @hwchase17
- @agola11
Agents / Tools / Toolkits
- @vowelparrot
VectorStores / Retrievers / Memory
- @dev2049
-->
---------
Co-authored-by: ljeagle <vincent_jieli@yeah.net>
Co-authored-by: vincent <awadb.vincent@gmail.com>
Based on the inspiration from the SQL chain, the following three
parameters are added to Graph Cypher Chain.
- top_k: Limited the number of results from the database to be used as
context
- return_direct: Return database results without transforming them to
natural language
- return_intermediate_steps: Return intermediate steps
"One Retriever to merge them all, One Retriever to expose them, One
Retriever to bring them all and in and process them with Document
formatters."
Hi @dev2049! Here bothering people again!
I'm using this simple idea to deal with merging the output of several
retrievers into one.
I'm aware of DocumentCompressorPipeline and
ContextualCompressionRetriever but I don't think they allow us to do
something like this. Also I was getting in trouble to get the pipeline
working too. Please correct me if i'm wrong.
This allow to do some sort of "retrieval" preprocessing and then using
the retrieval with the curated results anywhere you could use a
retriever.
My use case is to generate diff indexes with diff embeddings and sources
for a more colorful results then filtering them with one or many
document formatters.
I saw some people looking for something like this, here:
https://github.com/hwchase17/langchain/issues/3991
and something similar here:
https://github.com/hwchase17/langchain/issues/5555
This is just a proposal I know I'm missing tests , etc. If you think
this is a worth it idea I can work on tests and anything you want to
change.
Let me know!
---------
Co-authored-by: Harrison Chase <hw.chase.17@gmail.com>
<!--
Thank you for contributing to LangChain! Your PR will appear in our
release under the title you set. Please make sure it highlights your
valuable contribution.
Replace this with a description of the change, the issue it fixes (if
applicable), and relevant context. List any dependencies required for
this change.
After you're done, someone will review your PR. They may suggest
improvements. If no one reviews your PR within a few days, feel free to
@-mention the same people again, as notifications can get lost.
Finally, we'd love to show appreciation for your contribution - if you'd
like us to shout you out on Twitter, please also include your handle!
-->
#### Add start index to metadata in TextSplitter
- Modified method `create_documents` to track start position of each
chunk
- The `start_index` is included in the metadata if the `add_start_index`
parameter in the class constructor is set to `True`
This enables referencing back to the original document, particularly
useful when a specific chunk is retrieved.
<!-- If you're adding a new integration, please include:
1. a test for the integration - favor unit tests that does not rely on
network access.
2. an example notebook showing its use
See contribution guidelines for more information on how to write tests,
lint
etc:
https://github.com/hwchase17/langchain/blob/master/.github/CONTRIBUTING.md
-->
#### Who can review?
Tag maintainers/contributors who might be interested:
@eyurtsev @agola11
<!-- For a quicker response, figure out the right person to tag with @
@hwchase17 - project lead
Tracing / Callbacks
- @agola11
Async
- @agola11
DataLoaders
- @eyurtsev
Models
- @hwchase17
- @agola11
Agents / Tools / Toolkits
- @vowelparrot
VectorStores / Retrievers / Memory
- @dev2049
-->
This PR adds a Baseten integration. I've done my best to follow the
contributor's guidelines and add docs, an example notebook, and an
integration test modeled after similar integrations' test.
Please let me know if there is anything I can do to improve the PR. When
it is merged, please tag https://twitter.com/basetenco and
https://twitter.com/philip_kiely as contributors (the note on the PR
template said to include Twitter accounts)
<!--
Thank you for contributing to LangChain! Your PR will appear in our
release under the title you set. Please make sure it highlights your
valuable contribution.
Replace this with a description of the change, the issue it fixes (if
applicable), and relevant context. List any dependencies required for
this change.
After you're done, someone will review your PR. They may suggest
improvements. If no one reviews your PR within a few days, feel free to
@-mention the same people again, as notifications can get lost.
Finally, we'd love to show appreciation for your contribution - if you'd
like us to shout you out on Twitter, please also include your handle!
-->
<!-- Remove if not applicable -->
Fixes#3983
Mimicing what we do for saving and loading VectorDBQA chain, I added the
logic for RetrievalQA chain.
Also added a unit test. I did not find how we test other chains for
their saving and loading functionality, so I just added a file with one
test case. Let me know if there are recommended ways to test it.
#### Before submitting
<!-- If you're adding a new integration, please include:
1. a test for the integration - favor unit tests that does not rely on
network access.
2. an example notebook showing its use
See contribution guidelines for more information on how to write tests,
lint
etc:
https://github.com/hwchase17/langchain/blob/master/.github/CONTRIBUTING.md
-->
#### Who can review?
Tag maintainers/contributors who might be interested:
@dev2049
<!-- For a quicker response, figure out the right person to tag with @
@hwchase17 - project lead
Tracing / Callbacks
- @agola11
Async
- @agola11
DataLoaders
- @eyurtsev
Models
- @hwchase17
- @agola11
Agents / Tools / Toolkits
- @vowelparrot
VectorStores / Retrievers / Memory
- @dev2049
-->
---------
Co-authored-by: Harrison Chase <hw.chase.17@gmail.com>
- Added `SingleStoreDB` vector store, which is a wrapper over the
SingleStore DB database, that can be used as a vector storage and has an
efficient similarity search.
- Added integration tests for the vector store
- Added jupyter notebook with the example
@dev2049
---------
Co-authored-by: Volodymyr Tkachuk <vtkachuk-ua@singlestore.com>
Co-authored-by: Dev 2049 <dev.dev2049@gmail.com>
Co-authored-by: Harrison Chase <hw.chase.17@gmail.com>
Implementation of similarity_search_with_relevance_scores for quadrant
vector store.
As implemented the method is also compatible with other capacities such
as filtering.
Integration tests updated.
#### Who can review?
Tag maintainers/contributors who might be interested:
VectorStores / Retrievers / Memory
- @dev2049
### Summary
Adds an `UnstructuredCSVLoader` for loading CSVs. One advantage of using
`UnstructuredCSVLoader` relative to the standard `CSVLoader` is that if
you use `UnstructuredCSVLoader` in `"elements"` mode, an HTML
representation of the table will be available in the metadata.
#### Who can review?
@hwchase17
@eyurtsev
#### Who can review?
Tag maintainers/contributors who might be interested:
@hwchase17 - project lead
- @agola11
---------
Co-authored-by: Yessen Kanapin <yessen@deepinfra.com>
Fixes # 5807
Realigned tests with implementation.
Also reinforced folder unicity for the test_faiss_local_save_load test
using date-time suffix
#### Before submitting
- Integration test updated
- formatting and linting ok (locally)
#### Who can review?
Tag maintainers/contributors who might be interested:
@hwchase17 - project lead
VectorStores / Retrievers / Memory
-@dev2049
This introduces the `YoutubeAudioLoader`, which will load blobs from a
YouTube url and write them. Blobs are then parsed by
`OpenAIWhisperParser()`, as show in this
[PR](https://github.com/hwchase17/langchain/pull/5580), but we extend
the parser to split audio such that each chuck meets the 25MB OpenAI
size limit. As shown in the notebook, this enables a very simple UX:
```
# Transcribe the video to text
loader = GenericLoader(YoutubeAudioLoader([url],save_dir),OpenAIWhisperParser())
docs = loader.load()
```
Tested on full set of Karpathy lecture videos:
```
# Karpathy lecture videos
urls = ["https://youtu.be/VMj-3S1tku0"
"https://youtu.be/PaCmpygFfXo",
"https://youtu.be/TCH_1BHY58I",
"https://youtu.be/P6sfmUTpUmc",
"https://youtu.be/q8SA3rM6ckI",
"https://youtu.be/t3YJ5hKiMQ0",
"https://youtu.be/kCc8FmEb1nY"]
# Directory to save audio files
save_dir = "~/Downloads/YouTube"
# Transcribe the videos to text
loader = GenericLoader(YoutubeAudioLoader(urls,save_dir),OpenAIWhisperParser())
docs = loader.load()
```
# What does this PR do?
Change the HTML tags so that a tag with attributes can be found.
## Before submitting
- [x] Tests added
- [x] CI/CD validated
### Who can review?
Anyone in the community is free to review the PR once the tests have
passed. Feel free to tag
members/contributors who may be interested in your PR.
- Remove the client implementation (this breaks backwards compatibility
for existing testers. I could keep the stub in that file if we want, but
not many people are using it yet
- Add SDK as dependency
- Update the 'run_on_dataset' method to be a function that optionally
accepts a client as an argument
- Remove the langchain plus server implementation (you get it for free
with the SDK now)
We could make the SDK optional for now, but the plan is to use w/in the
tracer so it would likely become a hard dependency at some point.
Fixes#5614
#### Issue
The `***` combination produces an exception when used as a seperator in
`re.split`. Instead `\*\*\*` should be used for regex exprations.
#### Who can review?
@eyurtsev
Aviary is an open source toolkit for evaluating and deploying open
source LLMs. You can find out more about it on
[http://github.com/ray-project/aviary). You can try it out at
[http://aviary.anyscale.com](aviary.anyscale.com).
This code adds support for Aviary in LangChain. To minimize
dependencies, it connects directly to the HTTP endpoint.
The current implementation is not accelerated and uses the default
implementation of `predict` and `generate`.
It includes a test and a simple example.
@hwchase17 and @agola11 could you have a look at this?
---------
Co-authored-by: Dev 2049 <dev.dev2049@gmail.com>
# OpenAIWhisperParser
This PR creates a new parser, `OpenAIWhisperParser`, that uses the
[OpenAI Whisper
model](https://platform.openai.com/docs/guides/speech-to-text/quickstart)
to perform transcription of audio files to text (`Documents`). Please
see the notebook for usage.
Zep now supports persisting custom metadata with messages and hybrid
search across both message embeddings and structured metadata. This PR
implements custom metadata and enhancements to the
`ZepChatMessageHistory` and `ZepRetriever` classes to implement this
support.
Tag maintainers/contributors who might be interested:
VectorStores / Retrievers / Memory
- @dev2049
---------
Co-authored-by: Daniel Chalef <daniel.chalef@private.org>
# Check if generated Cypher code is wrapped in backticks
Some LLMs like the VertexAI like to explain how they generated the
Cypher statement and wrap the actual code in three backticks:
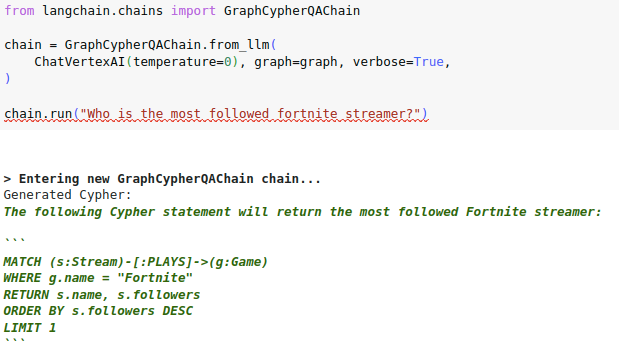
I have observed a similar pattern with OpenAI chat models in a
conversational settings, where multiple user and assistant message are
provided to the LLM to generate Cypher statements, where then the LLM
wants to maybe apologize for previous steps or explain its thoughts.
Interestingly, both OpenAI and VertexAI wrap the code in three backticks
if they are doing any explaining or apologizing. Checking if the
generated cypher is wrapped in backticks seems like a low-hanging fruit
to expand the cypher search to other LLMs and conversational settings.
# Token text splitter for sentence transformers
The current TokenTextSplitter only works with OpenAi models via the
`tiktoken` package. This is not clear from the name `TokenTextSplitter`.
In this (first PR) a token based text splitter for sentence transformer
models is added. In the future I think we should work towards injecting
a tokenizer into the TokenTextSplitter to make ti more flexible.
Could perhaps be reviewed by @dev2049
---------
Co-authored-by: Harrison Chase <hw.chase.17@gmail.com>
Raises exception if OutputParsers receive a response with both a valid
action and a final answer
Currently, if an OutputParser receives a response which includes both an
action and a final answer, they return a FinalAnswer object. This allows
the parser to accept responses which propose an action and hallucinate
an answer without the action being parsed or taken by the agent.
This PR changes the logic to:
1. store a variable checking whether a response contains the
`FINAL_ANSWER_ACTION` (this is the easier condition to check).
2. store a variable checking whether the response contains a valid
action
3. if both are present, raise a new exception stating that both are
present
4. if an action is present, return an AgentAction
5. if an answer is present, return an AgentAnswer
6. if neither is present, raise the relevant exception based around the
action format (these have been kept consistent with the prior exception
messages)
Disclaimer:
* Existing mock data included strings which did include an action and an
answer. This might indicate that prioritising returning AgentAnswer was
always correct, and I am patching out desired behaviour? @hwchase17 to
advice. Curious if there are allowed cases where this is not
hallucinating, and we do want the LLM to output an action which isn't
taken.
* I have not passed `send_to_llm` through this new exception
Fixes#5601
## Who can review?
Community members can review the PR once tests pass. Tag
maintainers/contributors who might be interested:
@hwchase17 - project lead
@vowelparrot
# Unstructured Excel Loader
Adds an `UnstructuredExcelLoader` class for `.xlsx` and `.xls` files.
Works with `unstructured>=0.6.7`. A plain text representation of the
Excel file will be available under the `page_content` attribute in the
doc. If you use the loader in `"elements"` mode, an HTML representation
of the Excel file will be available under the `text_as_html` metadata
key. Each sheet in the Excel document is its own document.
### Testing
```python
from langchain.document_loaders import UnstructuredExcelLoader
loader = UnstructuredExcelLoader(
"example_data/stanley-cups.xlsx",
mode="elements"
)
docs = loader.load()
```
## Who can review?
@hwchase17
@eyurtsev
# Chroma update_document full document embeddings bugfix
Chroma update_document takes a single document, but treats the
page_content sting of that document as a list when getting the new
document embedding.
This is a two-fold problem, where the resulting embedding for the
updated document is incorrect (it's only an embedding of the first
character in the new page_content) and it calls the embedding function
for every character in the new page_content string, using many tokens in
the process.
Fixes#5582
Co-authored-by: Caleb Ellington <calebellington@Calebs-MBP.hsd1.ca.comcast.net>
# Fix Qdrant ids creation
There has been a bug in how the ids were created in the Qdrant vector
store. They were previously calculated based on the texts. However,
there are some scenarios in which two documents may have the same piece
of text but different metadata, and that's a valid case. Deduplication
should be done outside of insertion.
It has been fixed and covered with the integration tests.
---------
Co-authored-by: Dev 2049 <dev.dev2049@gmail.com>