### Summary
Adds a new document loader for processing e-publications. Works with
`unstructured>=0.5.4`. You need to have
[`pandoc`](https://pandoc.org/installing.html) installed for this loader
to work.
### Testing
```python
from langchain.document_loaders import UnstructuredEPubLoader
loader = UnstructuredEPubLoader("winter-sports.epub", mode="elements")
data = loader.load()
data[0]
```
This upsteam wikipedia page loading seems to still have issues. Finding
a compromise solution where it does an exact match search and not a
search for the completion.
See previous PR: https://github.com/hwchase17/langchain/pull/2169
Creating a page using the title causes a wikipedia search with
autocomplete set to true. This frequently causes the summaries to be
unrelated to the actual page found.
See:
1554943e8a/wikipedia/wikipedia.py (L254-L280)
`predict_and_parse` exists, and it's a nice abstraction to allow for
applying output parsers to LLM generations. And async is very useful.
As an aside, the difference between `call/acall`, `predict/apredict` and
`generate/agenerate` isn't entirely
clear to me other than they all call into the LLM in slightly different
ways.
Is there some documentation or a good way to think about these
differences?
One thought:
output parsers should just work magically for all those LLM calls. If
the `output_parser` arg is set on the prompt, the LLM has access, so it
seems like extra work on the user's end to have to call
`output_parser.parse`
If this sounds reasonable, happy to throw something together. @hwchase17
- Current docs are pointing to the wrong module, fixed
- Added some explanation on how to find the necessary parameters
- Added chat-based codegen example w/ retrievers
Picture of the new page:
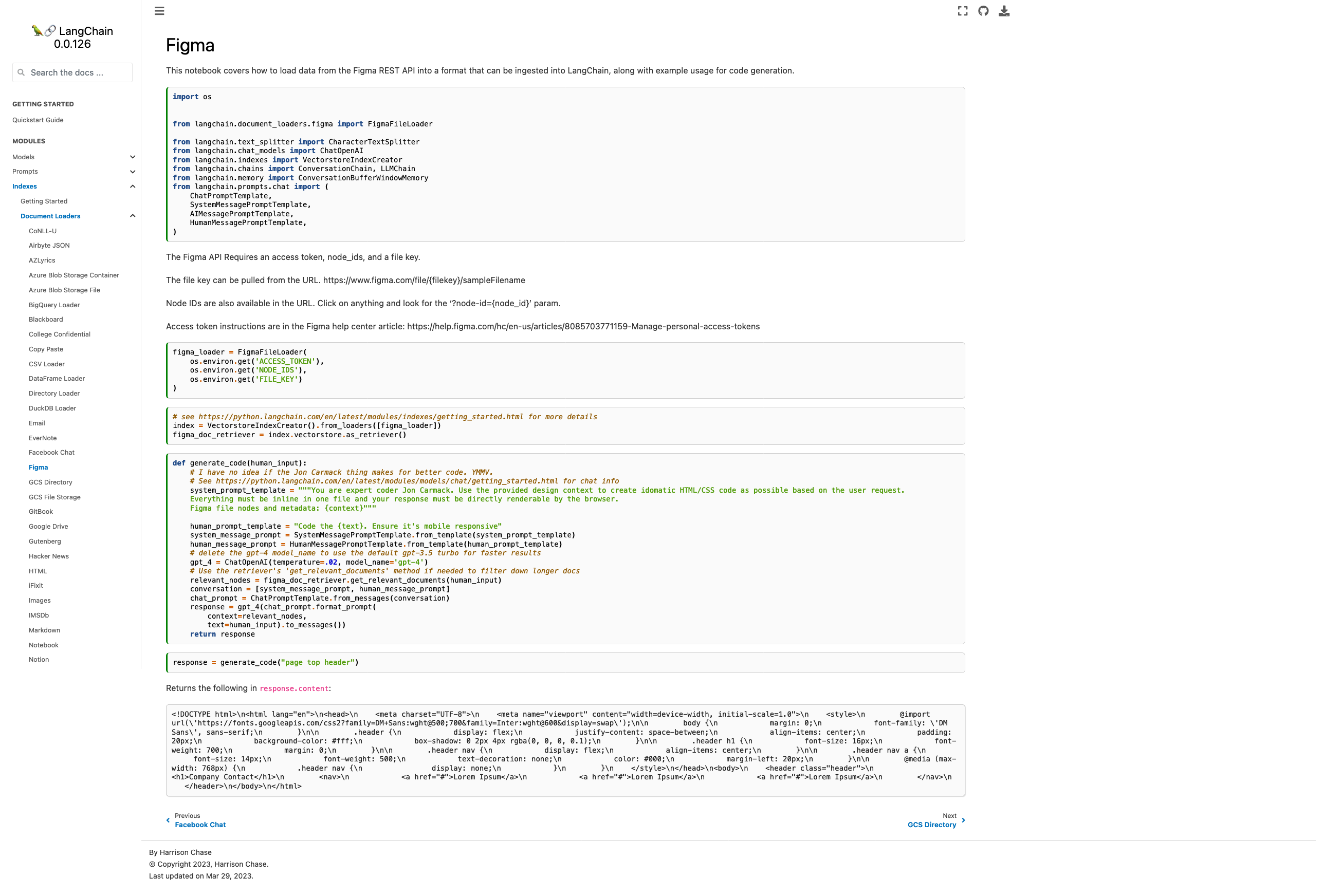
Please let me know if you'd like any tweaks! I wasn't sure if the
example was too heavy for the page or not but decided "hey, I probably
would want to see it" and so included it.
Co-authored-by: maxtheman <max@maxs-mbp.lan>
The new functionality of Redis backend for chat message history
([see](https://github.com/hwchase17/langchain/pull/2122)) uses the Redis
list object to store messages and then uses the `lrange()` to retrieve
the list of messages
([see](https://github.com/hwchase17/langchain/blob/master/langchain/memory/chat_message_histories/redis.py#L50)).
Unfortunately this retrieves the messages as a list sorted in the
opposite order of how they were inserted - meaning the last inserted
message will be first in the retrieved list - which is not what we want.
This PR fixes that as it changes the order to match the order of
insertion.
Currently, if a tool is set to verbose, an agent can override it by
passing in its own verbose flag. This is not ideal if we want to stream
back responses from agents, as we want the llm and tools to be sending
back events but nothing else. This also makes the behavior consistent
with ts.
This merge includes updated comments in the ElasticVectorSearch class to
provide information on how to connect to `Elasticsearch` instances that
require login credentials, including Elastic Cloud, without any
functional changes.
The `ElasticVectorSearch` class now inherits from the `ABC` abstract
base class, which does not break or change any functionality. This
allows for easy subclassing and creation of custom implementations in
the future or for any users, especially for me 😄
I confirm that before pushing these changes, I ran:
```bash
make format && make lint
```
To ensure that the new documentation is rendered correctly I ran
```bash
make docs_build
```
To ensure that the new documentation has no broken links, I ran a check
```bash
make docs_linkcheck
```
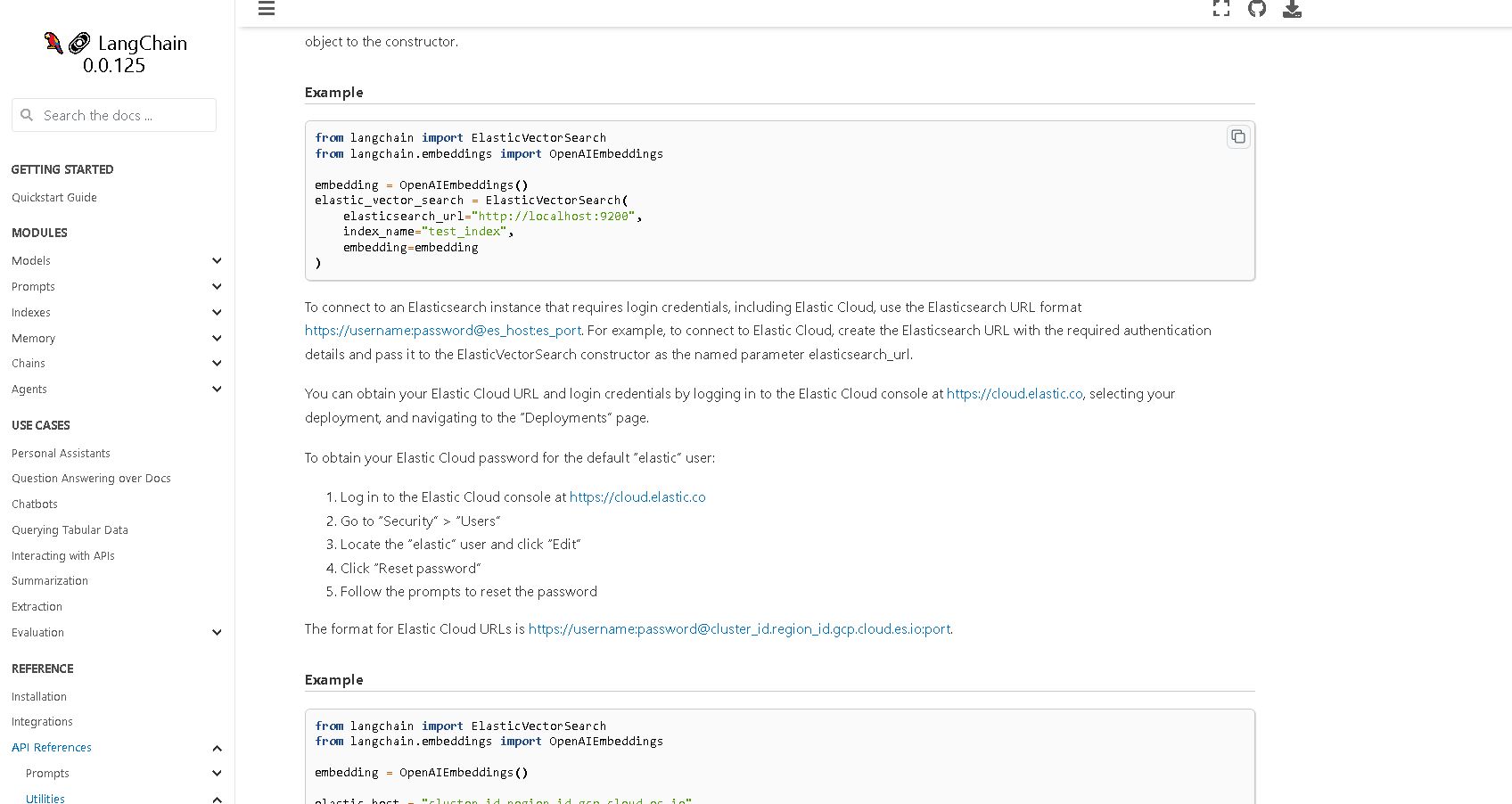
Also take a look at https://github.com/hwchase17/langchain/issues/1865
P.S. Sorry for spamming you with force-pushes. In the future, I will be
smarter.
@3coins + @zoltan-fedor.... heres the pr + some minor changes i made.
thoguhts? can try to get it into tmrws release
---------
Co-authored-by: Zoltan Fedor <zoltan.0.fedor@gmail.com>
Co-authored-by: Piyush Jain <piyushjain@duck.com>
Currently only google documents and pdfs can be loaded from google
drive. This PR implements the latest recommended method for getting
google sheets including all tabs.
It currently parses the google sheet data the exact same way as the csv
loader - the only difference is that the gdrive sheets loader is not
using the `csv` library since the data is already in a list.
I've found it useful to track the number of successful requests to
OpenAI. This gives me a better sense of the efficiency of my prompts and
helps compare map_reduce/refine on a cheaper model vs. stuffing on a
more expensive model with higher capacity.
Loading this sitemap didn't work for me
https://www.alzallies.com/sitemap.xml
Changing this fixed it and it seems like a good idea to do it in
general.
Integration tests pass
Fix the issue outlined in #1712 to ensure the `BaseQAWithSourcesChain`
can properly separate the sources from an agent response even when they
are delineated by a newline.
This will ensure the `BaseQAWithSourcesChain` can reliably handle both
of these agent outputs:
* `"This Agreement is governed by English law.\nSOURCES: 28-pl"` ->
`"This Agreement is governed by English law.\n`, `"28-pl"`
* `"This Agreement is governed by English law.\nSOURCES:\n28-pl"` ->
`"This Agreement is governed by English law.\n`, `"28-pl"`
I couldn't find any unit tests for this but please let me know if you'd
like me to add any test coverage.
1. Removed the `summaries` dictionary in favor of directly appending to
the summary_strings list, which avoids the unnecessary double-loop.
2. Simplified the logic for populating the `context` variable.
Co-created with GPT-4 @agihouse
This worked for me, but I'm not sure if its the right way to approach
something like this, so I'm open to suggestions.
Adds class properties `reduce_k_below_max_tokens: bool` and
`max_tokens_limit: int` to the `ConversationalRetrievalChain`. The code
is basically copied from
[`RetreivalQAWithSourcesChain`](46d141c6cb/langchain/chains/qa_with_sources/retrieval.py (L24))
Seems like a copy paste error. The very next example does have this
line.
Please tell me if I missed something in the process and should have
created an issue or something first!