mirror of https://github.com/arc53/DocsGPT
Nextra docs
parent
94738d8fc4
commit
4f735a5d11
@ -0,0 +1 @@
|
||||
# nextra-docsgpt
|
@ -0,0 +1,9 @@
|
||||
const withNextra = require('nextra')({
|
||||
theme: 'nextra-theme-docs',
|
||||
themeConfig: './theme.config.jsx'
|
||||
})
|
||||
|
||||
module.exports = withNextra()
|
||||
|
||||
// If you have other Next.js configurations, you can pass them as the parameter:
|
||||
// module.exports = withNextra({ /* other next.js config */ })
|
File diff suppressed because it is too large
Load Diff
@ -0,0 +1,10 @@
|
||||
{
|
||||
"dependencies": {
|
||||
"@vercel/analytics": "^1.0.2",
|
||||
"next": "^13.4.19",
|
||||
"nextra": "^2.12.3",
|
||||
"nextra-theme-docs": "^2.12.3",
|
||||
"react": "^18.2.0",
|
||||
"react-dom": "^18.2.0"
|
||||
}
|
||||
}
|
@ -0,0 +1,90 @@
|
||||
# Self-hosting DocsGPT on Amazon Lightsail
|
||||
|
||||
Here's a step-by-step guide on how to setup an Amazon Lightsail instance to host DocsGPT.
|
||||
|
||||
## Configuring your instance
|
||||
|
||||
(If you know how to create a Lightsail instance, you can skip to the recommended configuration part by clicking here)
|
||||
|
||||
### 1. Create an account or login to https://lightsail.aws.amazon.com
|
||||
|
||||
### 2. Click on "Create instance"
|
||||
|
||||
### 3. Create your instance
|
||||
|
||||
The first step is to select the "Instance location". In most cases there's no need to switch locations as the default one will work well.
|
||||
|
||||
After that it is time to pick your Instance Image. We recommend using "Linux/Unix" as the image and "Ubuntu 20.04 LTS" for Operating System.
|
||||
|
||||
As for instance plan, it'll vary depending on your unique demands, but a "1 GB, 1vCPU, 40GB SSD and 2TB transfer" setup should cover most scenarios.
|
||||
|
||||
Lastly, Identify your instance by giving it a unique name and then hit "Create instance".
|
||||
|
||||
PS: Once you create your instance, it'll likely take a few minutes for the setup to be completed.
|
||||
|
||||
#### The recommended configuration is as follows:
|
||||
|
||||
- Ubuntu 20.04 LTS
|
||||
- 1GB RAM
|
||||
- 1vCPU
|
||||
- 40GB SSD Hard Drive
|
||||
- 2TB transfer
|
||||
|
||||
### Connecting to your the newly created instance
|
||||
|
||||
Your instance will be ready for use a few minutes after being created. To access, just open it up and click on "Connect using SSH".
|
||||
|
||||
#### Clone the repository
|
||||
|
||||
A terminal window will pop up, and the first step will be to clone DocsGPT git repository.
|
||||
|
||||
`git clone https://github.com/arc53/DocsGPT.git`
|
||||
|
||||
#### Download the package information
|
||||
|
||||
Once it has finished cloning the repository, it is time to download the package information from all sources. To do so simply enter the following command:
|
||||
|
||||
`sudo apt update`
|
||||
|
||||
#### Install python3
|
||||
|
||||
DocsGPT backend uses python, which means it needs to be installed in order to use it.
|
||||
|
||||
`sudo apt install python3-pip`
|
||||
|
||||
#### Access the DocsGPT folder
|
||||
|
||||
Enter the following command to access the folder in which DocsGPT application was installed.
|
||||
|
||||
`cd DocsGPT/application`
|
||||
|
||||
#### Install the required dependencies
|
||||
|
||||
Inside the applications folder there's a .txt file with a list of all dependencies required to run DocsGPT.
|
||||
|
||||
`pip3 install -r requirements.txt`
|
||||
|
||||
#### Running the app
|
||||
|
||||
You're almost there! Now that all the necessary bits and pieces have been installed, it is time to run the application. To do so, use the following command:
|
||||
|
||||
`tmux new`
|
||||
|
||||
And then:
|
||||
|
||||
`python3 -m flask run --host 0.0.0.0 --port 5000`
|
||||
|
||||
Once this is done you can go ahead and close the terminal window.
|
||||
|
||||
#### Enabling port 5000
|
||||
|
||||
Before you being able to access your live instance, you must first enable the port which it is using.
|
||||
|
||||
Open your Lightsail instance and head to "Networking".
|
||||
|
||||
Then click on "Add rule" under "IPv4 Firewall", enter 5000 as your your port and hit "Create".
|
||||
|
||||
#### Access your instance
|
||||
|
||||
Your instance will now be available under your Public IP Address and port 5000. Enjoy!
|
||||
|
@ -0,0 +1,23 @@
|
||||
## Launching Web App
|
||||
Note: Make sure you have docker installed
|
||||
|
||||
1. Open dowload this repository with `git clone https://github.com/arc53/DocsGPT.git`
|
||||
2. Create .env file in your root directory and set your `OPENAI_API_KEY` with your openai api key
|
||||
3. Run `docker-compose build && docker-compose up`
|
||||
4. Navigate to `http://localhost:5173/`
|
||||
|
||||
To stop just run Ctrl + C
|
||||
|
||||
### Chrome Extension
|
||||
|
||||
To install the Chrome extension:
|
||||
|
||||
1. In the DocsGPT GitHub repository, click on the "Code" button and select Download ZIP
|
||||
2. Unzip the downloaded file to a location you can easily access
|
||||
3. Open the Google Chrome browser and click on the three dots menu (upper right corner)
|
||||
4. Select "More Tools" and then "Extensions"
|
||||
5. Turn on the "Developer mode" switch in the top right corner of the Extensions page
|
||||
6. Click on the "Load unpacked" button
|
||||
7. Select the "Chrome" folder where the DocsGPT files have been unzipped (docsgpt-main > extensions > chrome)
|
||||
8. The extension should now be added to Google Chrome and can be managed on the Extensions page
|
||||
9. To disable or remove the extension, simply turn off the toggle switch on the extension card or click the "Remove" button.
|
@ -0,0 +1,153 @@
|
||||
App currently has two main api endpoints:
|
||||
|
||||
### /api/answer
|
||||
Its a POST request that sends a JSON in body with 4 values. Here is a JavaScript fetch example
|
||||
It will recieve an answer for a user provided question
|
||||
|
||||
```
|
||||
// answer (POST http://127.0.0.1:5000/api/answer)
|
||||
fetch("http://127.0.0.1:5000/api/answer", {
|
||||
"method": "POST",
|
||||
"headers": {
|
||||
"Content-Type": "application/json; charset=utf-8"
|
||||
},
|
||||
"body": JSON.stringify({"question":"Hi","history":null,"api_key":"OPENAI_API_KEY","embeddings_key":"OPENAI_API_KEY",
|
||||
"active_docs": "javascript/.project/ES2015/openai_text-embedding-ada-002/"})
|
||||
})
|
||||
.then((res) => res.text())
|
||||
.then(console.log.bind(console))
|
||||
```
|
||||
|
||||
In response you will get a json document like this one:
|
||||
|
||||
```
|
||||
{
|
||||
"answer": " Hi there! How can I help you?\n",
|
||||
"query": "Hi",
|
||||
"result": " Hi there! How can I help you?\nSOURCES:"
|
||||
}
|
||||
```
|
||||
|
||||
### /api/docs_check
|
||||
It will make sure documentation is loaded on a server (just run it everytime user is switching between libraries (documentations)
|
||||
Its a POST request that sends a JSON in body with 1 value. Here is a JavaScript fetch example
|
||||
|
||||
```
|
||||
// answer (POST http://127.0.0.1:5000/api/docs_check)
|
||||
fetch("http://127.0.0.1:5000/api/docs_check", {
|
||||
"method": "POST",
|
||||
"headers": {
|
||||
"Content-Type": "application/json; charset=utf-8"
|
||||
},
|
||||
"body": JSON.stringify({"docs":"javascript/.project/ES2015/openai_text-embedding-ada-002/"})
|
||||
})
|
||||
.then((res) => res.text())
|
||||
.then(console.log.bind(console))
|
||||
```
|
||||
|
||||
In response you will get a json document like this one:
|
||||
```
|
||||
{
|
||||
"status": "exists"
|
||||
}
|
||||
```
|
||||
|
||||
|
||||
### /api/combine
|
||||
Provides json that tells UI which vectors are available and where they are located with a simple get request
|
||||
|
||||
Respsonse will include:
|
||||
date, description, docLink, fullName, language, location (local or docshub), model, name, version
|
||||
|
||||
Example of json in Docshub and local:
|
||||
<img width="295" alt="image" src="https://user-images.githubusercontent.com/15183589/224714085-f09f51a4-7a9a-4efb-bd39-798029bb4273.png">
|
||||
|
||||
|
||||
### /api/upload
|
||||
Uploads file that needs to be trained, response is json with task id, which can be used to check on tasks progress
|
||||
HTML example:
|
||||
|
||||
```
|
||||
<form action="/api/upload" method="post" enctype="multipart/form-data" class="mt-2">
|
||||
<input type="file" name="file" class="py-4" id="file-upload">
|
||||
<input type="text" name="user" value="local" hidden>
|
||||
<input type="text" name="name" placeholder="Name:">
|
||||
|
||||
|
||||
<button type="submit" class="py-2 px-4 text-white bg-blue-500 rounded-md hover:bg-blue-600 focus:outline-none focus:ring-2 focus:ring-offset-2 focus:ring-blue-500">
|
||||
Upload
|
||||
</button>
|
||||
</form>
|
||||
```
|
||||
|
||||
Response:
|
||||
```
|
||||
{
|
||||
"status": "ok",
|
||||
"task_id": "b2684988-9047-428b-bd47-08518679103c"
|
||||
}
|
||||
|
||||
```
|
||||
|
||||
### /api/task_status
|
||||
Gets task status (task_id) from /api/upload
|
||||
```
|
||||
// Task status (Get http://127.0.0.1:5000/api/task_status)
|
||||
fetch("http://localhost:5001/api/task_status?task_id=b2d2a0f4-387c-44fd-a443-e4fe2e7454d1", {
|
||||
"method": "GET",
|
||||
"headers": {
|
||||
"Content-Type": "application/json; charset=utf-8"
|
||||
},
|
||||
})
|
||||
.then((res) => res.text())
|
||||
.then(console.log.bind(console))
|
||||
```
|
||||
|
||||
Responses:
|
||||
There are two types of repsonses:
|
||||
1. while task it still running, where "current" will show progress from 0 - 100
|
||||
```
|
||||
{
|
||||
"result": {
|
||||
"current": 1
|
||||
},
|
||||
"status": "PROGRESS"
|
||||
}
|
||||
```
|
||||
|
||||
2. When task is completed
|
||||
```
|
||||
{
|
||||
"result": {
|
||||
"directory": "temp",
|
||||
"filename": "install.rst",
|
||||
"formats": [
|
||||
".rst",
|
||||
".md",
|
||||
".pdf"
|
||||
],
|
||||
"name_job": "somename",
|
||||
"user": "local"
|
||||
},
|
||||
"status": "SUCCESS"
|
||||
}
|
||||
```
|
||||
|
||||
### /api/delete_old
|
||||
deletes old vecotstores
|
||||
```
|
||||
// Task status (GET http://127.0.0.1:5000/api/docs_check)
|
||||
fetch("http://localhost:5001/api/task_status?task_id=b2d2a0f4-387c-44fd-a443-e4fe2e7454d1", {
|
||||
"method": "GET",
|
||||
"headers": {
|
||||
"Content-Type": "application/json; charset=utf-8"
|
||||
},
|
||||
})
|
||||
.then((res) => res.text())
|
||||
.then(console.log.bind(console))
|
||||
```
|
||||
response:
|
||||
|
||||
```
|
||||
{"status": 'ok'}
|
||||
```
|
@ -0,0 +1,6 @@
|
||||
{
|
||||
"API-docs": {
|
||||
"title": "🗂️️ API-docs",
|
||||
"href": "/Developing/API-docs"
|
||||
}
|
||||
}
|
@ -0,0 +1,29 @@
|
||||
### To start chatwoot extension:
|
||||
1. Prepare and start the DocsGPT itself (load your documentation too)
|
||||
Follow our [wiki](https://github.com/arc53/DocsGPT/wiki) to start it and to [ingest](https://github.com/arc53/DocsGPT/wiki/How-to-train-on-other-documentation) data
|
||||
2. Go to chatwoot, Navigate to your profile (bottom left), click on profile settings, scroll to the bottom and copy Access Token
|
||||
2. Navigate to `/extensions/chatwoot`. Copy .env_sample and create .env file
|
||||
3. Fill in the values
|
||||
|
||||
```
|
||||
docsgpt_url=<docsgpt_api_url>
|
||||
chatwoot_url=<chatwoot_url>
|
||||
docsgpt_key=<openai_api_key or other llm key>
|
||||
chatwoot_token=<from part 2>
|
||||
```
|
||||
|
||||
4. start with `flask run` command
|
||||
|
||||
If you want for bot to stop responding to questions for a specific user or session just add label `human-requested` in your conversation
|
||||
|
||||
|
||||
### Optional (extra validation)
|
||||
In app.py uncomment lines 12-13 and 71-75
|
||||
|
||||
in your .env file add:
|
||||
|
||||
`account_id=(optional) 1 `
|
||||
|
||||
`assignee_id=(optional) 1`
|
||||
|
||||
Those are chatwoot values and will allow you to check if you are responding to correct widget and responding to questions assigned to specific user
|
@ -0,0 +1,6 @@
|
||||
{
|
||||
"Chatwoot-extension": {
|
||||
"title": "💬️ Chatwoot Extension",
|
||||
"href": "/Extensions/Chatwoot-extension"
|
||||
}
|
||||
}
|
@ -0,0 +1,4 @@
|
||||
## To customise a main prompt navigate to `/application/prompt/combine_prompt.txt`
|
||||
|
||||
You can try editing it to see how the model responds.
|
||||
|
@ -0,0 +1,60 @@
|
||||
## How to train on other documentation
|
||||
This AI can use any documentation, but first it needs to be prepared for similarity search.
|
||||
|
||||
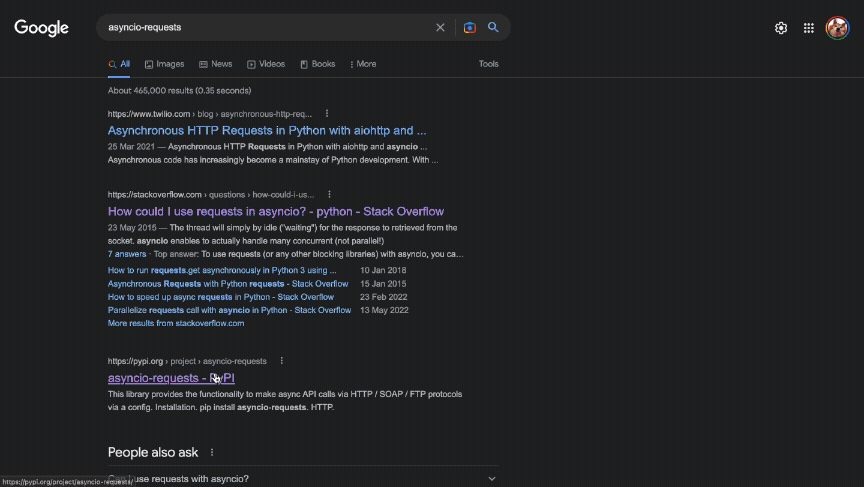
|
||||
|
||||
Start by going to
|
||||
`/scripts/` folder
|
||||
|
||||
If you open this file you will see that it uses RST files from the folder to create a `index.faiss` and `index.pkl`.
|
||||
|
||||
It currently uses OPEN_AI to create vector store, so make sure your documentation is not too big. Pandas cost me around 3-4$
|
||||
|
||||
You can usually find documentation on github in docs/ folder for most open-source projects.
|
||||
|
||||
### 1. Find documentation in .rst/.md and create a folder with it in your scripts directory
|
||||
Name it `inputs/`
|
||||
Put all your .rst/.md files in there
|
||||
The search is recursive, so you don't need to flatten them
|
||||
|
||||
If there are no .rst/.md files just convert whatever you find to txt and feed it. (don't forget to change the extension in script)
|
||||
|
||||
### 2. Create .env file in `scripts/` folder
|
||||
And write your OpenAI API key inside
|
||||
`OPENAI_API_KEY=<your-api-key>`
|
||||
|
||||
### 3. Run scripts/ingest.py
|
||||
|
||||
`python ingest.py ingest`
|
||||
|
||||
It will tell you how much it will cost
|
||||
|
||||
### 4. Move `index.faiss` and `index.pkl` generated in `scripts/output` to `application/` folder.
|
||||
|
||||
|
||||
### 5. Run web app
|
||||
Once you run it will use new context that is relevant to your documentation
|
||||
Make sure you select default in the dropdown in the UI
|
||||
|
||||
## Customisation
|
||||
You can learn more about options while running ingest.py by running:
|
||||
|
||||
`python ingest.py --help`
|
||||
| Options | |
|
||||
|:--------------------------------:|:------------------------------------------------------------------------------------------------------------------------------:|
|
||||
| **ingest** | Runs 'ingest' function converting documentation to to Faiss plus Index format |
|
||||
| --dir TEXT | List of paths to directory for index creation. E.g. --dir inputs --dir inputs2 [default: inputs] |
|
||||
| --file TEXT | File paths to use (Optional; overrides directory) E.g. --files inputs/1.md --files inputs/2.md |
|
||||
| --recursive / --no-recursive | Whether to recursively search in subdirectories [default: recursive] |
|
||||
| --limit INTEGER | Maximum number of files to read |
|
||||
| --formats TEXT | List of required extensions (list with .) Currently supported: .rst, .md, .pdf, .docx, .csv, .epub, .html [default: .rst, .md] |
|
||||
| --exclude / --no-exclude | Whether to exclude hidden files (dotfiles) [default: exclude] |
|
||||
| -y, --yes | Whether to skip price confirmation |
|
||||
| --sample / --no-sample | Whether to output sample of the first 5 split documents. [default: no-sample] |
|
||||
| --token-check / --no-token-check | Whether to group small documents and split large. Improves semantics. [default: token-check] |
|
||||
| --min_tokens INTEGER | Minimum number of tokens to not group. [default: 150] |
|
||||
| --max_tokens INTEGER | Maximum number of tokens to not split. [default: 2000] |
|
||||
| | |
|
||||
| **convert** | Creates documentation in .md format from source code |
|
||||
| --dir TEXT | Path to a directory with source code. E.g. --dir inputs [default: inputs] |
|
||||
| --formats TEXT | Source code language from which to create documentation. Supports py, js and java. E.g. --formats py [default: py] |
|
@ -0,0 +1,32 @@
|
||||
Fortunately there are many providers for LLM's and some of them can even be ran locally
|
||||
|
||||
There are two models used in the app:
|
||||
1. Embeddings
|
||||
2. Text generation
|
||||
|
||||
By default we use OpenAI's models but if you want to change it or even run it locally, its very simple!
|
||||
|
||||
### Go to .env file or set environment variables:
|
||||
|
||||
`LLM_NAME=<your Text generation>`
|
||||
|
||||
`API_KEY=<api_key for Text generation>`
|
||||
|
||||
`EMBEDDINGS_NAME=<llm for embeddings>`
|
||||
|
||||
`EMBEDDINGS_KEY=<api_key for embeddings>`
|
||||
|
||||
`VITE_API_STREAMING=<true or false (true if using openai, false for all others)>`
|
||||
|
||||
You dont need to provide keys if you are happy with users providing theirs, so make sure you set LLM_NAME and EMBEDDINGS_NAME
|
||||
|
||||
Options:
|
||||
LLM_NAME (openai, manifest, cohere, Arc53/docsgpt-14b, Arc53/docsgpt-7b-falcon)
|
||||
EMBEDDINGS_NAME (openai_text-embedding-ada-002, huggingface_sentence-transformers/all-mpnet-base-v2, huggingface_hkunlp/instructor-large, cohere_medium)
|
||||
|
||||
Thats it!
|
||||
|
||||
### Hosting everything locally and privately (for using our optimised open-source models)
|
||||
If you are working with important data and dont want anything to leave your premises.
|
||||
|
||||
Make sure you set SELF_HOSTED_MODEL as true in you .env variable and for your LLM_NAME you can use anything thats on Huggingface
|
@ -0,0 +1,19 @@
|
||||
If your AI uses external knowledge and is not explicit enough it ok, because we try to make docsgpt friendly.
|
||||
|
||||
But if you want to adjust it here is a simple way.
|
||||
|
||||
Got to `application/prompts/chat_combine_prompt.txt`
|
||||
|
||||
And change it to
|
||||
|
||||
|
||||
```
|
||||
|
||||
You are a DocsGPT, friendly and helpful AI assistant by Arc53 that provides help with documents. You give thorough answers with code examples if possible.
|
||||
Write an answer for the question below based on the provided context.
|
||||
If the context provides insufficient information, reply "I cannot answer".
|
||||
You have access to chat history, and can use it to help answer the question.
|
||||
----------------
|
||||
{summaries}
|
||||
|
||||
```
|
@ -0,0 +1,18 @@
|
||||
{
|
||||
"Customising-prompts": {
|
||||
"title": "🏗️️ Customising Prompts",
|
||||
"href": "/Guides/Customising-prompts"
|
||||
},
|
||||
"How-to-train-on-other-documentation": {
|
||||
"title": "📥 Training on docs",
|
||||
"href": "/Guides/How-to-train-on-other-documentation"
|
||||
},
|
||||
"How-to-use-different-LLM": {
|
||||
"title": "⚙️️ How to use different LLM's",
|
||||
"href": "/Guides/How-to-use-different-LLM"
|
||||
},
|
||||
"My-AI-answers-questions-using-external-knowledge": {
|
||||
"title": "💭️ Avoiding hallucinations",
|
||||
"href": "/Guides/My-AI-answers-questions-using-external-knowledge"
|
||||
}
|
||||
}
|
@ -0,0 +1,32 @@
|
||||
---
|
||||
title: 'Home'
|
||||
---
|
||||
import { Cards, Card } from 'nextra/components'
|
||||
import deployingGuides from './Deploying/_meta.json';
|
||||
import developingGuides from './Developing/_meta.json';
|
||||
import extensionGuides from './Extensions/_meta.json';
|
||||
import mainGuides from './Guides/_meta.json';
|
||||
|
||||
export const allGuides = {
|
||||
...mainGuides,
|
||||
...developingGuides,
|
||||
...deployingGuides,
|
||||
...extensionGuides,
|
||||
};
|
||||
|
||||
### **DocsGPT 🦖**
|
||||
|
||||
DocsGPT 🦖 is an innovative open-source tool designed to simplify the retrieval of information from project documentation using advanced GPT models 🤖. Eliminate lengthy manual searches 🔍 and enhance your documentation experience with DocsGPT, and consider contributing to its AI-powered future 🚀.
|
||||
|
||||
|
||||
|
||||
<Cards
|
||||
num={3}
|
||||
children={Object.keys(allGuides).map((key, i) => (
|
||||
<Card
|
||||
key={i}
|
||||
title={allGuides[key].title}
|
||||
href={allGuides[key].href}
|
||||
/>
|
||||
))}
|
||||
/>
|
Binary file not shown.
After Width: | Height: | Size: 191 KiB |
Binary file not shown.
After Width: | Height: | Size: 17 KiB |
Binary file not shown.
After Width: | Height: | Size: 1.1 KiB |
Binary file not shown.
After Width: | Height: | Size: 1.9 KiB |
@ -0,0 +1,19 @@
|
||||
{
|
||||
"name": "",
|
||||
"short_name": "",
|
||||
"icons": [
|
||||
{
|
||||
"src": "/android-chrome-192x192.png",
|
||||
"sizes": "192x192",
|
||||
"type": "image/png"
|
||||
},
|
||||
{
|
||||
"src": "/android-chrome-512x512.png",
|
||||
"sizes": "512x512",
|
||||
"type": "image/png"
|
||||
}
|
||||
],
|
||||
"theme_color": "#ffffff",
|
||||
"background_color": "#ffffff",
|
||||
"display": "standalone"
|
||||
}
|
Loading…
Reference in New Issue